Learning Semantic Information from Machine Translation to Improve Speech-to-Text Translation
2023 ASIA PACIFIC SIGNAL AND INFORMATION PROCESSING ASSOCIATION ANNUAL SUMMIT AND CONFERENCE, APSIPA ASC(2023)
摘要
End-to-end speech translation (ST) directly translates the source speech to the target text, following a typical encoder-decoder framework. However, it has shown that the conventional ST encoder is mainly used to extract long but locally attentive acoustic features, which may lead to a lack of global semantic features. In this work, we therefore propose to integrate a semantic decoder into the speech translation (SD-ST) model, where the semantic decoder can generate text-like features with more global semantic information analogously to the machine translation system (MT). We also investigate different strategies to ensure length consistency between text-like features and text sequences. Experimental results show that the proposed SD-ST model achieves the best BLEU score on the 40-hour subset of the Fisher Spanish English dataset and a comparable BLEU score on the MuST-C dataset. Furthermore, it is shown that the SD-ST model can even perform zero-shot ST.
更多查看译文
关键词
End-to-end speech translation,semantic information,encoder-decoder,speech recognition
AI 理解论文
溯源树
样例
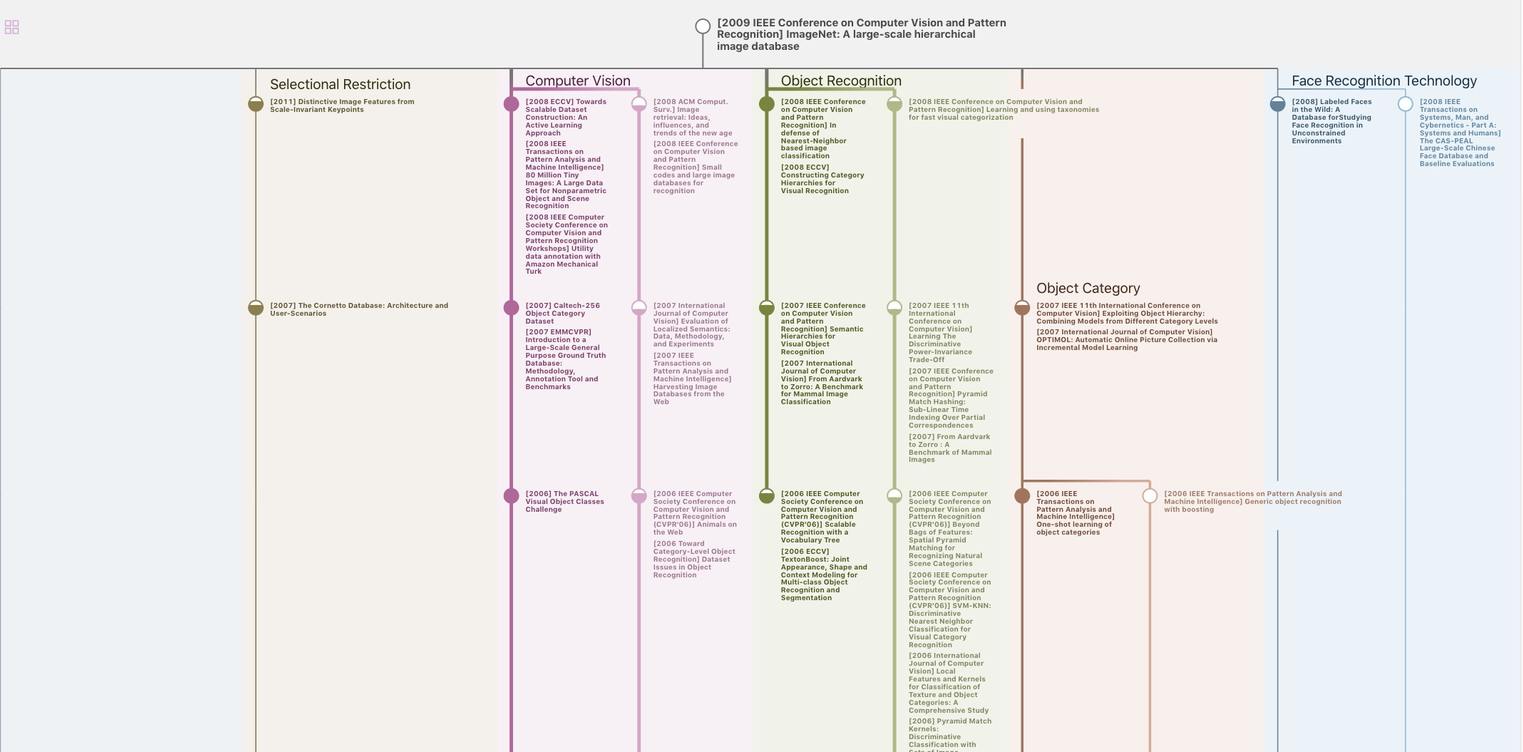
生成溯源树,研究论文发展脉络
Chat Paper
正在生成论文摘要