Journal of Information Security and Applications(2023)
摘要
With the popularity of blockchain, the amount of smart contracts has increased very fast, and the safety of smart contracts has come to more extensive notice. Recently, machine learning technology has been widely applied in vulnerability detection for smart contracts. However, it implements effective smart contract vulnerability detection still faces a major challenge, that is, there is a problem of insufficient labeled data in the current field. Active learning can label data more efficiently. Nevertheless, classical active learning only uses limited labeled data for model training, contrary to the deep learning of a large amount of data required for model training. Because of the above, we provide a new framework, called ASSBert , that leverages active and semi-supervised bidirectional encoder representation from transformers network, which is dedicated to completing the task of smart contract vulnerability classification with a little amount of labeled code data and a large number of unlabeled code data. In our framework, active learning is responsible for selecting highly uncertain code data from unlabeled sol files and putting them into the training set after manual labeling. Besides, semi-supervised learning is charged to continuously pick a certain number of high-confidence unlabeled code data from unlabeled sol files, and put them into the training dataset behind pseudo-labeling. Intuitively, by combining active learning and semi-supervised learning, we are able to get more valuable data to increase the performance of our detection model. In our experiments, we collect our benchmark dataset included 6 vulnerabilities in about 20829 smart contracts. The result of the experiment demonstrates that our framework is superior to the baseline methods with a little amount of labeled code data and a large number of unlabeled code data.
更多查看译文
关键词
Smart contract,Vulnerability detection,Active learning,Semi-supervised learning
AI 理解论文
溯源树
样例
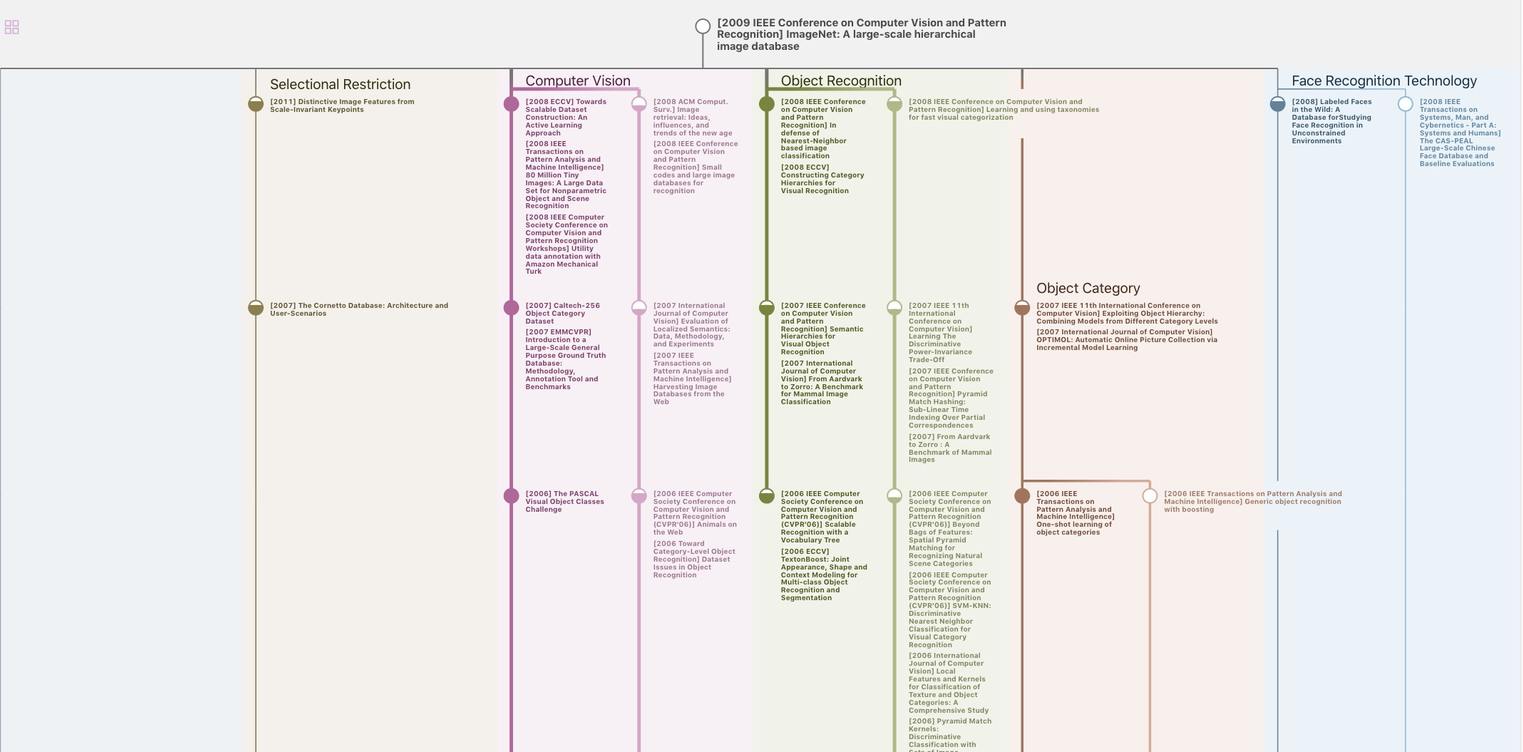
生成溯源树,研究论文发展脉络
Chat Paper
正在生成论文摘要