Adversarial Testing: A Novel On-line Testing Method for Deep Learning Processors
2023 IEEE 32ND ASIAN TEST SYMPOSIUM, ATS(2023)
摘要
Deep neural networks have shown outstanding performance on complex tasks. Recently, various researches have been developed to pursue fast and energy-efficient deep learning accelerators. However, devices may suffer from hard defects and hardware variability during its lifetime, which poses severe challenges to deep learning accelerators. To protect edge deep learning accelerators from fault-induced failures, we leverage the adversarial deep learning technique to tailor a lightweight online fault detection method for neural network accelerator chips. The proposed Adversarial Testing scheme (AT) is a function-level testing method outcompeting conventional test in several ways: negligible run-time overhead, super sensitivity to subtle hardware variations, which reduces chip over-kills and also the unnecessary diagnosis operations. The evaluation results show that AT can accurately detect fault occurrence and ensure the normal use of deep learning accelerator during its lifetime.
更多查看译文
AI 理解论文
溯源树
样例
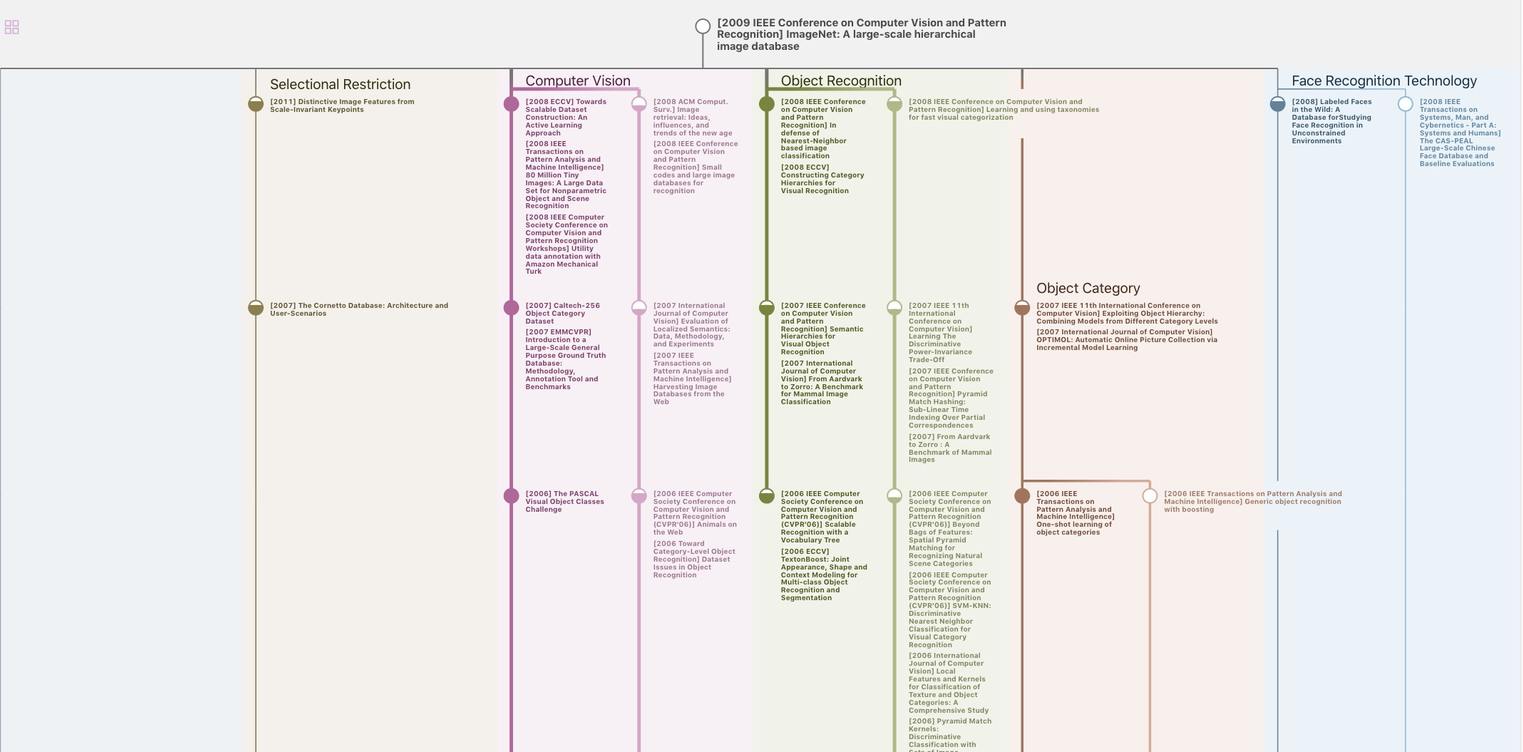
生成溯源树,研究论文发展脉络
Chat Paper
正在生成论文摘要