A Compact and Semantic Latent Space for Disentangled and Controllable Image Editing
20TH ACM SIGGRAPH EUROPEAN CONFERENCE ON VISUAL MEDIA PRODUCTION, CVMP 2023(2023)
Abstract
Recent advances in the field of generative models and in particular generative adversarial networks (GANs) have lead to substantial progress for controlled image editing. Despite their powerful ability to apply realistic modifications to an image, these methods often lack properties such as disentanglement (the capacity to edit attributes independently). In this paper, we propose an auto-encoder which re-organizes the latent space of StyleGAN, so that each attribute which we wish to edit corresponds to an axis of the new latent space, and furthermore that the latent axes are decorrelated, encouraging disentanglement. We work in a compressed version of the latent space, using Principal Component Analysis, meaning that the parameter complexity of our autoencoder is reduced, leading to short training times (similar to 45 mins). Qualitative and quantitative results demonstrate the editing capabilities of our approach, with greater disentanglement than competing methods, while maintaining fidelity to the original image with respect to identity. Our autoencoder architecture is simple and straightforward, facilitating implementation.
MoreTranslated text
Key words
Image Editing,Disentanglement,GANs,Latent navigation,Neural Networks,Generative Models
AI Read Science
Must-Reading Tree
Example
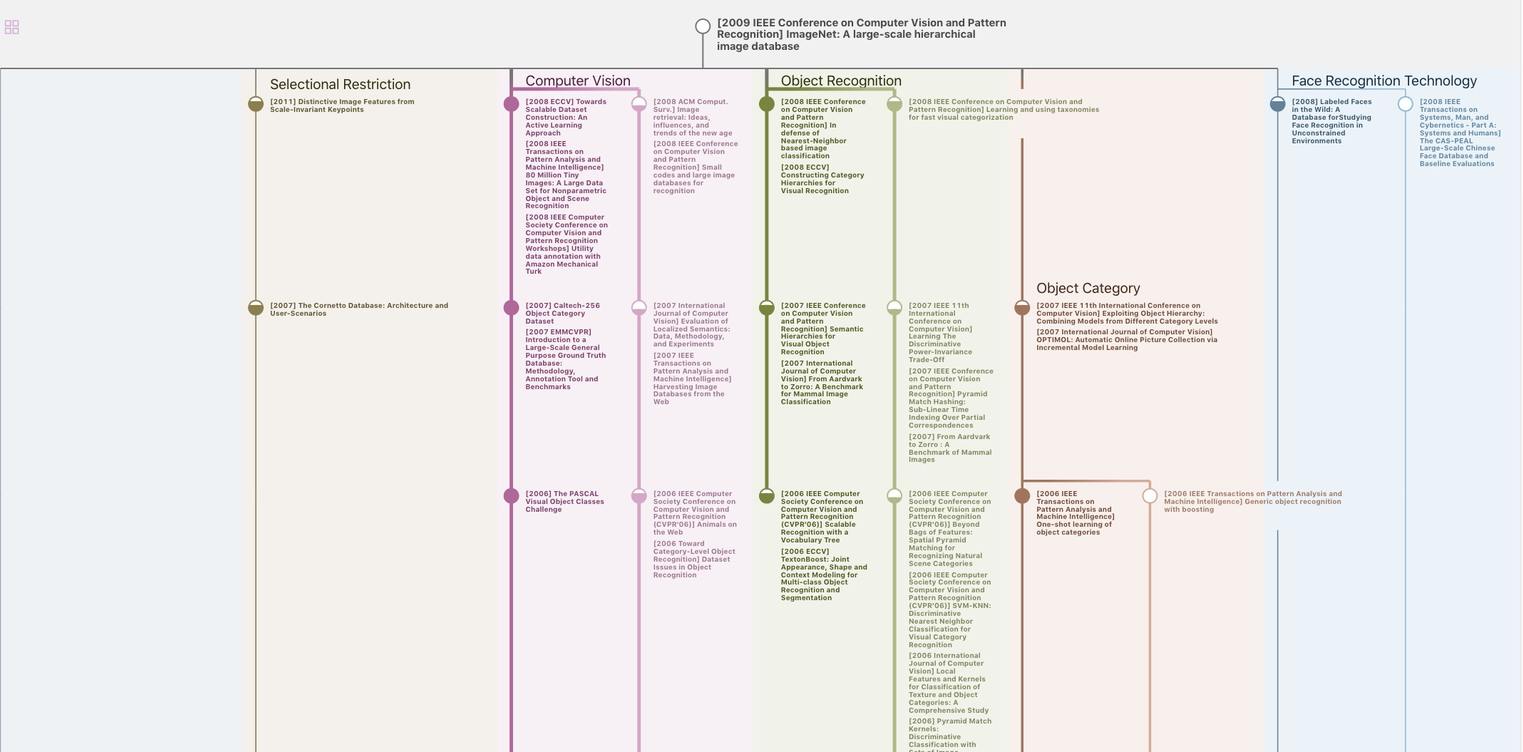
Generate MRT to find the research sequence of this paper
Chat Paper
Summary is being generated by the instructions you defined