Efficient processing of
Information Sciences: an International Journal(2022)
摘要
Assisting end users to identify desired results from a large dataset is an important problem for multi-criteria decision making. To address this problem, top- k and skyline queries have been widely adopted, but they both have inherent drawbacks, i.e., the user either has to provide a specific utility function or faces many results. The k -regret minimization query is proposed, which integrates the merits of top- k and skyline queries. Due to the NP-hardness of the problem, the k -regret minimization query is time consuming and the greedy framework is widely adopted. However, formal theoretical analysis of the greedy approaches for the quality of the returned results is still lacking. In this paper, we first fill this gap by conducting a nontrivial theoretical analysis of the approximation ratio of the returned results. To speed up query processing, a sampling-based method, S toc P res G reed , is developed to reduce the evaluation cost. In addition, a theoretical analysis of the required sample size is conducted to bound the quality of the returned results. Finally, comprehensive experiments are conducted on both real and synthetic datasets to demonstrate the efficiency and effectiveness of the proposed methods.
更多查看译文
关键词
Regret minimization,Submodularity ratio,Curvature,Skyline query
AI 理解论文
溯源树
样例
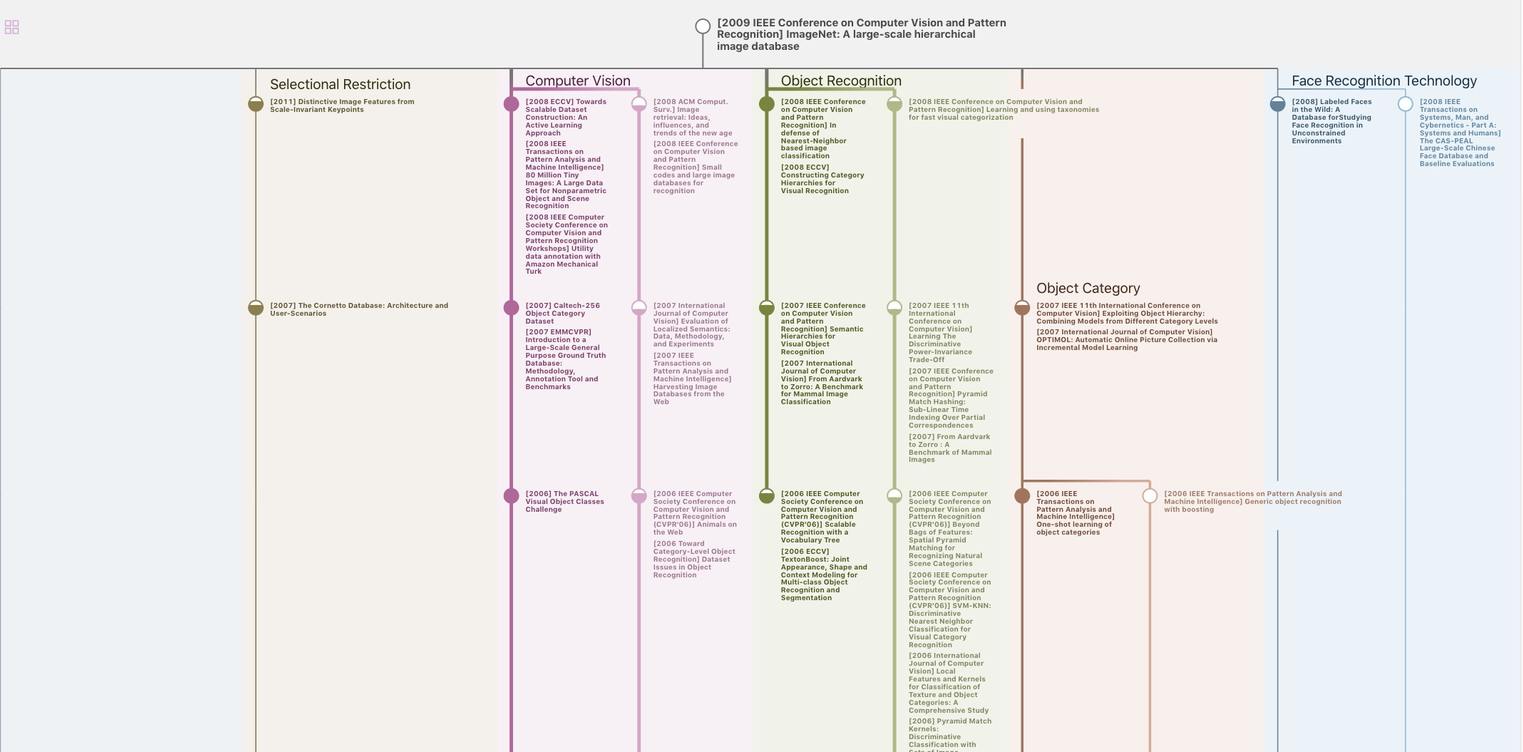
生成溯源树,研究论文发展脉络
Chat Paper
正在生成论文摘要