Deep Learning in IoT: An LSTM Approach for NDVI Forecasting
2023 3rd International Conference on Electronic Engineering (ICEEM)(2023)
摘要
This study presents a novel application of a Long Short-Term Memory (LSTM) deep learning model for time-series analysis of the Normalized Difference Vegetation Index (NDVI) from January 1, 1984, to April 21, 2023. As remote sensing technologies generate substantial environmental data, advanced analytics like LSTM provide essential tools for precise interpretation and forecasting. Through grid search optimization, hyperparameters were fine-tuned for optimal LSTM performance. The NDVI mean value over the study period is 0.332, indicative of a moderate vegetation presence. The data series’ sta-tionarity, confirmed through the Dickey-Fuller test, contributes to accurate prediction outcomes. The LSTM model demonstrates superior predictive performance, evidenced by the Root Mean Squared Error (RMSE) values of 0.000764 and 0.000900 for the training and testing datasets respectively. The high R-squared and correlation values further substantiate its efficacy. This study paves the way for leveraging LSTM models in large-scale NDVI data analysis, contributing to environmental monitoring, climate change tracking, and vegetation health assessments. Future work can extend this model to other remote sensing indices and explore various deep learning architectures for enhanced predictive accuracy. The main objective is to identify the optimal LSTM hyperparameters for NDVI prediction using grid search optimization. Our results are expected to provide valuable insights into how LSTM models can be effectively tuned for improved NDVI prediction, potentially benefiting environmental monitoring and decision-making processes.
更多查看译文
关键词
Forecasting,Mathematical model,Grid search,NDVI,dynamic system,Iot,and crops
AI 理解论文
溯源树
样例
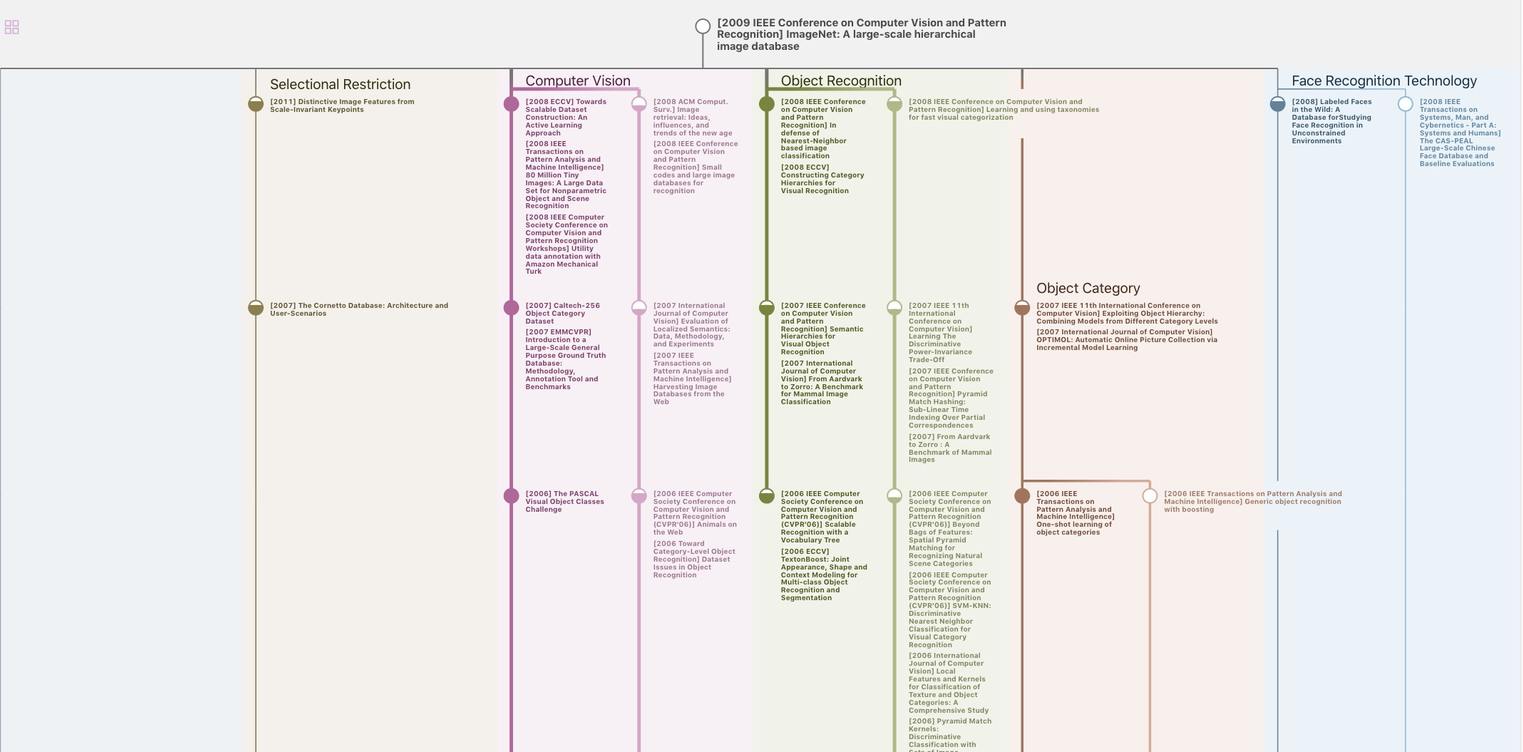
生成溯源树,研究论文发展脉络
Chat Paper
正在生成论文摘要