Heavy Hitter Identification Over Large-Domain Set-Valued Data With Local Differential Privacy
IEEE TRANSACTIONS ON INFORMATION FORENSICS AND SECURITY(2024)
摘要
Set-valued data are widely used to represent information in the real word, such as individual daily behaviors, items in shopping carts and web browsing history. By collecting set-valued data and identifying heavy hitters, service providers (i.e., the collector) can learn usage preferences of costumers (i.e., users), and improve the quality of their services by the learned information. However, the collection of raw data would bring privacy risks to users. Recently, local differential privacy (LDP) has emerged as a rigorous privacy framework for user private data collection. At the same time, many LDP schemes have been designed to achieve heavy hitters, but most of them are limited by the large data domain due to the huge computation cost. In this paper, we propose an LDP framework: PemSet, to efficiently identify heavy hitters from set-valued data with a large domain. In PemSet, users mainly focus on the prefix of each item (i.e., the first few bits of the binary expression of each item), and only perturb and report prefixes to reduce computation cost. Sometimes the prefixes of different items are the same, so the reported set-valued data could be a multiset, i.e., a set including multiple same items. As such, we design four LDP protocols MOLH, MOLH-S, MPCKV, MWheel to estimate frequencies of items in the multiset setting, and compare their performance under PemSet framework by experiments. Experimental results demonstrate that MOLH can perform the best in a high privacy region, i.e., epsilon < 1, while MWheel can obtain the highest utility when privacy budget is large, i.e., epsilon >= 1 .
更多查看译文
关键词
Local differential privacy,heavy hitter,set-valued data collection
AI 理解论文
溯源树
样例
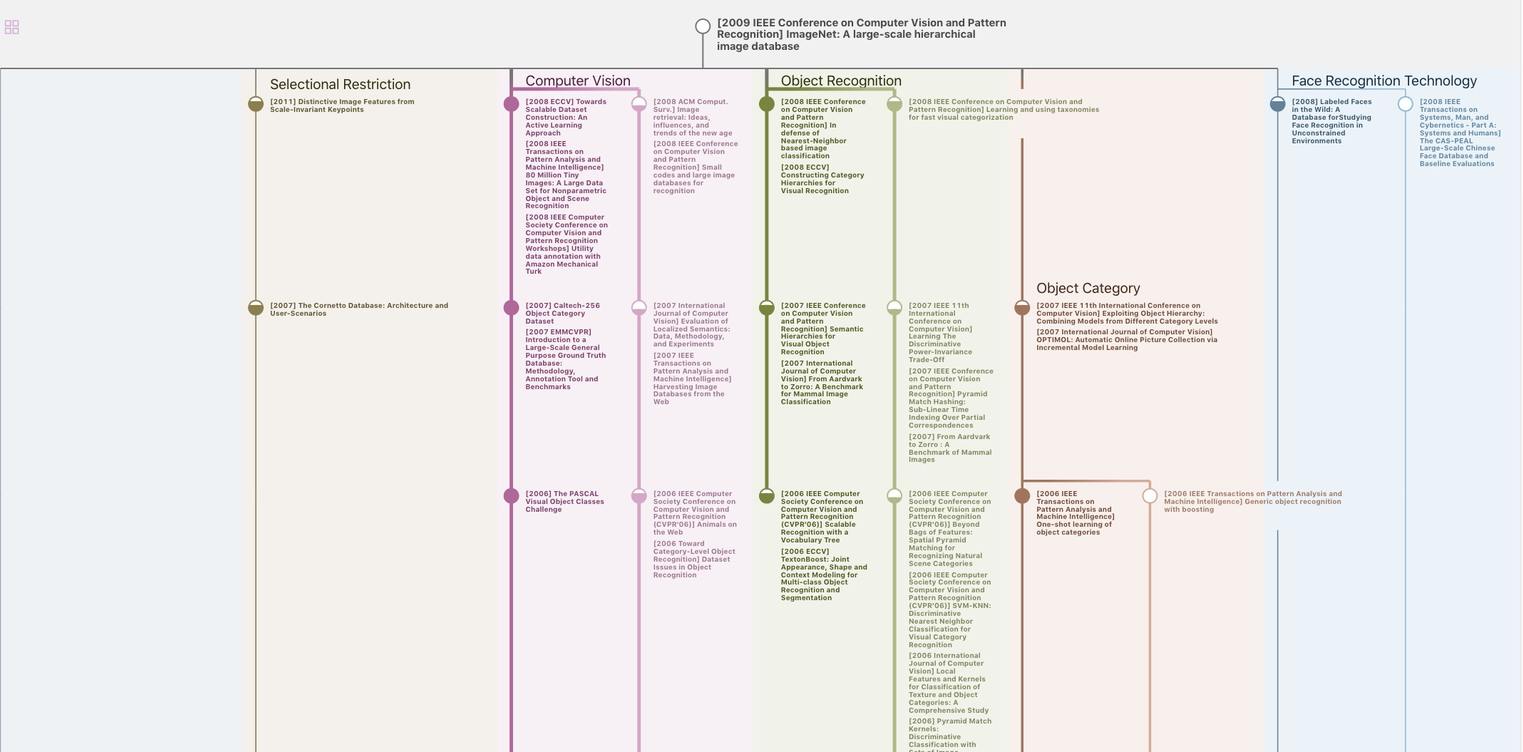
生成溯源树,研究论文发展脉络
Chat Paper
正在生成论文摘要