DRL-dEWMA: a composite framework for run-to-run control in the semiconductor manufacturing process
Neural Computing and Applications(2024)
Abstract
This study aims to develop a weight-adjustment scheme for a double exponentially weighted moving average (dEWMA) controller using deep reinforcement learning (DRL) techniques. Under the run-to-run control framework, the weight adjustment of the dEWMA is formulated as a Markovian decision process in which the candidate weights are viewed as the DRL agent’s decision action. Accordingly, a composite control strategy integrating DRL and dEWMA is proposed. Specifically, a well-trained DRL agent serves as an auxiliary controller that produces the preferred weights of the dEWMA. The optimized dEWMA serves as a master controller to provide a suitable recipe for the manufacturing process. Furthermore, two classical deterministic policy-gradient algorithms are leveraged for automatic weight tuning. The simulation results show that the proposed scheme outperforms existing RtR controllers in terms of disturbance rejection and target tracking. The proposed scheme has significant practical application prospects in smart semiconductor manufacturing.
MoreTranslated text
Key words
Deep reinforcement learning,Semiconductor manufacturing,Run-to-run control,Double exponentially weighted moving average,Parameter optimization
AI Read Science
Must-Reading Tree
Example
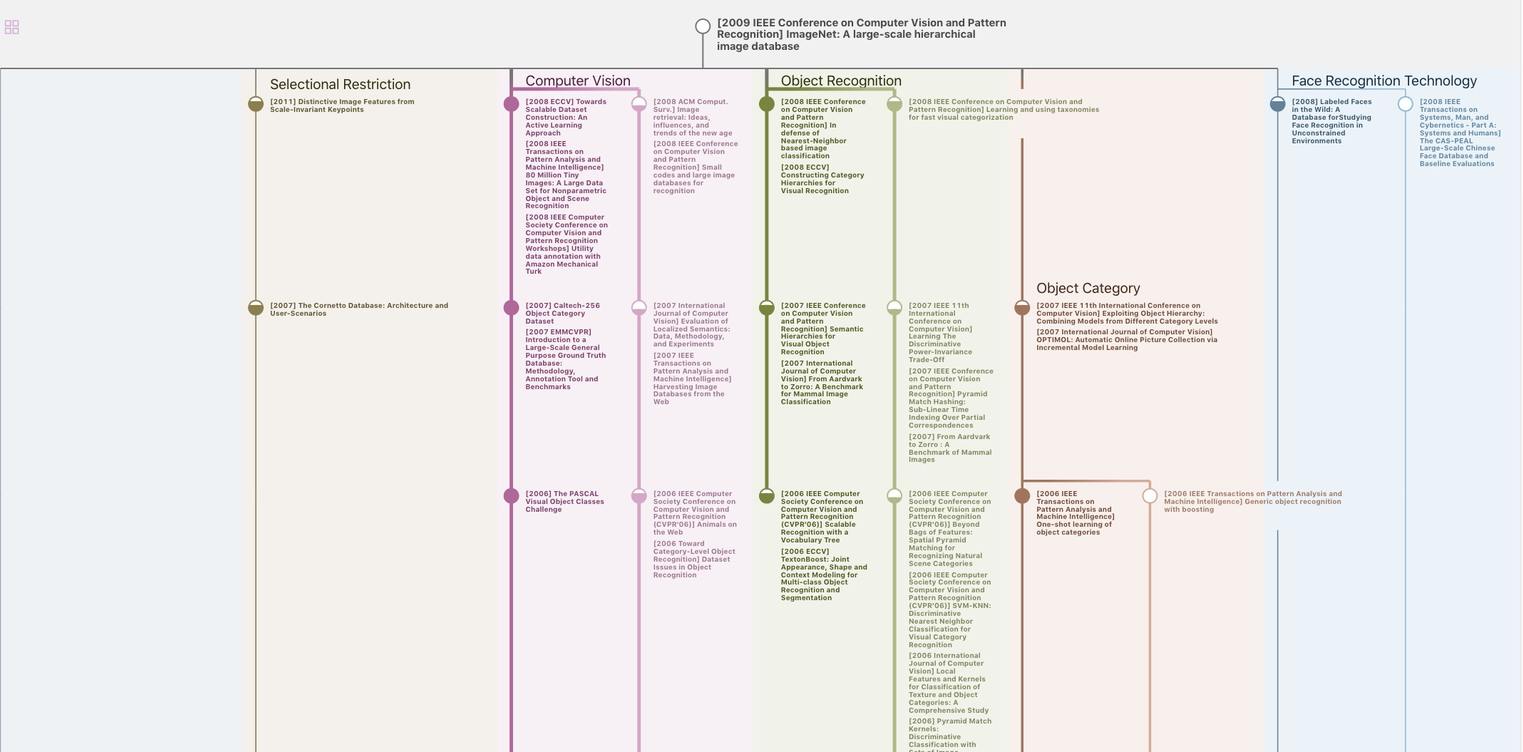
Generate MRT to find the research sequence of this paper
Chat Paper
Summary is being generated by the instructions you defined