Language modeling for spontaneous speech recognition based on disfluency labeling and generation of disfluent text
2023 ASIA PACIFIC SIGNAL AND INFORMATION PROCESSING ASSOCIATION ANNUAL SUMMIT AND CONFERENCE, APSIPA ASC(2023)
摘要
Disfluencies in spontaneous speech such as fillers and hesitations are major causes of automatic speech recognition (ASR) errors. In our previous work, we proposed a "disfluency labeling" method allows an end-to-end (E2E) ASR model to recognize such disfluencies as symbolized recognition targets. This study proposes a method of further improving spontaneous speech recognition by integrating a language model (LM) that can predict disfluencies as pre-defined symbols. First, we fine-tuned a pre-trained Bidirectional Encoder Representations from Transformers (BERT) to predict word boundaries where disfluency symbols ("#" for a filler and "@" for a hesitation) should be inserted within a corpus of normal written text. We then used it to generated a large corpus of disfluent text. Finally, we trained an LM to predict the disfluency symbols with the BERT-generated corpus, and integrated the LM into an E2E ASR model using Shallow Fusion. Our experimental results show that the LM trained using the generated disfluent text corpus improved disfluency prediction and, as a result, spontaneous speech recognition performance.
更多查看译文
AI 理解论文
溯源树
样例
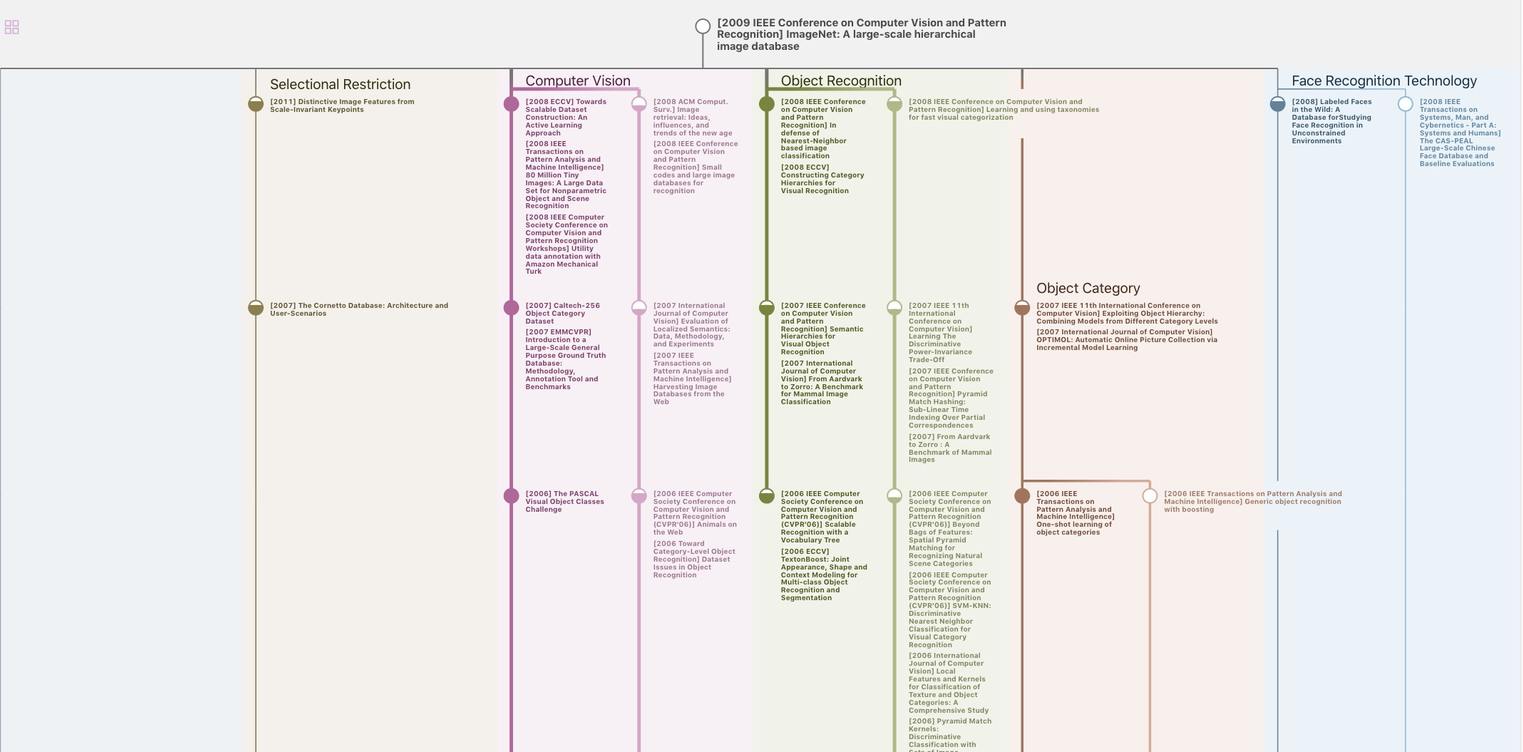
生成溯源树,研究论文发展脉络
Chat Paper
正在生成论文摘要