Early diagnosis of Parkinson’s disease
Computational Biology and Chemistry(2023)
摘要
Predicting Unified Parkinson's Disease Rating Scale (UPDRS) in Total- UPDRS and Motor-UPDRS clinical scales is an important part of controlling PD. Computational intelligence approaches have been used effectively in the early diagnosis of PD by predicting UPDRS. In this research, we target to present a combined approach for PD diagnosis using an ensemble learning approach with the ability of online learning from clinical large datasets. The method is developed using Deep Belief Network (DBN) and Neuro-Fuzzy approaches. A clustering approach, Expectation-Maximization (EM), is used to handle large datasets. The Principle Component Analysis (PCA) technique is employed for noise removal from the data. The UPDRS prediction models are constructed for PD diagnosis. To handle the missing data, K-NN is used in the proposed method. We use incremental machine learning approaches to improve the efficiency of the proposed method. We assess our approach on a real-world PD dataset and the findings are assessed compared to other PD diagnosis approaches developed by machine learning techniques. The findings revealed that the approach can improve the UPDRS prediction accuracy and the time complexity of previous methods in handling large datasets. Display Omitted • A new method using EM, DBN and ANFIS is developed for PD diagnosis. • Accuracy and computation time are improved by the proposed method. • UPDRS is predicted through the input variables in the dataset. • The results showed that the method is efficient for PD diagnosis.
更多查看译文
关键词
Computational intelligence,Parkinson’s disease,UPDRS,Diagnosis,Accuracy,Time complexity
AI 理解论文
溯源树
样例
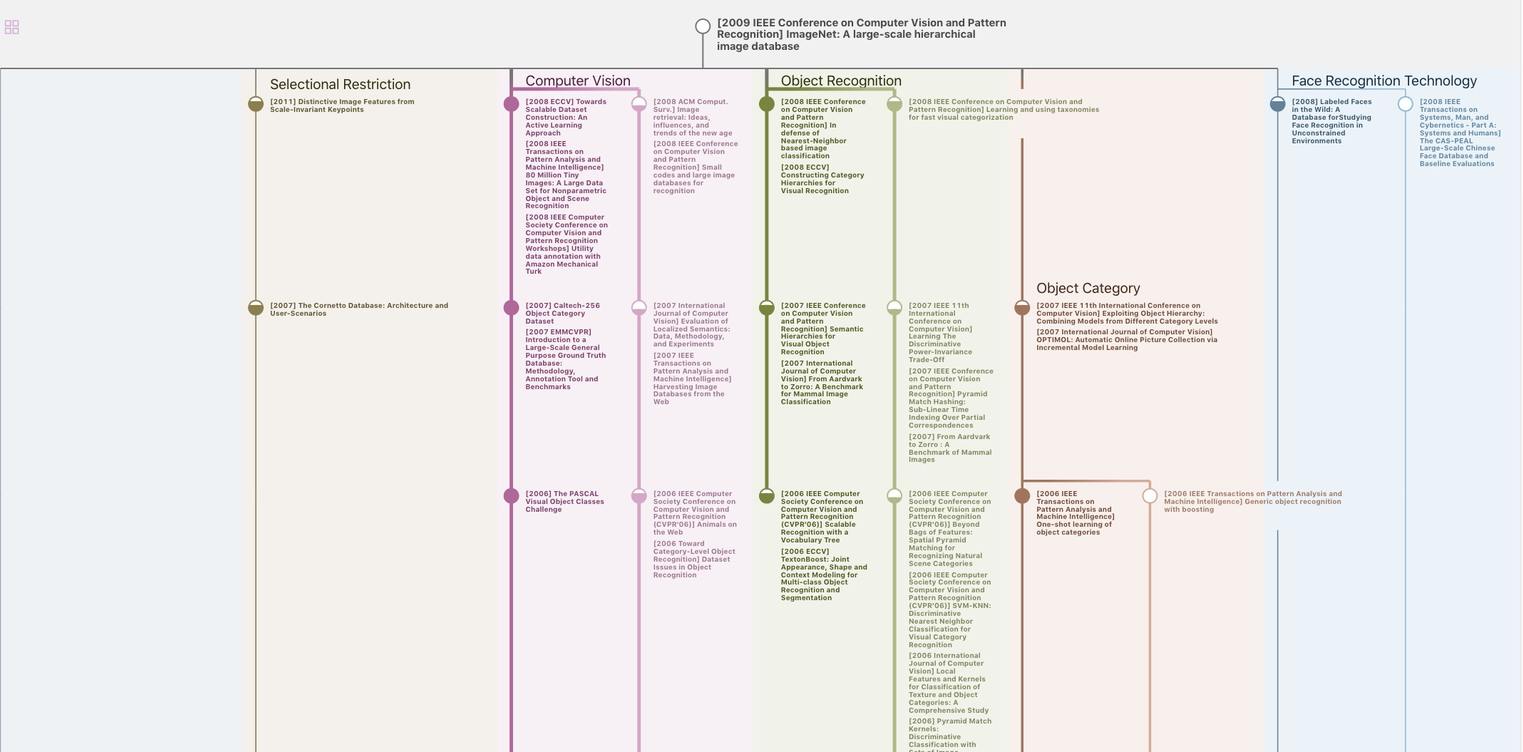
生成溯源树,研究论文发展脉络
Chat Paper
正在生成论文摘要