Linear Log-Normal Attention with Unbiased Concentration
ICLR 2024(2023)
摘要
Transformer models have achieved remarkable results in a wide range of
applications. However, their scalability is hampered by the quadratic time and
memory complexity of the self-attention mechanism concerning the sequence
length. This limitation poses a substantial obstacle when dealing with long
documents or high-resolution images. In this work, we study the self-attention
mechanism by analyzing the distribution of the attention matrix and its
concentration ability. Furthermore, we propose instruments to measure these
quantities and introduce a novel self-attention mechanism, Linear Log-Normal
Attention, designed to emulate the distribution and concentration behavior of
the original self-attention. Our experimental results on popular natural
language benchmarks reveal that our proposed Linear Log-Normal Attention
outperforms other linearized attention alternatives, offering a promising
avenue for enhancing the scalability of transformer models. Our code is
available in supplementary materials.
更多查看译文
关键词
Neural Networks,Transformers,Self-Attention,Linear Attention,Scalable Transformers,Efficient Attention,Attention with Linear Complexity,Linearized Attention,Self-Attention Analysis
AI 理解论文
溯源树
样例
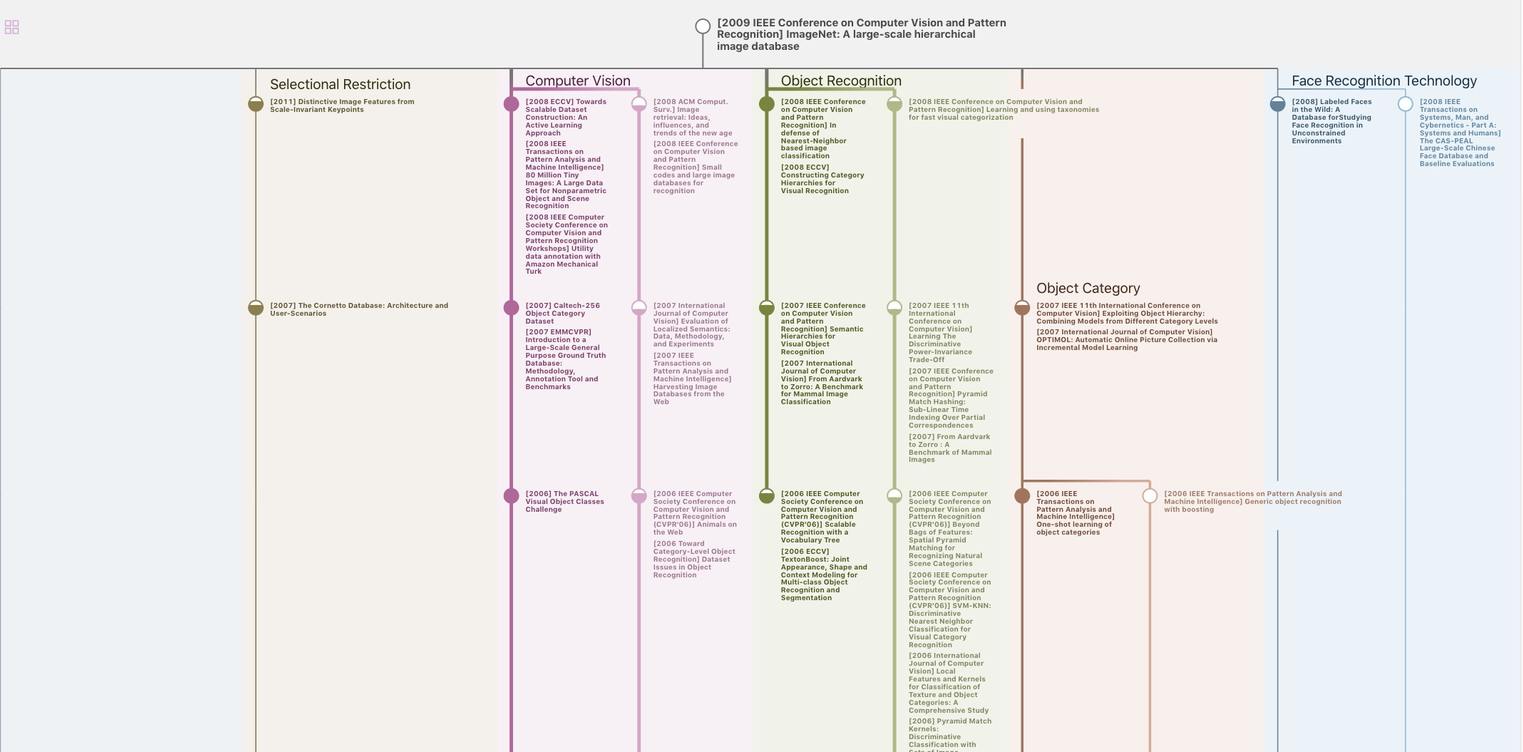
生成溯源树,研究论文发展脉络
Chat Paper
正在生成论文摘要