Training Deep 3D Convolutional Neural Networks to Extract BSM Physics Parameters Directly from HEP Data: a Proof-of-Concept Study Using Monte Carlo Simulations.
CoRR(2023)
摘要
We report on a novel application of computer vision techniques to extract beyond the Standard Model (BSM) parameters directly from high energy physics (HEP) flavor data. We develop a method of transforming angular and kinematic distributions into "quasi-images" that can be used to train a convolutional neural network to perform regression tasks, similar to fitting. This contrasts with the usual classification functions performed using ML/AI in HEP. As a proof-of-concept, we train a 34-layer Residual Neural Network to regress on these images and determine the Wilson Coefficient $C_{9}$ in MC (Monte Carlo) simulations of $B \rightarrow K^{*}\mu^{+}\mu^{-}$ decays. The technique described here can be generalized and may find applicability across various HEP experiments and elsewhere.
更多查看译文
AI 理解论文
溯源树
样例
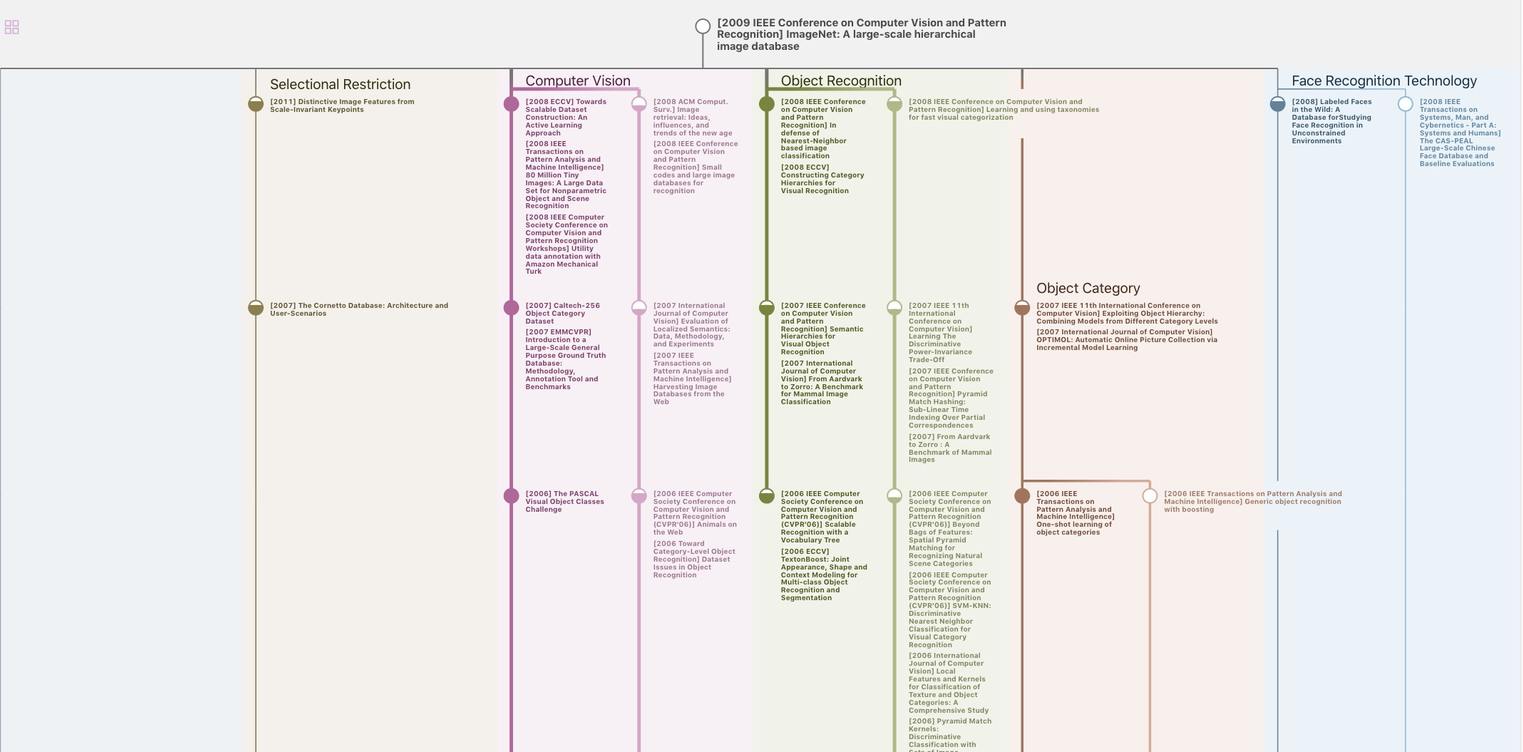
生成溯源树,研究论文发展脉络
Chat Paper
正在生成论文摘要