Visual Analytics for Generative Transformer Models.
CoRR(2023)
摘要
While transformer-based models have achieved state-of-the-art results in a variety of classification and generation tasks, their black-box nature makes them challenging for interpretability. In this work, we present a novel visual analytical framework to support the analysis of transformer-based generative networks. In contrast to previous work, which has mainly focused on encoder-based models, our framework is one of the first dedicated to supporting the analysis of transformer-based encoder-decoder models and decoder-only models for generative and classification tasks. Hence, we offer an intuitive overview that allows the user to explore different facets of the model through interactive visualization. To demonstrate the feasibility and usefulness of our framework, we present three detailed case studies based on real-world NLP research problems.
更多查看译文
AI 理解论文
溯源树
样例
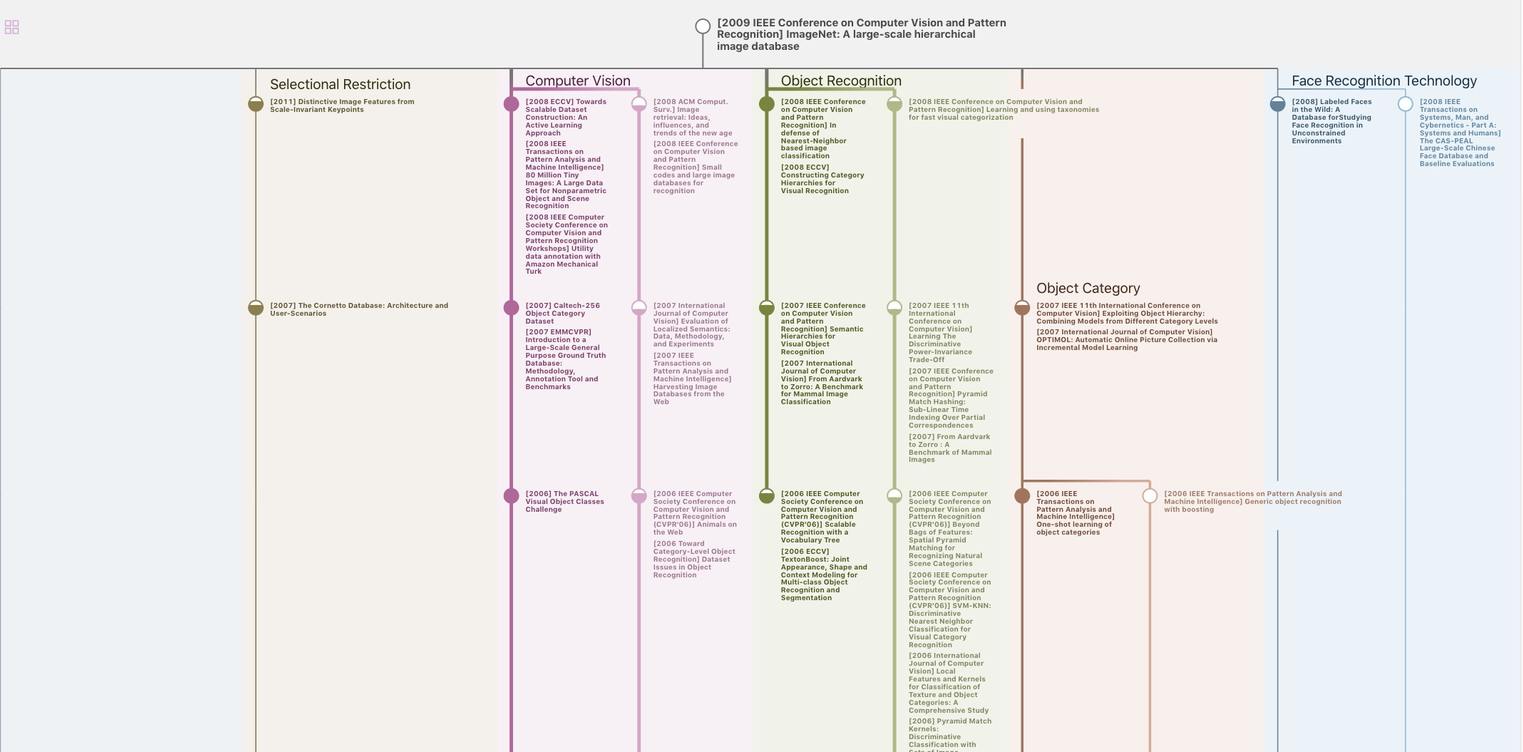
生成溯源树,研究论文发展脉络
Chat Paper
正在生成论文摘要