ScaleFormer: Transformer-based speech enhancement in the multi-scale time domain
2023 ASIA PACIFIC SIGNAL AND INFORMATION PROCESSING ASSOCIATION ANNUAL SUMMIT AND CONFERENCE, APSIPA ASC(2023)
Abstract
Processing speech at multiple temporal scales greatly improves the performance of automatic speech recognition, but its effect has not been fully exploited in speech enhancement tasks. In this study, we propose a novel Transformer-based neural network termed ScaleFormer, which analyzes speech at multiple temporal resolutions. In ScaleFormer, we utilize an encoder that employs multi-scale convolution to extract different temporal scale features. Then, an intra-scale transformer is used to extract the representation within each scale. After obtaining the output of the intra-scale transformer, an inter-scale transformer is used to model the relationship between multiple scales. All transformer block in ScaleFormer is designed with a dual-path framework to learn short and long-term dependencies. We conduct the experiments on the WSJ0 SI-84 corpus. Experimental results show that our approach outperforms previous representative systems in terms of objective metrics.
MoreTranslated text
AI Read Science
Must-Reading Tree
Example
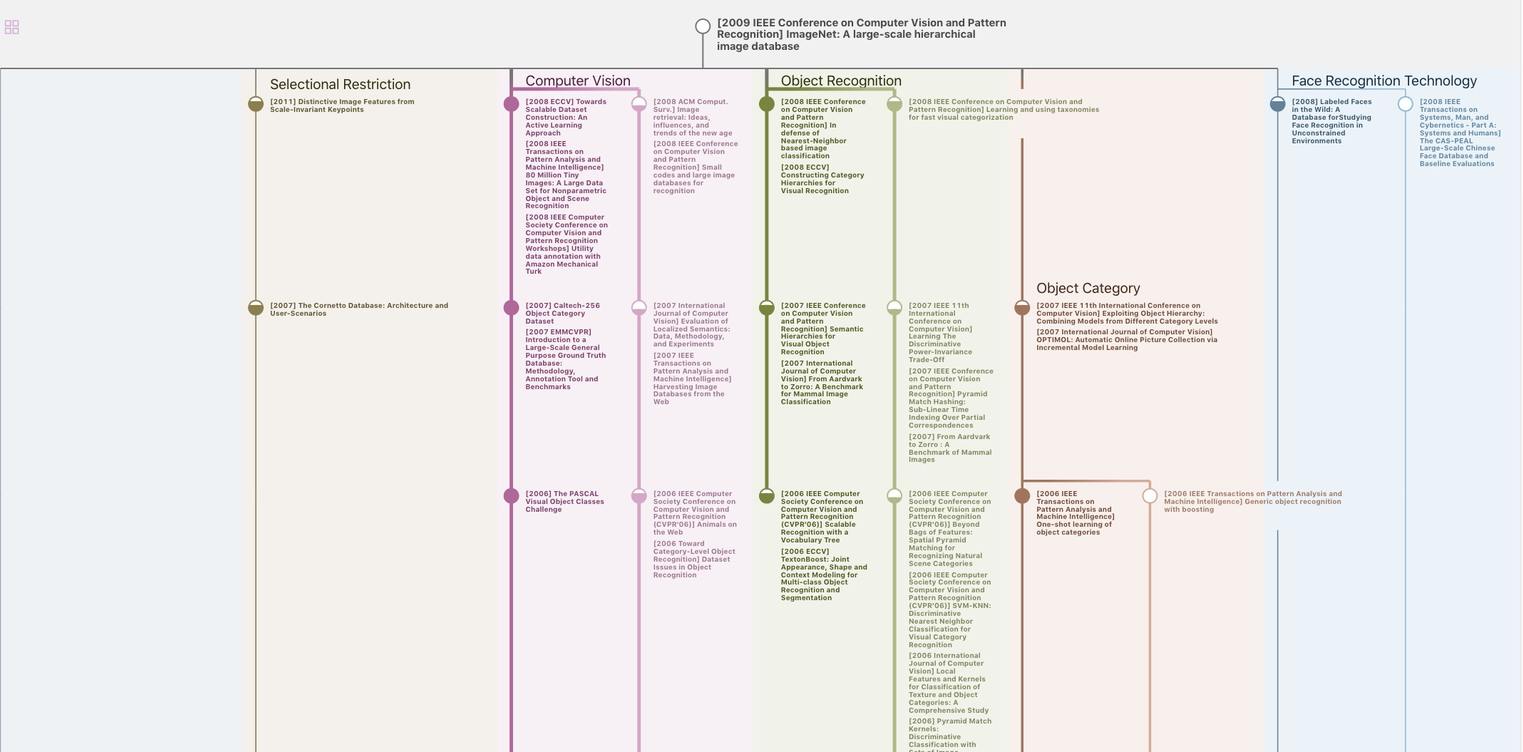
Generate MRT to find the research sequence of this paper
Chat Paper
Summary is being generated by the instructions you defined