Predicting single cell genotypes from single cell expression profiles in AML using deep learning
2023 13TH INTERNATIONAL CONFERENCE ON BIOSCIENCE, BIOCHEMISTRY AND BIOINFORMATICS, ICBBB 2023(2023)
摘要
Acute myeloid leukemia (AML) is an aggressive hematologic malignancy composed of a mixture of genotypically, phenotypically and functionally diverse cell populations including wild-type (WT) cells. The generation of high throughput single cell gene expression and mutational profiles in AML enables the deployment of deep learning frameworks for gaining insights on how genotypic changes are associated with disease phenotypes. However, the question if the single cell gene expression patterns together with the computational power of neural networks have the capacity to predict a cell's genotype remains unclear. In this study, we train two supervised deep learning models to predict the cell's malignant or wild-type (WT) status as well as the mutational status of specific genomic abnormalities in a binary and multi-class multi-label setting respectively, based on single cell RNA sequencing data from 6 AML patients and 4 healthy individuals. In the independent test sets, the binary classification model achieved an accuracy of 98% while the multi-class multi-label model achieved a macro-average AUC ROC of 0.84. Moreover, applying black box feature selection on the trained networks identified genes involved in biological processes and pathways of reported significance in AML, such as the IL-2/STAT5 and NF-kB signaling pathways. Overall, this study proposes two deep learning tasks for the prediction of single cell genotypic profiles from single cell expression data and showcases howthe trained models can be used for the derivation of biologically related signals.
更多查看译文
关键词
Single cell RNA-sequencing,Deep learning,Acute Myeloid Leukemia
AI 理解论文
溯源树
样例
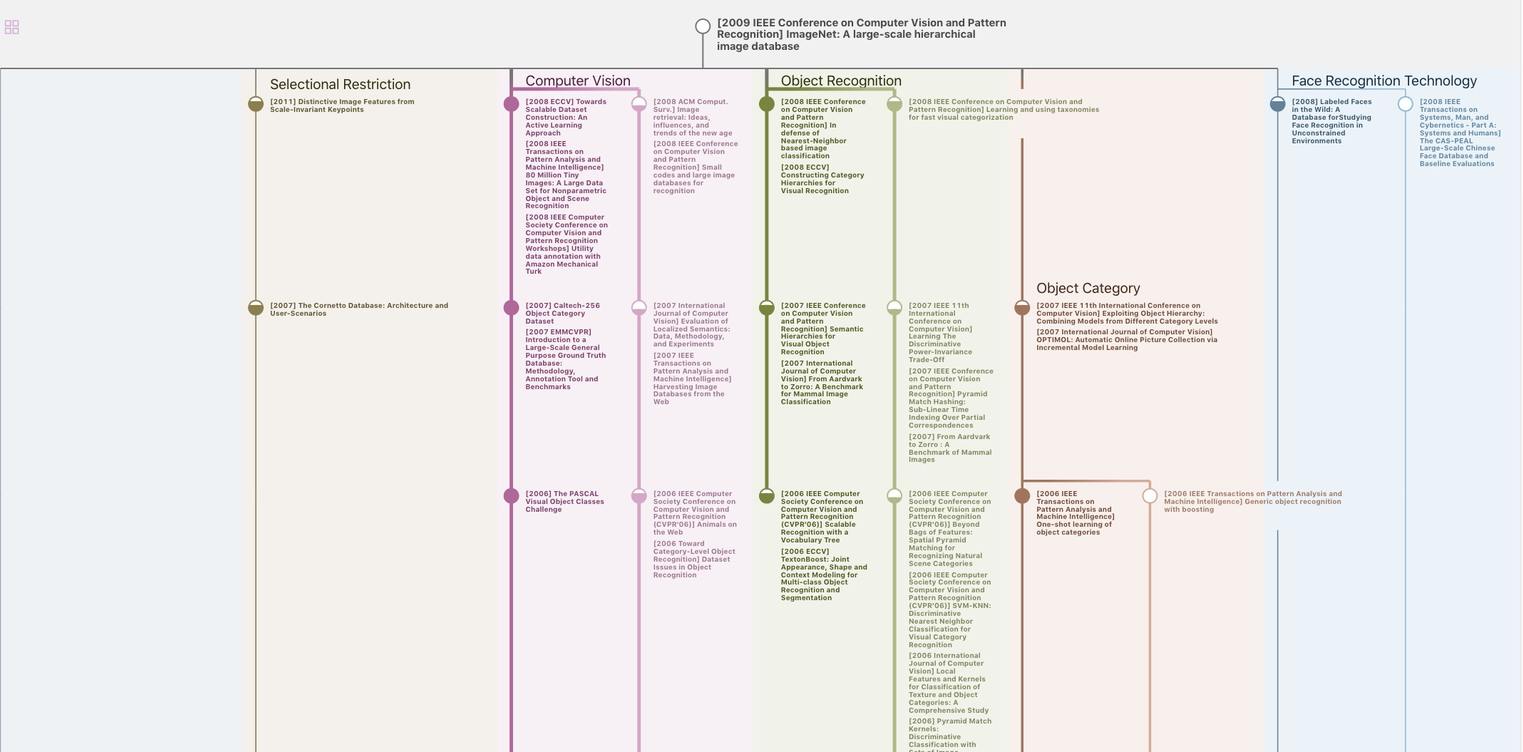
生成溯源树,研究论文发展脉络
Chat Paper
正在生成论文摘要