Poster: Query-efficient Black-box Attack for Image Forgery Localization via Reinforcement Learning.
CCS '23: Proceedings of the 2023 ACM SIGSAC Conference on Computer and Communications Security(2023)
摘要
Recently, deep learning has been widely used in forensics tools to detect and localize forgery images. However, its susceptibility to adversarial attacks highlights the need for the exploration of anti-forensics research. To achieve this, we introduce an innovative and query-efficient black-box anti-forensics framework tailored for the generation of adversarial forgery images. This framework is designed to simulate the query dynamics of online forensic services, utilizing a Markov Decision Process formulation within the paradigm of reinforcement learning. We further introduce a novel reward function, which evaluates the efficacy of attacks based on the disjunction between query results and attack targets. To improve the query efficiency of these attacks, an actor-critic algorithm is employed to maximize cumulative rewards. Empirical findings substantiate the efficacy of our proposed methodology. Specifically, it demonstrates pronounced adversarial effects on a range of prevailing image forgery detectors, while ensuring negligible visually perceptible distortions in the resultant anti-forensics images.
更多查看译文
AI 理解论文
溯源树
样例
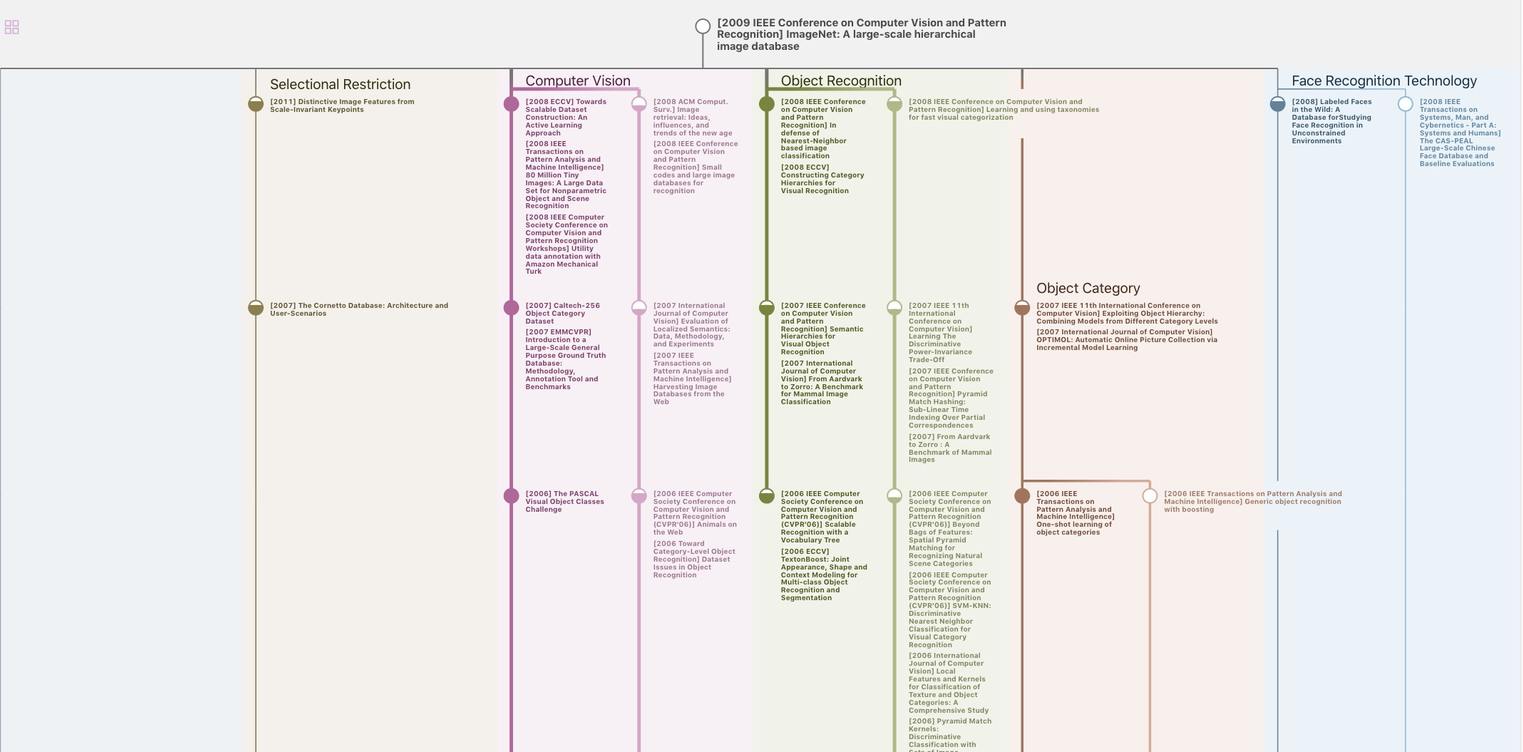
生成溯源树,研究论文发展脉络
Chat Paper
正在生成论文摘要