Combining multiple end-to-end speech recognition models based on density ratio approach
2023 ASIA PACIFIC SIGNAL AND INFORMATION PROCESSING ASSOCIATION ANNUAL SUMMIT AND CONFERENCE, APSIPA ASC(2023)
摘要
Automatic speech recognition (ASR) systems which involve the use of multiple ASR models, known as "system combination" approaches, have been confirmed by many studies to be effective for improving speech recognition performance. In this study, we propose a system combination method using multiple end-to-end (E2E) ASR models trained under different conditions (i.e., using data from different source domains), in order to perform ASR on an unknown target domain without the need for additional, expensive model training. In order to efficiently adapt existing ASR models to the target domain, we exploit a density ratio approach (DRA), and experimentally demonstrate that by combining ASRs trained with multiple types of acoustic information with an external language model for the target domain, i.e., the standard DRA, our method can more robustly perform ASR for an unknown target domain than a single ASR model trained under one domain condition.
更多查看译文
AI 理解论文
溯源树
样例
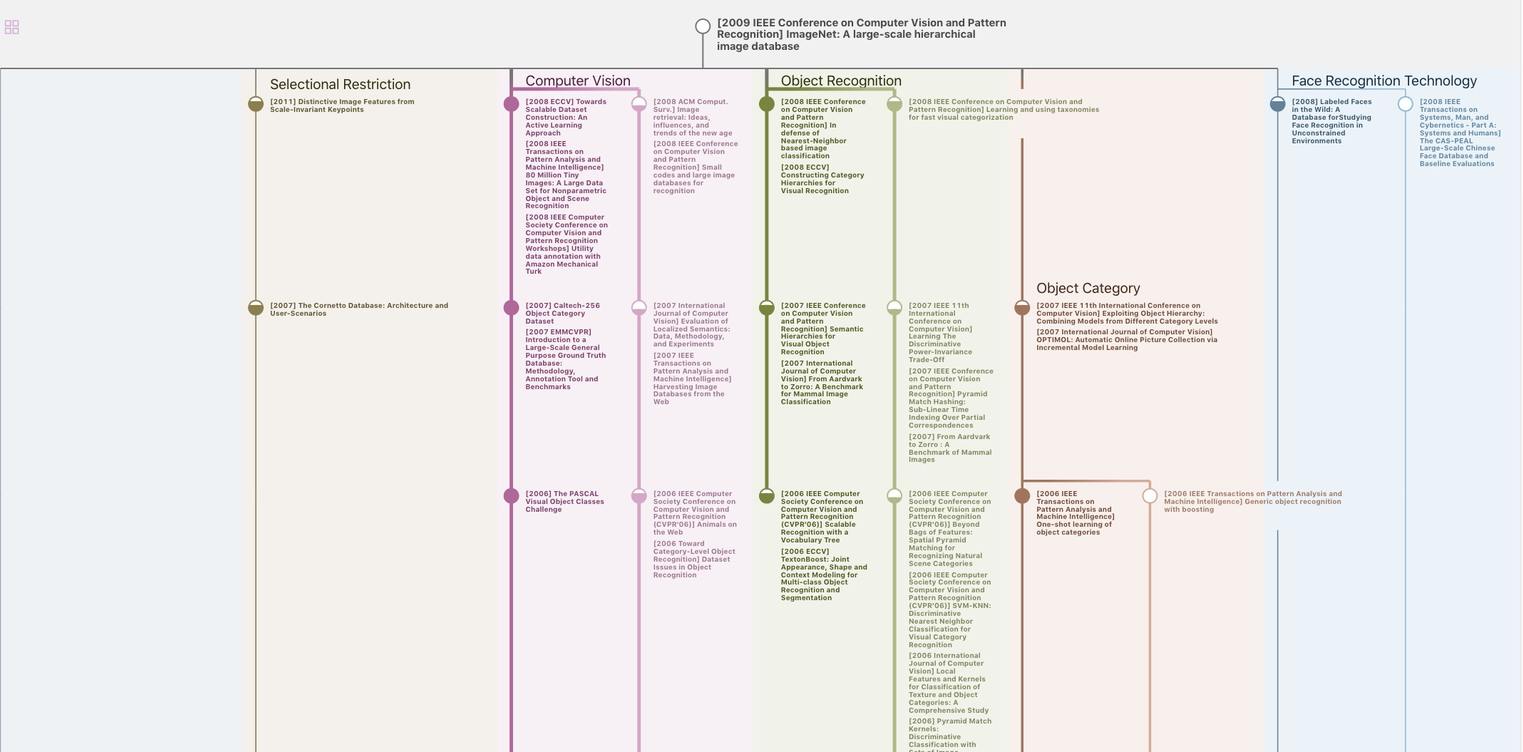
生成溯源树,研究论文发展脉络
Chat Paper
正在生成论文摘要