Zone-of-Interest Strategy for the Creation of High-Resolution Adversarial Images Against Convolutional Neural Networks
2023 15th International Conference on Information Technology and Electrical Engineering (ICITEE)(2023)
摘要
Trained convolutional neural networks (CNNs) are among the leading tools used for the automatic classification of images. They are nevertheless exposed to attacks: Given an input clean image classified by a CNN in a category, carefully designed adversarial images may lead CNNs to erroneous classifications, although humans would still classify “correctly” the constructed adversarial images in the same category as the input image. Currently most attacks are performed in the image input size domain of the considered CNN, which is usually small. However, due to privacy concerns with personal images on social media, there is a demand for generating large adversarial images that preserve the visual information of original images with the highest possible quality, while preventing automatic tracking and personal identification. Creating large-size adversarial images is difficult due to speed, adversity, and visual quality challenges, in particular if a requirement on adversarial images is the inability for humans to notice any difference between them and the original clean images. This paper describes the zone-of-interest generic strategy that aims at increasing drastically the efficiency of any type of attack (white-box or black-box, untargeted or targeted) and any specific attack (FGSM, PGD, BIM, SimBA, AdvGAN, EA-based attacks, etc.) on CNNs. Instead of exploring the full image size, the strategy identifies zones on which to focus the attacks. Although applying to any image size, the strategy is especially valuable for large high-resolution images. This strategy can be combined with other generic approaches, like the noise blowing-up method, to further improve attacks' performances.
更多查看译文
关键词
Black-box attack,Convolutional Neural Network,High resolution adversarial image,Noise Blowing-Up method
AI 理解论文
溯源树
样例
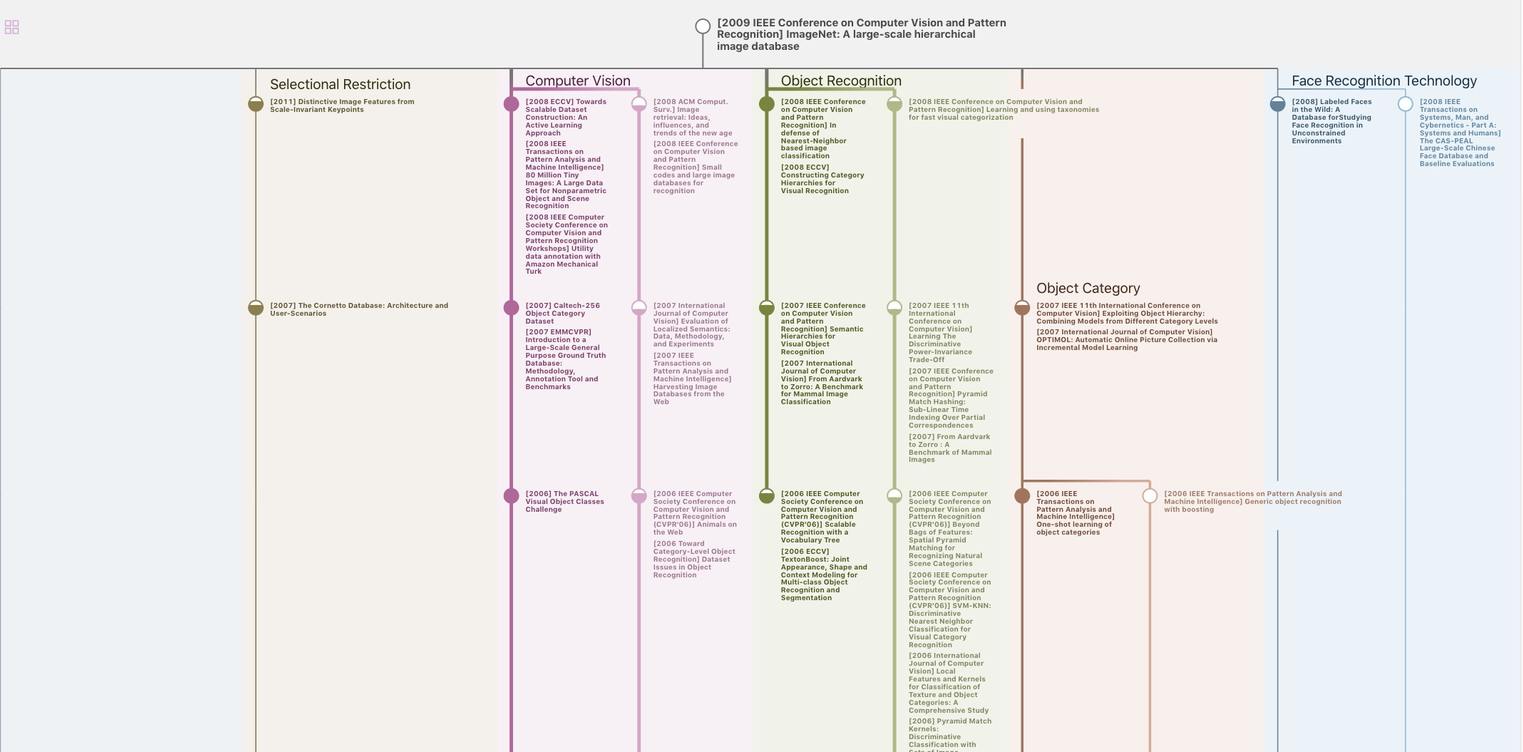
生成溯源树,研究论文发展脉络
Chat Paper
正在生成论文摘要