End-to-end machine-learning for high-gravity ammonia stripping: Bridging the gap between scientific research and user-friendly applications
WATER RESEARCH(2024)
摘要
The removal and recovery of ammonia from wastewater are critical processes for achieving global environmental sustainability and promoting circular economic development. High-gravity technology is an advanced solution to achieve ammonia stripping from wastewater. This study used machine-learning (ML) techniques to provide more comprehensive insights on various influencing factors, including the operating parameters, wastewater characteristics, and design parameters of rotating packed beds. Bayesian auto-optimization combined with a boosting algorithm effectively overcame the challenges of modeling complex datasets with small sample sizes, multidimensional data, missing values, and skewed distributions. Accurate ML based predictive models for the ammonia removal efficiency (eta) and mass transfer coefficient (KLa) were developed, the performance on the training set was R2 = 0.98 and R2 = 0.89, and on the testing set was R2 = 0.98 and R2 = 0.82. The developed model revealed that the stripping stage and gas-liquid ratio were the most influential features for predicting eta, whereas the liquid flow and high-gravity factor were the most important features for predicting KLa. The well-trained model was then deployed in an online software application that could provide both predictive and auto-update functions for operators and managers, ensuring that practitioners could use the model. The end-to-end machine-learning approach used in this study-that is, covering data collection, model development, and application-could improve the availability of research results, providing valuable references for the further advancement of technology in the field of environmental.
更多查看译文
关键词
Wastewater,Ammonia removal,High-gravity technology,Machine learning,Model deployment
AI 理解论文
溯源树
样例
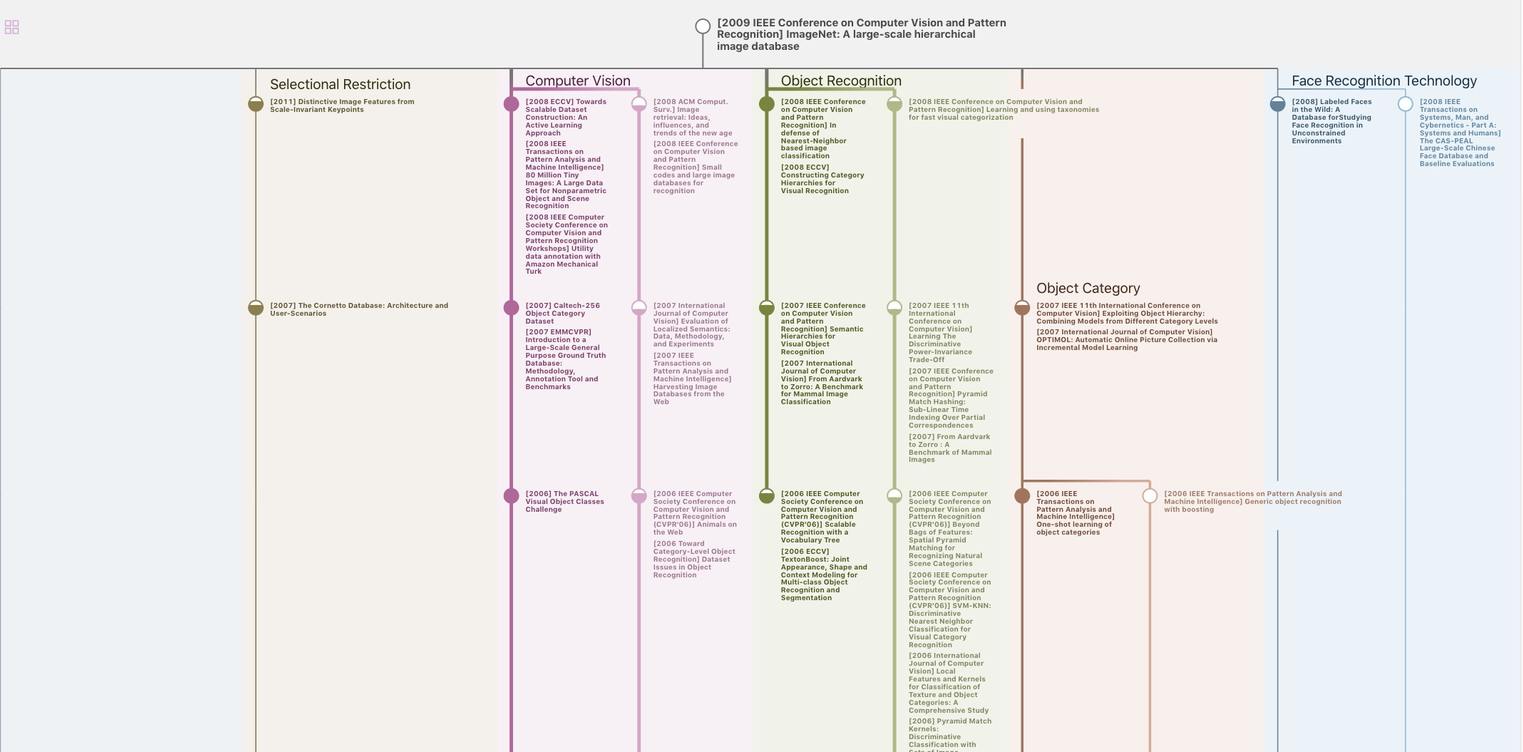
生成溯源树,研究论文发展脉络
Chat Paper
正在生成论文摘要