Adaptive parametric change point inference under covariance structure changes
Statistical Papers(2023)
摘要
The article offers a method for estimating the volatility covariance matrix of vectors of financial time series data using a change point approach. The proposed method supersedes general varying-coefficient parametric models, such as GARCH, whose coefficients may vary with time, by a change point model. In this study, an adaptive pointwise selection of homogeneous segments with a given right-end point by a local change point analysis is introduced. Sufficient conditions are obtained under which the maximum likelihood process is adaptive against the covariance estimate to yield an optimal rate of convergence with respect to the change size. This rate is preserved while allowing the jump size to diminish. Under these circumstances, argmax results of a two-sided negative Brownian motion or a two-sided negative drift random walk under vanishing and non-vanishing jump size regimes, respectively, provide inference for the change point parameter. Theoretical results are supported by the Monte–Carlo simulation study. A bivariate data on daily log returns of two US stock market indices as well as tri-variate data on daily log returns of three banks are analyzed by constructing confidence interval estimates for multiple change points that have been identified previously for each of the two data sets.
更多查看译文
关键词
Brownian motion,Change point,Covariance structure,Maximum likelihood estimator,Random walk
AI 理解论文
溯源树
样例
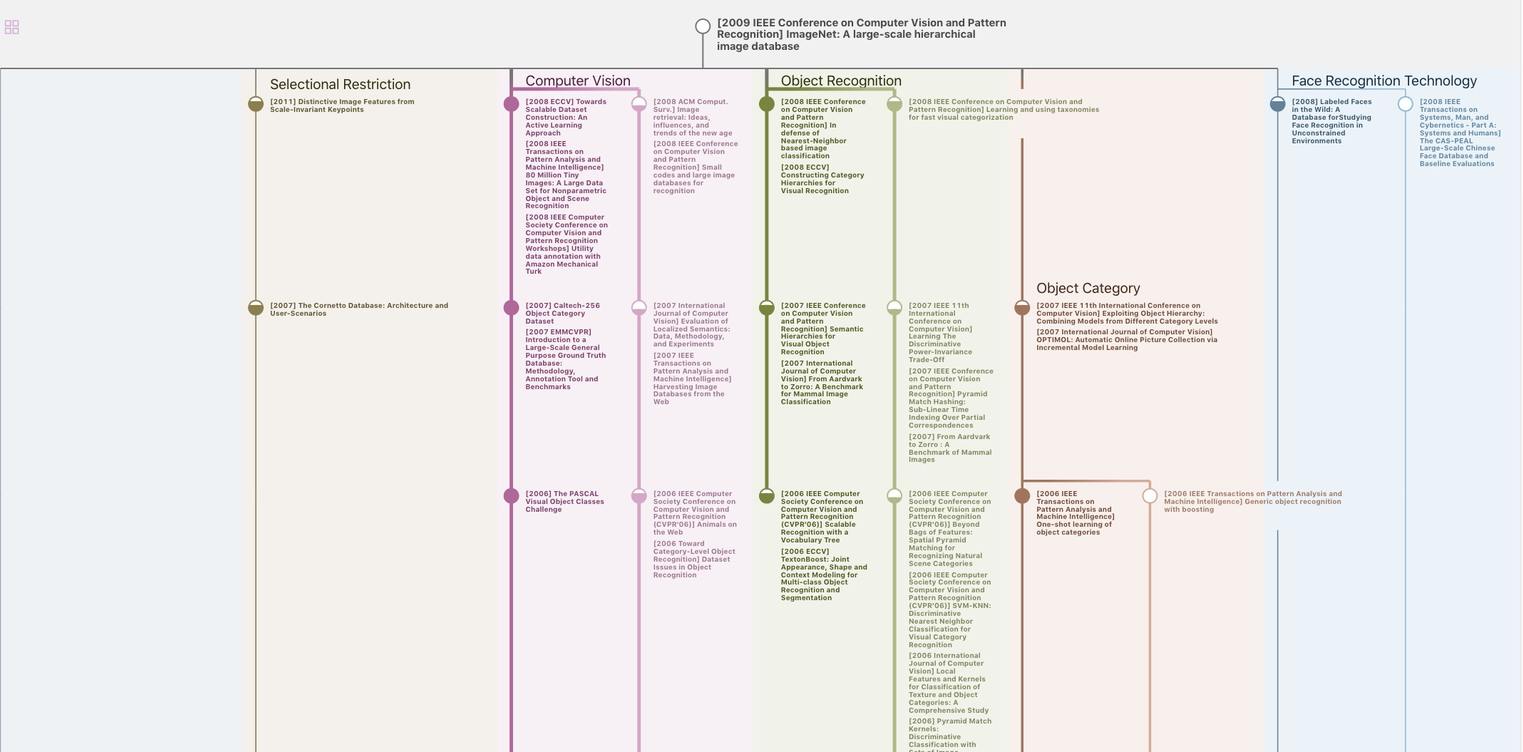
生成溯源树,研究论文发展脉络
Chat Paper
正在生成论文摘要