Study on Lightweight and Efficient Deep Image Compression Method Based on MBConv
PROCEEDINGS OF 2023 THE 7TH INTERNATIONAL CONFERENCE ON GRAPHICS AND SIGNAL PROCESSING, ICGSP(2023)
摘要
There have been many studies on image compression using deep learning methods. The most of these studies have focused mainly on increasing the image compression ratio. However, for effective implementation in a wide range of mobile devices, it is crucial to also develop lightweight network architectures that can cope with the limited computational resources and power constraints of these devices. We have proposed three image compression methods based on the MBConv (Mobile inverted Bottleneck Convolution) architecture, which is proposed for image recognition tasks in mobile devices. The main goal of this research is to improve the performance of image compression while at the same time reducing the computational complexity of the network. To validate the effectiveness of the proposed method, we constructed a network based on previous research and conducted a series of experiments. Then, using Rate-Distortion curves, we compared the performance of the proposed method with that of a comparison method. The results showed that all three proposed methods improve the peak signal-to-noise ratio (PSNR), an objective evaluation metric, especially at medium and low bit rates. In addition, the proposed methods were shown to successfully reduce the number of parameters in the network by up to 22%.
更多查看译文
关键词
Deep learning,MBConv,Image compression
AI 理解论文
溯源树
样例
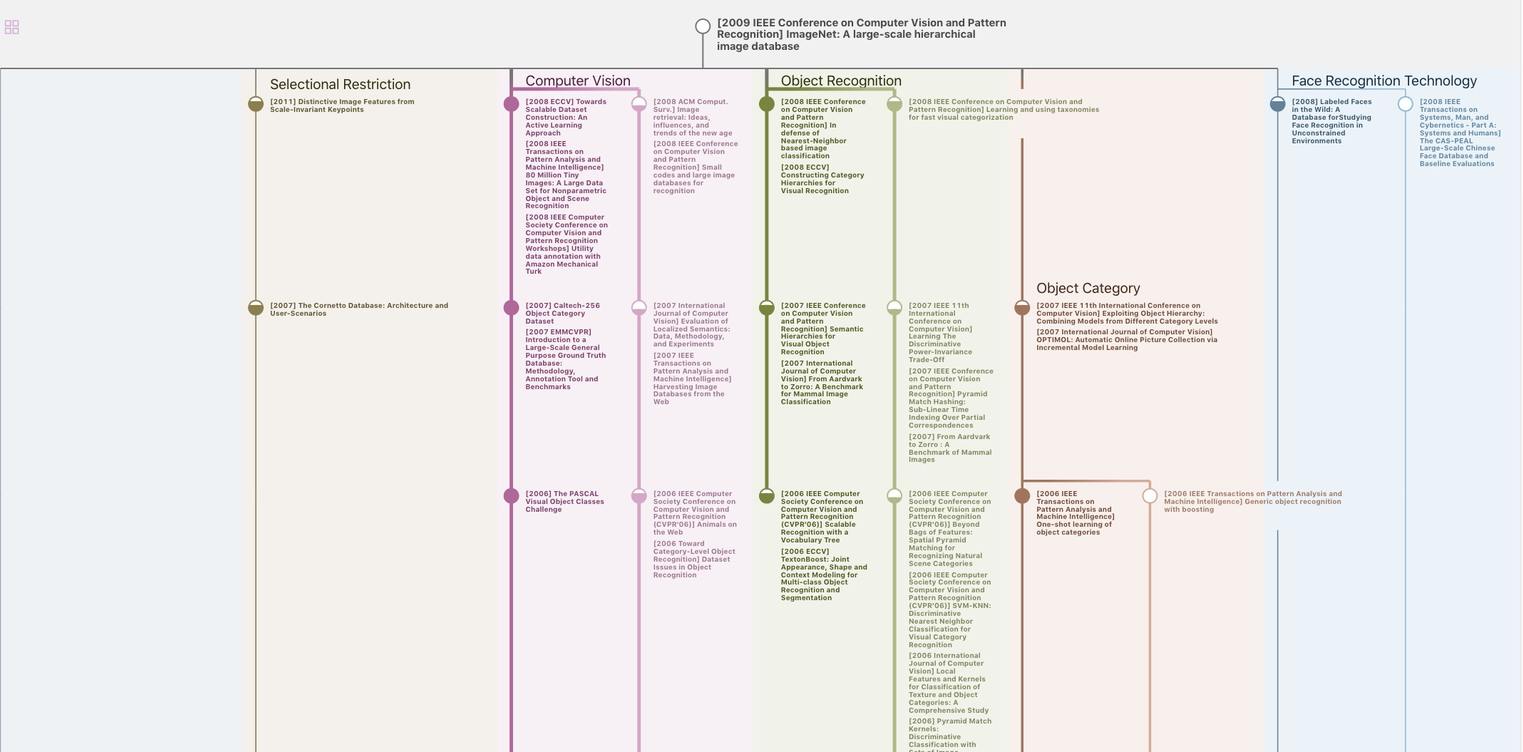
生成溯源树,研究论文发展脉络
Chat Paper
正在生成论文摘要