Constructing New Backbone Networks via Space-Frequency Interactive Convolution for Deepfake Detection
IEEE TRANSACTIONS ON INFORMATION FORENSICS AND SECURITY(2024)
Abstract
The serious concerns over the negative impacts of Deepfakes have attracted wide attentions in the community of multimedia forensics. The existing detection works achieve deepfake detection by improving the traditional backbone networks to capture subtle manipulation traces. However, there is no attempt to construct new backbone networks with different structures for Deepfake detection by improving the internal feature representation of convolution. In this work, we propose a novel Space-Frequency Interactive Convolution (SFIConv) to efficiently model the manipulation clues left by Deepfake. To obtain high-frequency features from tampering traces, a Multichannel Constrained Separable Convolution (MCSConv) is designed as the component of the proposed SFIConv, which learns space-frequency features via three stages, namely generation, interaction and fusion. In addition, SFIConv can replace the vanilla convolution in any backbone networks without changing the network structure. Extensive experimental results show that seamlessly equipping SFIConv into the backbone network greatly improves the accuracy for Deepfake detection. In addition, the space-frequency interaction mechanism does benefit to capturing common artifact features, thus achieving better results in cross-dataset evaluation. Our code will be available at https://github.com/EricGzq/SFIConv.
MoreTranslated text
Key words
Deepfake detection,space-frequency interactive convolution,backbone network,manipulation traces
AI Read Science
Must-Reading Tree
Example
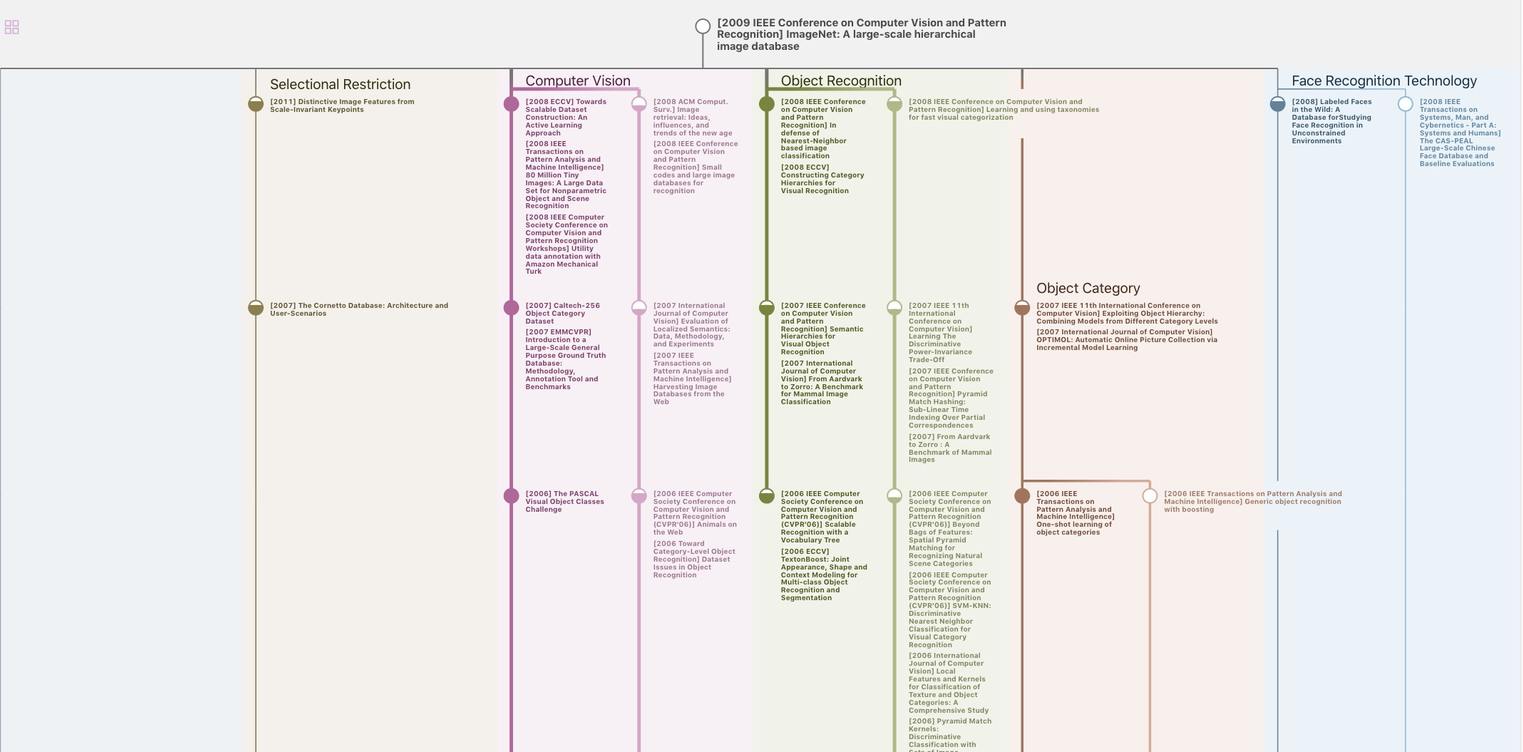
Generate MRT to find the research sequence of this paper
Chat Paper
Summary is being generated by the instructions you defined