FGT-SAMK-NN: impact of the right to be forgotten using a lazy algorithm in data stream learning.
ICEGOV '23: Proceedings of the 16th International Conference on Theory and Practice of Electronic Governance(2023)
摘要
“Right to Be Forgotten" is guaranteed by new international regulations on personal management data. This means that individuals can request the erasure of their data from third-party tools and services. However, this poses a challenge for machine learning estimators, who will need to forget parts of their knowledge. This paper examines the impact of learning and forgetting policies in Data Stream Learning. Storing data or retraining learning models from scratch in data stream mining is usually not feasible due to the large volume of instances. Therefore, more efficient solutions are necessary to deal with the dynamic nature of online machine learning. To address this issue, we implemented FGT-SAMK-NN, an incremental version of one of the most knowledgeable algorithms in Data Stream lazy algorithms: The SAMK-NN classifier. FGT-SAMK-NN can erase its past data, and we investigate the impact of data forgetting on predictive performance. Our proposal is compared to the original SAMK-NN algorithm using four non-stationary stream datasets. Our results demonstrate that evaluation metrics did not undergo significant changes, which may support the idea that has a good architecture for adaptations of the proposed nature. However, it was also noted that the processing time is very high for cases involving more forgettings, which may indicate that the high complexity of the model creates conflicts if the pattern of data streams, where the algorithm is used, involves a high forgetfulness rate.
更多查看译文
AI 理解论文
溯源树
样例
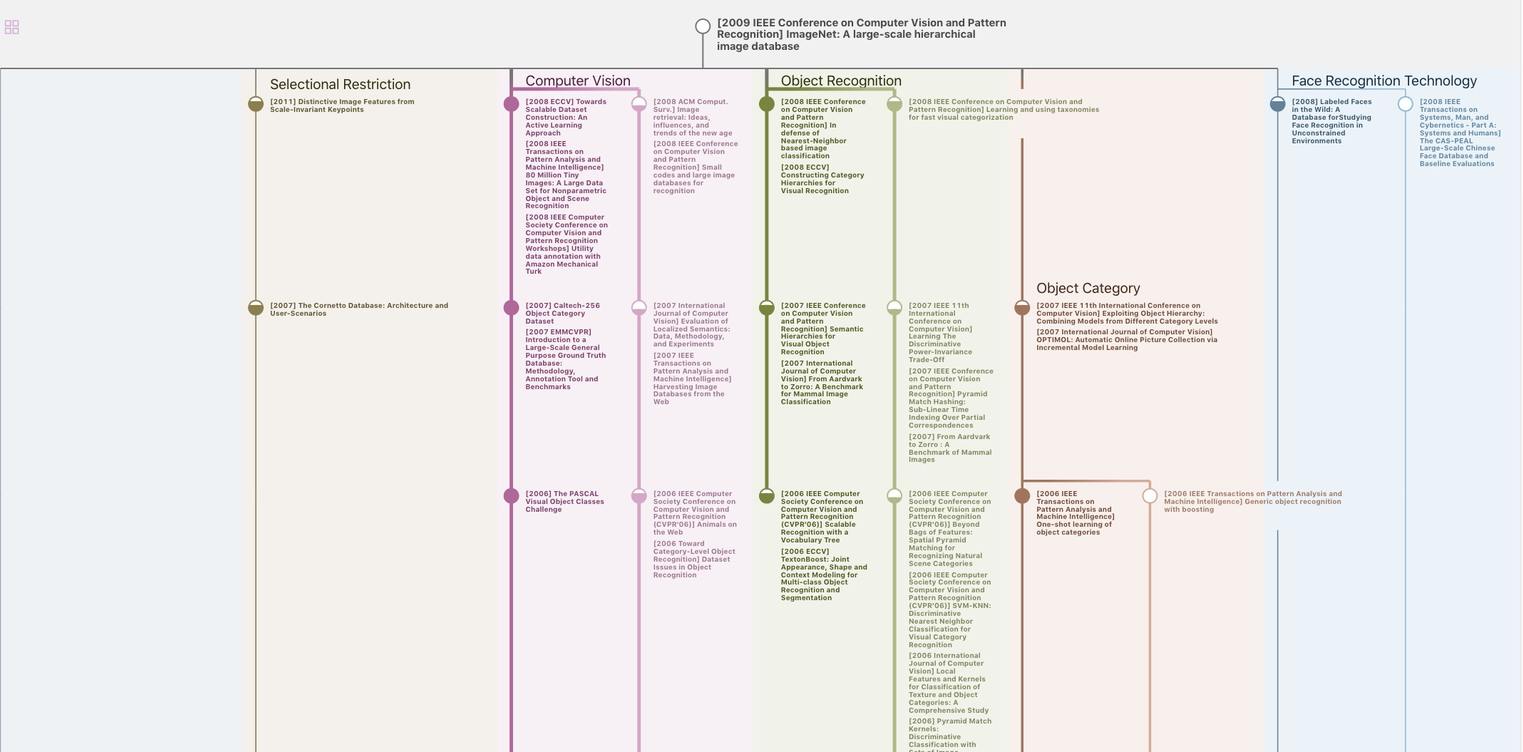
生成溯源树,研究论文发展脉络
Chat Paper
正在生成论文摘要