Binary Discriminator Facilitates GPT-based Protein Design
biorxiv(2023)
摘要
Generative pre-trained transformers (GPT) models provide powerful platforms for pioneering de novo protein design (DNPD). GPT-based DNPD involves three procedures: a) finetuning the model with proteins of interest; b) generating sequence candidates with the finetuned model; and c) prioritizing the sequence candidates. Existing prioritization strategies heavily rely on sequence identity, undermining the diversity. Here, we coupled a protein GPT model with a custom discriminator, which enables selecting candidates of low identity to natural sequences while highly likely with desired functions. We applied this framework to creating novel antimicrobial peptides (AMPs) and malate dehydrogenases (MDHs). Experimental verification pinpointed four broad-spectrum AMPs from 24 candidates, and comprehensive computational analyses on the prioritized MDHs candidates provide compelling evidence for the anticipated function. This framework is time- and data-efficient and may therefore considerably expedite the DNPD process.
### Competing Interest Statement
X.L. has a financial interest in Demetrix and Synceres.
更多查看译文
AI 理解论文
溯源树
样例
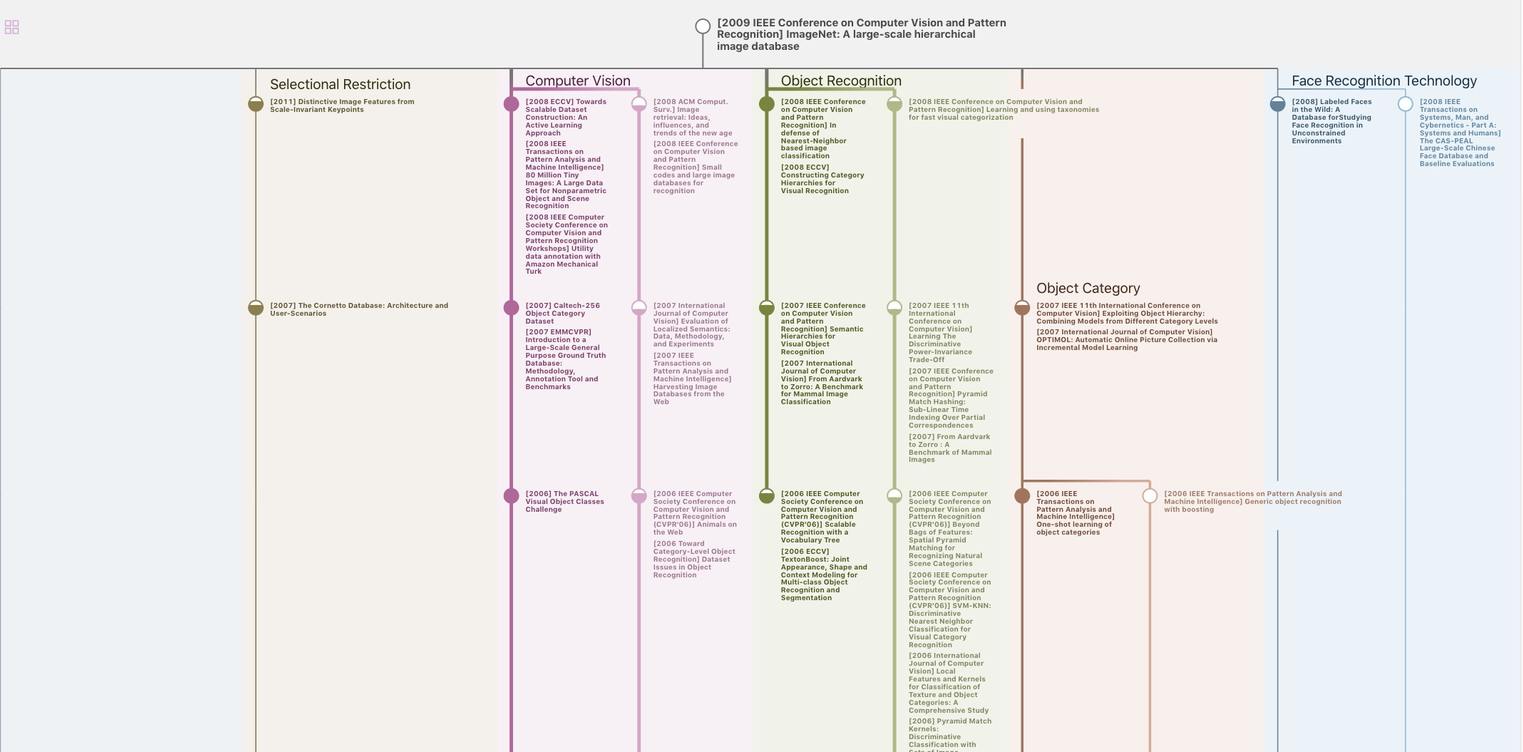
生成溯源树,研究论文发展脉络
Chat Paper
正在生成论文摘要