"Get in Researchers; We're Measuring Reproducibility": A Reproducibility Study of Machine Learning Papers in Tier 1 Security Conferences.
CCS '23: Proceedings of the 2023 ACM SIGSAC Conference on Computer and Communications Security(2023)
摘要
Reproducibility is crucial to the advancement of science; it strengthens confidence in seemingly contradictory results and expands the boundaries of known discoveries. Computer Security has the natural benefit of creating artifacts that should facilitate computational reproducibility, the ability for others to use someone else's code and data to independently recreate results, in a relatively straightforward fashion. While the Security community has recently increased its attention on reproducibility, an independent and comprehensive measurement of the current state of reproducibility has not been conducted. In this paper, we perform the first such study, targeting reproducible artifacts generated specifically by papers on machine learning security (one of the most popular areas in academic research) published in Tier 1 security conferences over the past ten years (2013-2022). We perform our measurement study of indirect and direct reproducibility over nearly 750 papers, their codebases, and datasets. Our analysis shows that there is no statistically significant difference between the availability of artifacts before and after the introduction of Artifact Evaluation Committees in Tier 1 conferences. However, based on three years of results, artifacts that pass through this process work at a higher rate than those that do not. From our collected findings, we offer data-driven suggestions for improving reproducibility in our community, including five common problems observed in our study. In so doing, we demonstrate that significant progress still needs to be made in computational reproducibility in Computer Security research.
更多查看译文
AI 理解论文
溯源树
样例
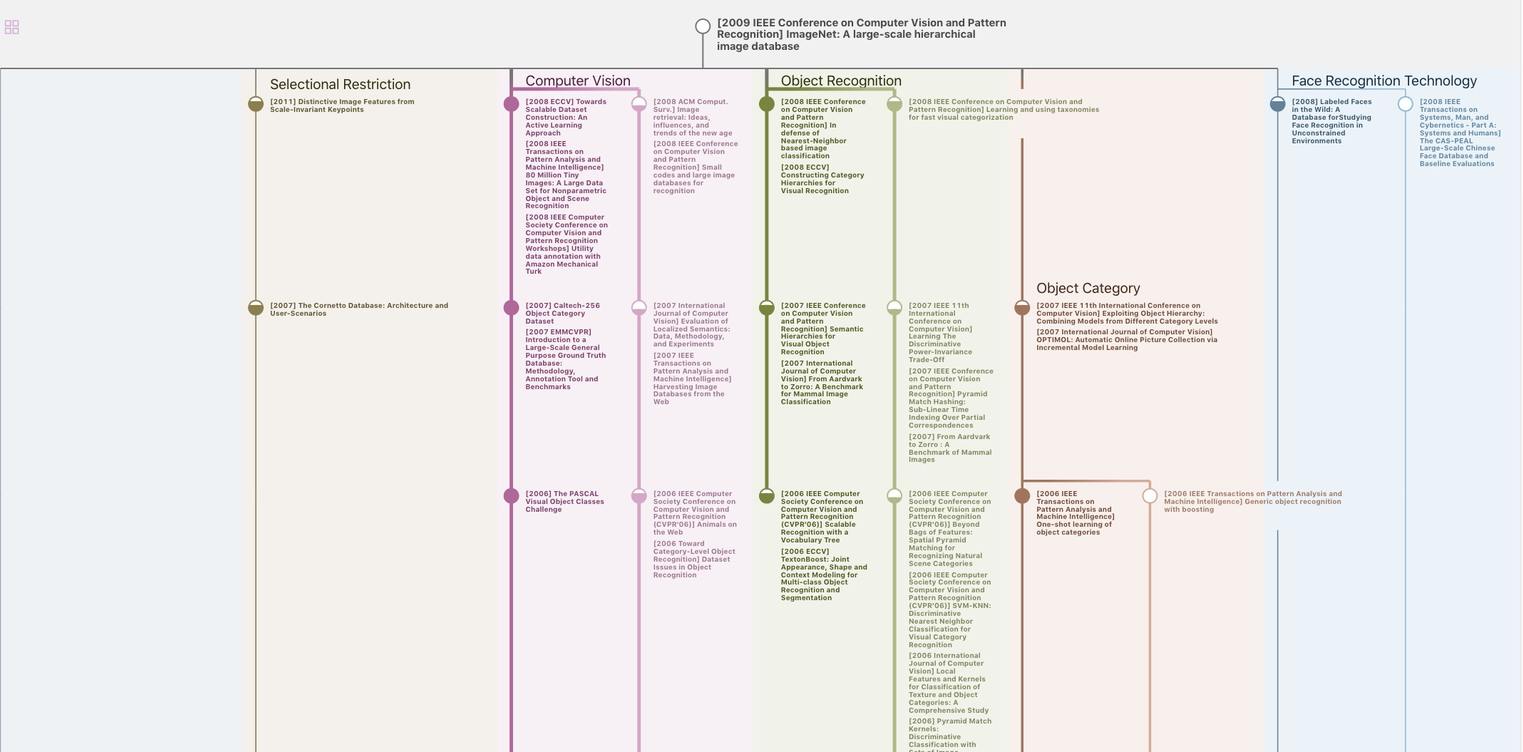
生成溯源树,研究论文发展脉络
Chat Paper
正在生成论文摘要