Learning Multifaceted Self-Similarity for Musical Structure Analysis
2023 ASIA PACIFIC SIGNAL AND INFORMATION PROCESSING ASSOCIATION ANNUAL SUMMIT AND CONFERENCE, APSIPA ASC(2023)
摘要
This paper describes a data-driven music structure analysis (MSA) method that performs segmentation and clustering of musical sections for a music signal. Since the intra-section homogeneity and inter-section difference are important clues for MSA, most studies on MSA have focused on self-similarity matrices (SSMs) computed from various acoustic features of a music signal. The performance of this approach, however, might be limited because the acoustic features used for computing SSMs are designed manually, and multiple SSMs are often integrated in a heuristic manner. To overcome these limitations, we propose a method that learns latent features useful for MSA with a stack of convolution-augmented multi-head self-attention (CAMHSA) layers that compute and fuse multiple self-attention maps representing multifaceted self-similarity. The estimated features are then clustered into an appropriate number of sections with a Gaussian mixture model (GMM). In the segmentation and clustering tasks, the proposed method outperformed baseline methods based on hand-crafted SSMs. In particular, it achieved state-of-the-art performance on the segmentation task. We found that the internal attention maps represent the section boundaries at the fine and course levels.
更多查看译文
AI 理解论文
溯源树
样例
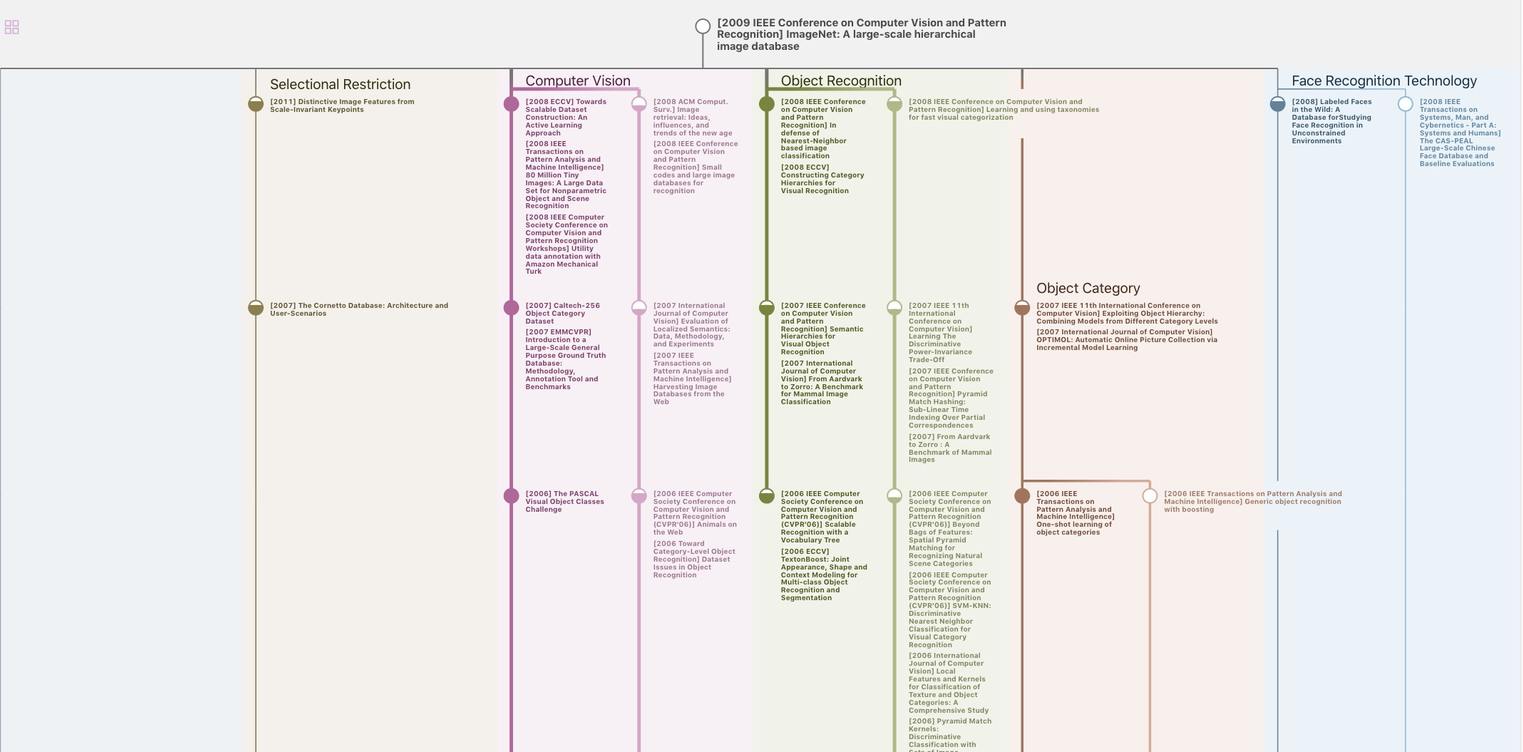
生成溯源树,研究论文发展脉络
Chat Paper
正在生成论文摘要