Correction to: Improving rainfall forecast at the district scale over the eastern Indian region using deep neural network
Theoretical and Applied Climatology(2024)
摘要
Indian Summer Monsoon (ISM) rainfall is largely contributed by synoptic scale low-pressure systems over the Bay of Bengal and moves towards Indian landmass through eastern Indian states such as Odisha. These systems often cause heavy to very heavy rainfall localized events. The prediction of these events with high accuracy is still a major challenge for deterministic weather models. For the first time, this study has used machine learning (ML) and deep learning (DL) methods to improve the rainfall forecast using Weather Research and Forecast (WRF) forecasts output up to a lead time of 96 h (day 4) at the district scale of Odisha. Our findings demonstrate that the ML model improves the cumulative rainfall forecast (> 70%) but not more than the DL (multilayer perceptron (MLP) and convolutional neural network (CNN)) models, i.e., > 80%. Overall, on average, the DL model improved the rainfall prediction accuracy by 14% compared to ML models and 16% compared to the WRF model respectively. Results suggested that the CNN predicts rainfall with more than 70% for heavy and very heavy rainfall events for all days. It is also noted that WRF microphysics schemes are biased towards light rainfall class and the same has been effectively corrected by DL models. Furthermore, CNN shows promising results with more than 80% percent accuracy in forecasting rainfall for heavy rainfall events at the district scale. The inclusion of DL models in the Numerical Weather Prediction (NWP) model forecast output convincingly enhances the prediction skills. The findings of this study are highly significant for operational agencies, and disaster managers for effective planning, management, and preparedness at the district scale.
更多查看译文
AI 理解论文
溯源树
样例
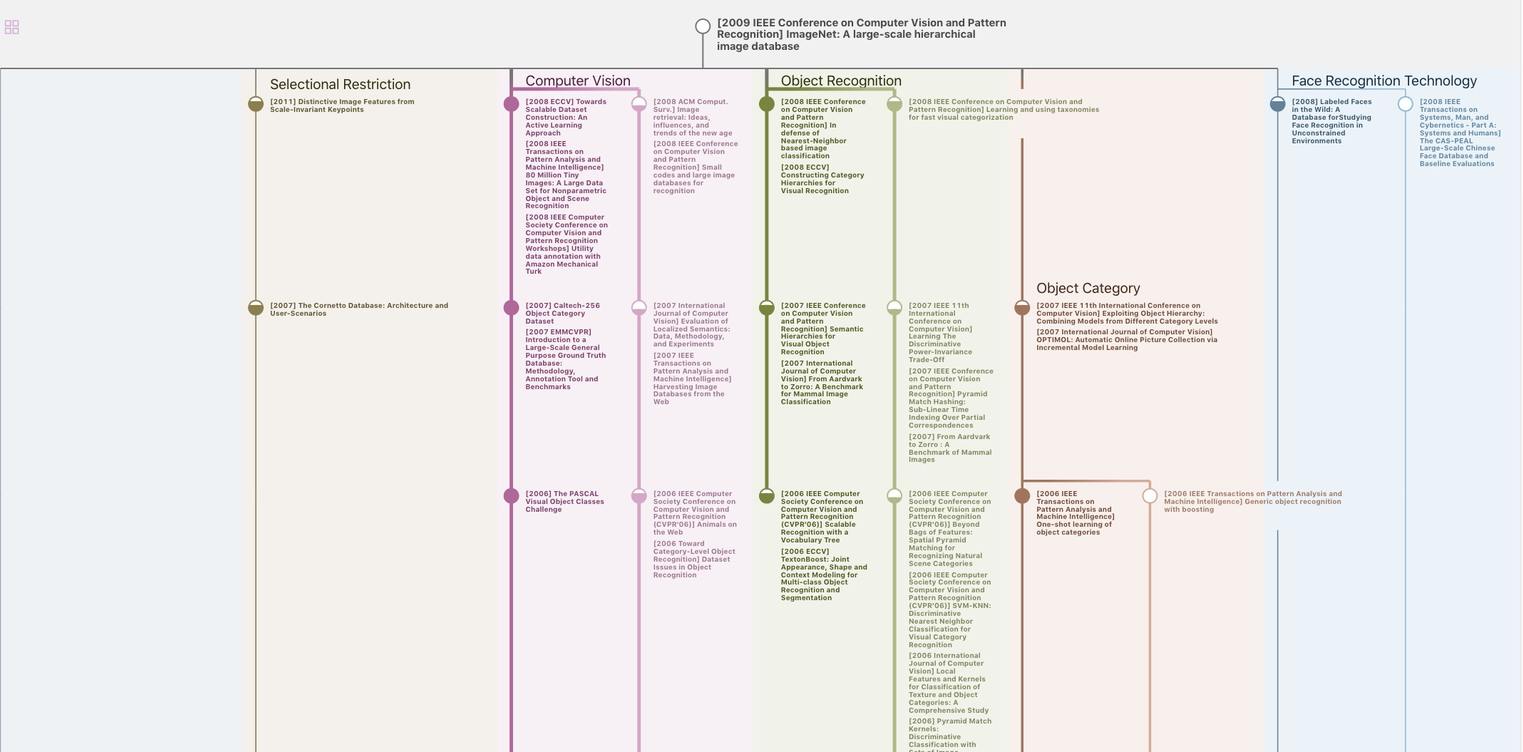
生成溯源树,研究论文发展脉络
Chat Paper
正在生成论文摘要