A Robust Continuous Authentication System Using Smartphone Sensors and Wasserstein Generative Adversarial Networks
Security and Communication Networks(2023)
Abstract
Since the continuous authentication (CA) system based on smartphone sensors has been facing the challenge of the low-data regime under some practical scenarios, which leads to low accuracy of CA, it needs to be solved urgently. To this end, currently, the generative adversarial networks (GAN) provide a powerful method to train the result generative model that could generate very convincing verisimilar data. The framework of the GAN and its variants shed much light on improving the performance of CA. Therefore, in this article, we propose a continuous authentication system on smartphones based on a Wasserstein generative adversarial network (WGAN) for sensor data augmentation, which utilizes accelerometers, gyroscopes, and magnetometers of smartphone sensors to sense phone movements caused by user operation behavior. Specifically, based on sensor data under different user activities, the WGAN is used to create additional data in training data for data augmentation. With the augmented data, we design a convolutional neural network to learn and extract deep features from sensor data, and then use four classifiers of RF, OCSVM, DT, and KNN to train these features. Finally, we train and test on the HMOG dataset, and the results show that the EER of the authentication system is between 3.68% and 6.39% on the sensor data with a time window of 2 s.
MoreTranslated text
Key words
robust continuous authentication system,generative adversarial networks,smartphone sensors
AI Read Science
Must-Reading Tree
Example
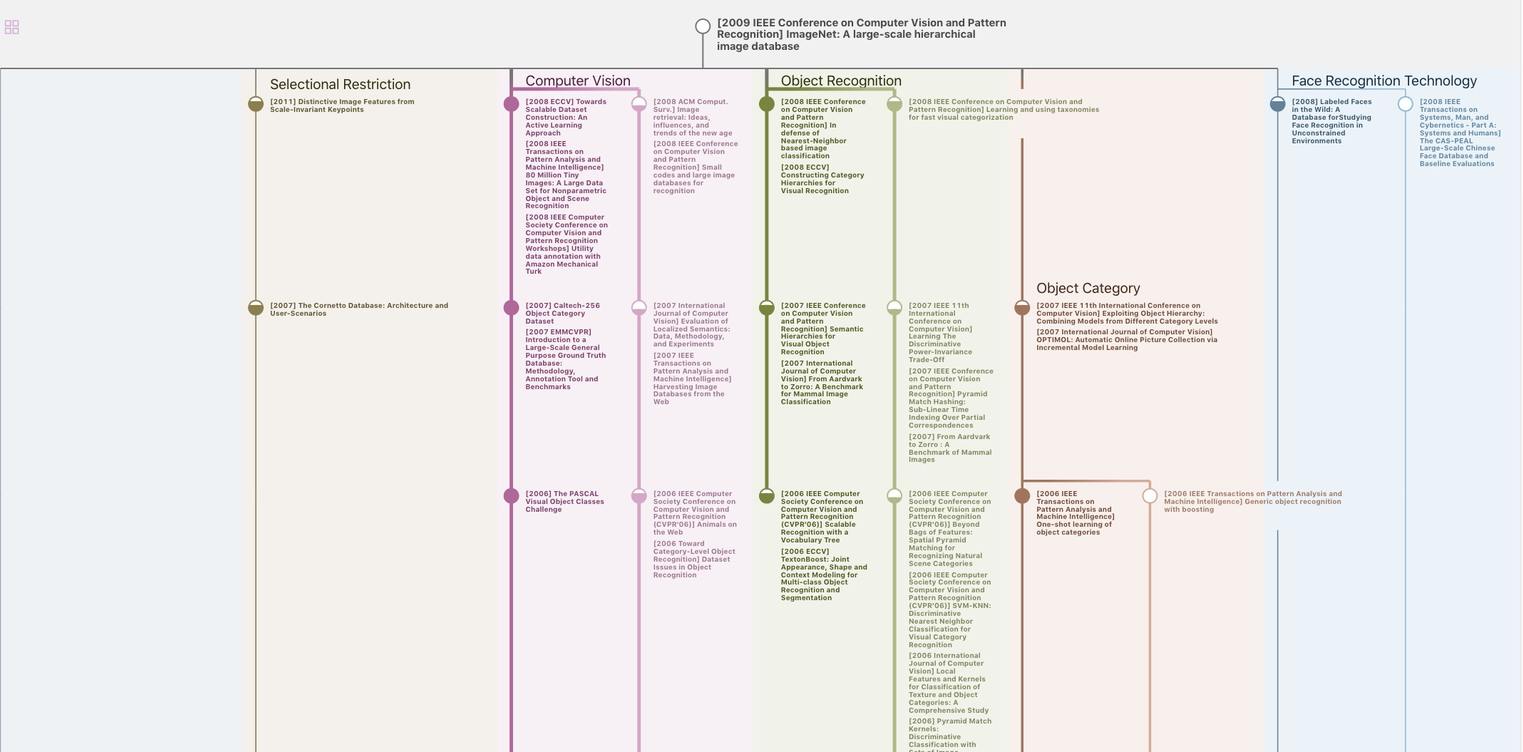
Generate MRT to find the research sequence of this paper
Chat Paper
Summary is being generated by the instructions you defined