Advancing the rate-distortion-computation frontier for neural image compression
2023 IEEE INTERNATIONAL CONFERENCE ON IMAGE PROCESSING, ICIP(2023)
摘要
The rate-distortion performance of neural image compression models has exceeded the state-of-the-art for non-learned codecs, but neural codecs are still far from widespread deployment and adoption. The largest obstacle is having efficient models that are feasible on a wide variety of consumer hardware. Comparative research and evaluation is difficult due to the lack of standard benchmarking platforms and due to variations in hardware architectures and test environments. Through our rate-distortion-computation (RDC) study we demonstrate that neither floating-point operations (FLOPs) nor runtime are sufficient on their own to accurately rank neural compression methods. We also explore the RDC frontier, which leads to a family of model architectures with the best empirical trade-off between computational requirements and RD performance. Finally, we identify a novel neural compression architecture that yields state-of-the-art RD performance with rate savings of 23.1% over BPG (7.0% over VTM and 3.0% over ELIC) without requiring significantly more FLOPs than other learning-based codecs.
更多查看译文
关键词
image compression,neural networks,FLOPs,runtime
AI 理解论文
溯源树
样例
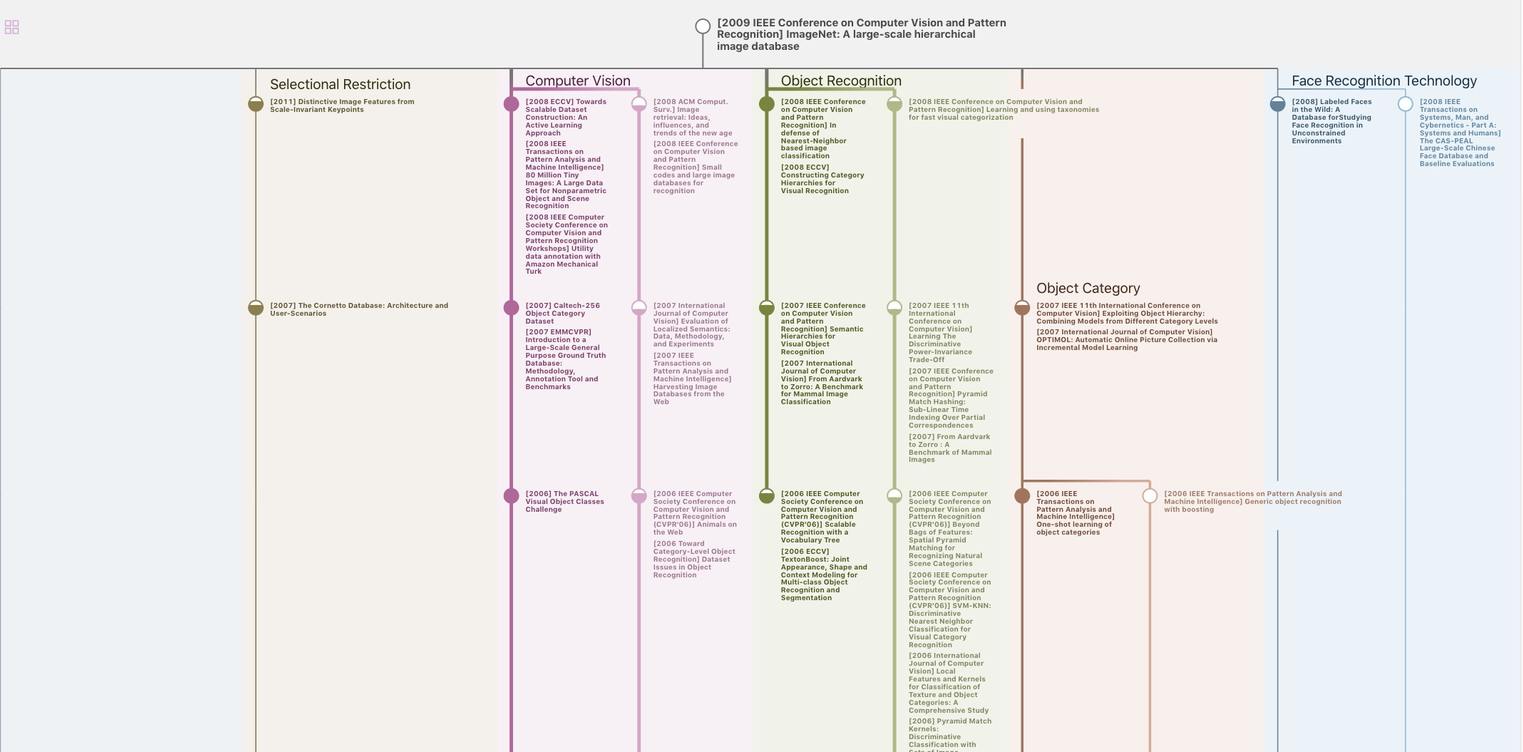
生成溯源树,研究论文发展脉络
Chat Paper
正在生成论文摘要