NLDDPG Based Joint Optimization Decision Scheme for Vehicular Network Offloading and Resource Allocation
TENCON 2023 - 2023 IEEE Region 10 Conference (TENCON)(2023)
摘要
In response to the explosive growth of data computation in vehicular terminals, computation offloading has emerged as a viable solution to mitigate the limitations of resources. Efficient offloading decisions not only meet the demanding requirements of complex vehicular tasks in terms of time, energy consumption, and computational performance but also minimize competition and resource consumption in the network. However, existing work on task offloading in vehicular networks often exhibits certain limitations, such as incomplete consideration of relevant factors or suboptimal utilization of available resources. This research presents the construction of a three-layer vehicular network environment, which is based on cloud and edge computing paradigms. The design entails the formulation of real-time vehicle location tracking and task priority metrics, while also considering the challenges posed by time-varying channels and signal blockage prevalent in vehicular network environments. In this paper, a novel variant of the Deep Deterministic Policy Gradient (DDPG) algorithm NLDDPG is proposed to iteratively train the model, aiming to optimize a weighted objective function. Simulation results show that this algorithm can improve the efficiency and optimize the task average utility.
更多查看译文
关键词
Offloading decision making,Deep reinforce-ment learning,Deep reinforcement learning,Cloud computing,Edge computing
AI 理解论文
溯源树
样例
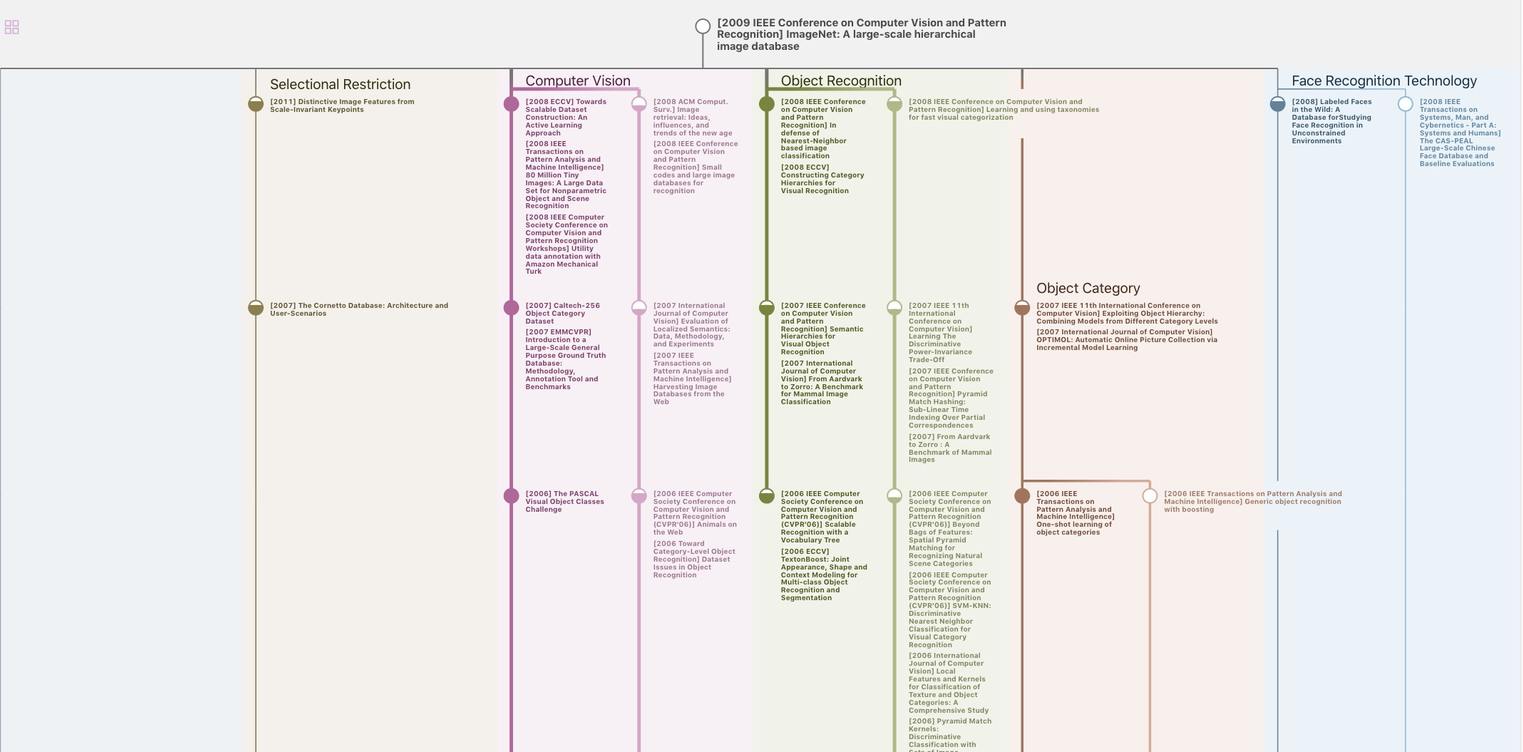
生成溯源树,研究论文发展脉络
Chat Paper
正在生成论文摘要