Constructing a PM2.5 concentration prediction model by combining auto-encoder with Bi-LSTM neural networks
Environmental Modelling & Software(2020)
Abstract
Air pollution problems have a severe effect on the natural environment and public health. The application of machine learning to air pollutant data can result in a better understanding of environmental quality. Of these methods, the deep learning method has proven to be a very efficient and accurate method to forecast complex air quality data. This paper proposes a deep learning model based on an auto-encoder and bidirectional long short-term memory (Bi-LSTM) to forecast PM 2.5 concentrations to reveal the correlation between PM 2.5 and multiple climate variables. The model comprises several aspects, including data preprocessing, auto-encoder layer, and Bi-LSTM layer. The performance of the proposed model was verified based on a real-world air pollution dataset, and the results indicated this model can improve the prediction accuracy in an experimental scenario. • The deep learning neural network is introduced in constructing prediction model for PM2.5 concentration. • The proposed model is based on combining auto-encoder with Bi-LSTM neural networks, namely AE-Bi-LSTM model. • The Auto-encoder layer of the proposed model aims to extract the internal features of pollution data. • The Bi-LSTM layer of the proposed model aims to predict the PM2.5 concentration as a time series problem.
MoreTranslated text
Key words
Deep learning,Auto-encoder,Bi-LSTM,Data preprocessing,PM2.5 concentration prediction,Air pollution
AI Read Science
Must-Reading Tree
Example
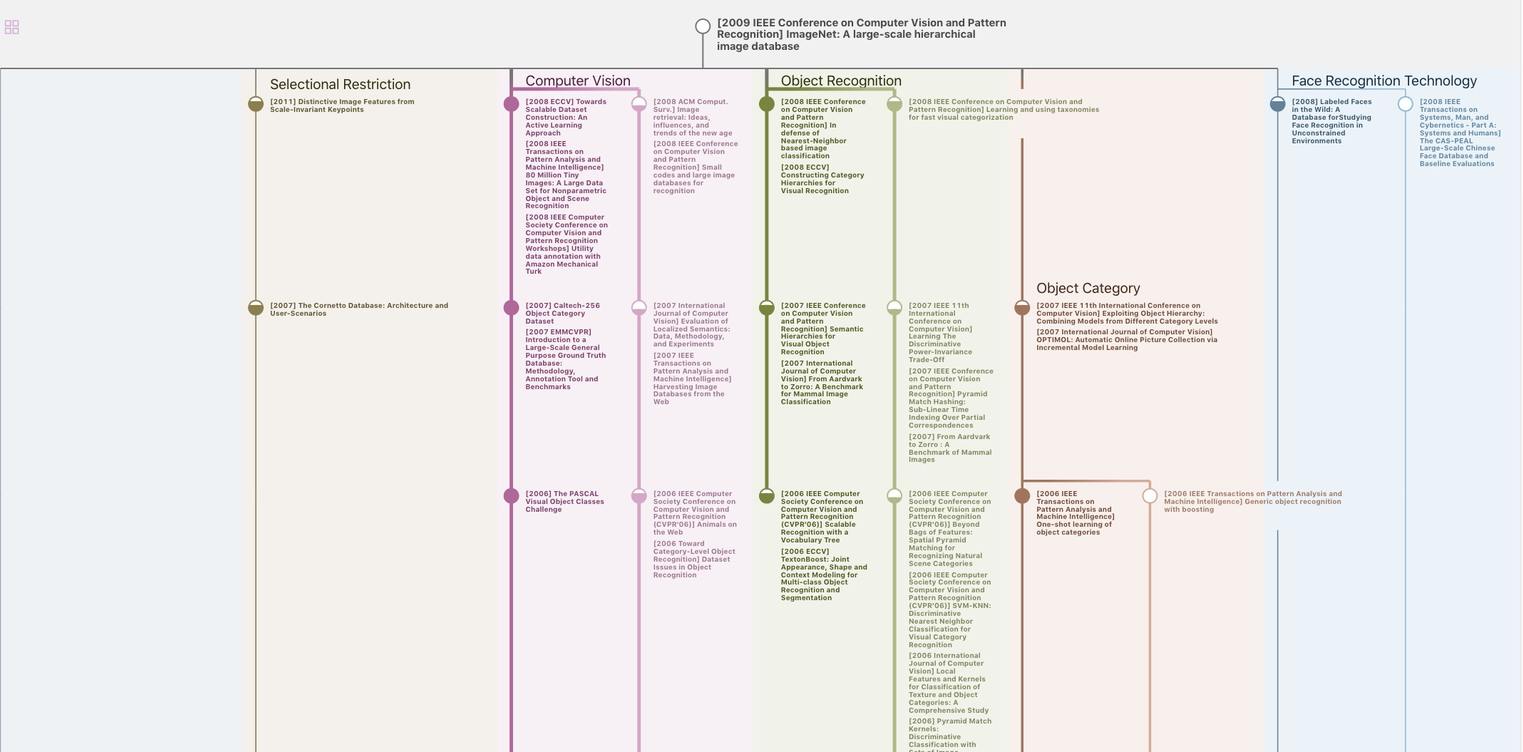
Generate MRT to find the research sequence of this paper
Chat Paper
Summary is being generated by the instructions you defined