Optimal Locally Private Nonparametric Classification with Public Data.
CoRR(2023)
摘要
In this work, we investigate the problem of public data-assisted
non-interactive LDP (Local Differential Privacy) learning with a focus on
non-parametric classification. Under the posterior drift assumption, we for the
first time derive the mini-max optimal convergence rate with LDP constraint.
Then, we present a novel approach, the locally private classification tree,
which attains the mini-max optimal convergence rate. Furthermore, we design a
data-driven pruning procedure that avoids parameter tuning and produces a fast
converging estimator. Comprehensive experiments conducted on synthetic and real
datasets show the superior performance of our proposed method. Both our
theoretical and experimental findings demonstrate the effectiveness of public
data compared to private data, which leads to practical suggestions for
prioritizing non-private data collection.
更多查看译文
AI 理解论文
溯源树
样例
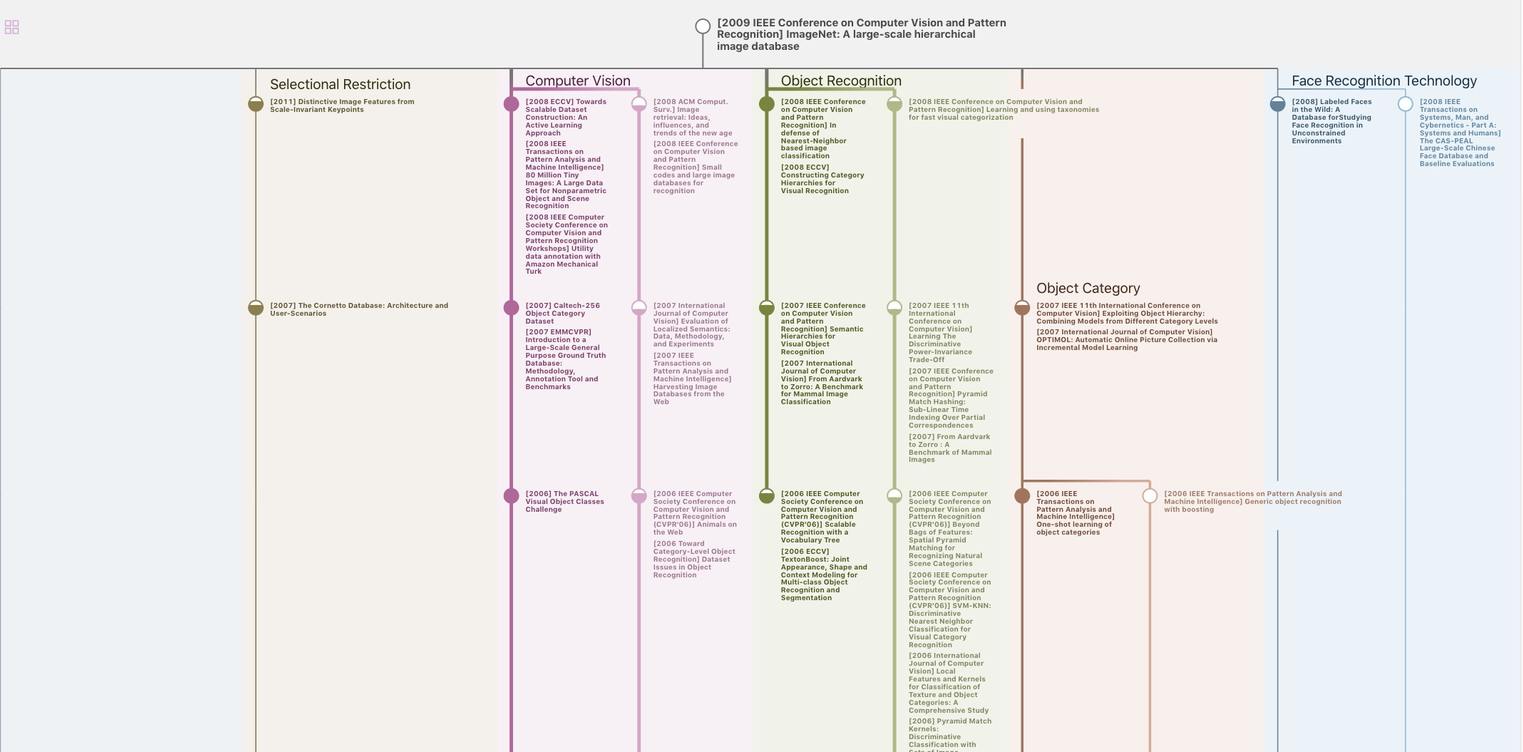
生成溯源树,研究论文发展脉络
Chat Paper
正在生成论文摘要