Deep-Learning-Based 3-D Surface Reconstruction-A Survey
PROCEEDINGS OF THE IEEE(2023)
Abstract
In the last decade, deep learning (DL) has significantly impacted industry and science. Initially largely motivated by computer vision tasks in 2-D imagery, the focus has shifted toward 3-D data analysis. In particular, 3-D surface reconstruction, i.e., reconstructing a 3-D shape from sparse input, is of great interest to a large variety of application fields. DL-based approaches show promising quantitative and qualitative surface reconstruction performance compared to traditional computer vision and geometric algorithms. This survey provides a comprehensive overview of these DL-based methods for 3-D surface reconstruction. To this end, we will first discuss input data modalities, such as volumetric data, point clouds, and RGB, single-view, multiview, and depth images, along with corresponding acquisition technologies and common benchmark datasets. For practical purposes, we also discuss evaluation metrics enabling us to judge the reconstructive performance of different methods. The main part of the document will introduce a methodological taxonomy ranging from point-and mesh-based techniques to volumetric and implicit neural approaches. Recent research trends, both methodological and for applications, are highlighted, pointing toward future developments.
MoreTranslated text
Key words
3-D deep learning (DL),3-D surface reconstruction,geometric DL,geometry processing,machine learning
AI Read Science
Must-Reading Tree
Example
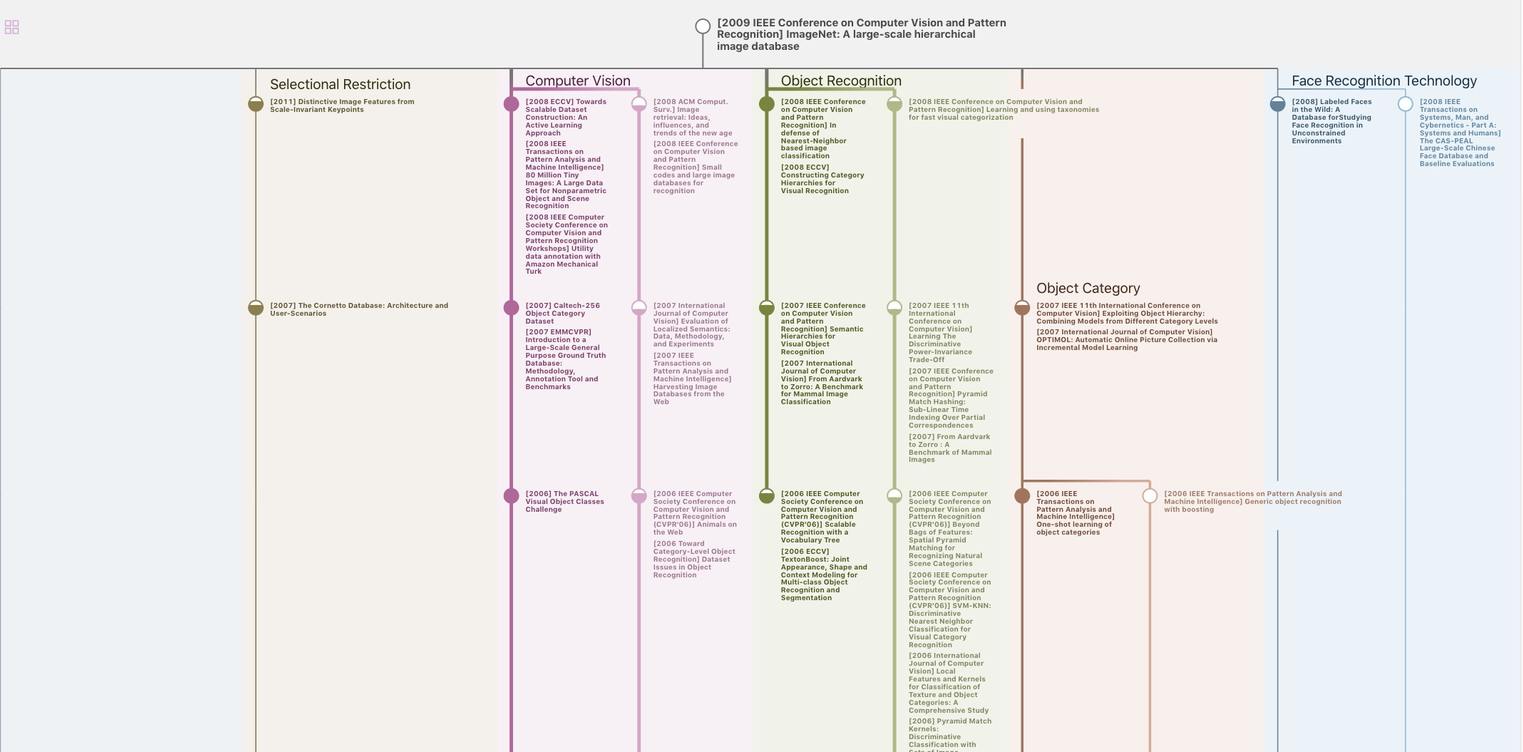
Generate MRT to find the research sequence of this paper
Chat Paper
Summary is being generated by the instructions you defined