Labeling Indoor Scenes with Fusion of Out-of-the-Box Perception Models
2024 IEEE/CVF Winter Conference on Applications of Computer Vision Workshops (WACVW)(2023)
摘要
The image annotation stage is a critical and often the most time-consuming
part required for training and evaluating object detection and semantic
segmentation models. Deployment of the existing models in novel environments
often requires detecting novel semantic classes not present in the training
data. Furthermore, indoor scenes contain significant viewpoint variations,
which need to be handled properly by trained perception models. We propose to
leverage the recent advancements in state-of-the-art models for bottom-up
segmentation (SAM), object detection (Detic), and semantic segmentation
(MaskFormer), all trained on large-scale datasets. We aim to develop a
cost-effective labeling approach to obtain pseudo-labels for semantic
segmentation and object instance detection in indoor environments, with the
ultimate goal of facilitating the training of lightweight models for various
downstream tasks. We also propose a multi-view labeling fusion stage, which
considers the setting where multiple views of the scenes are available and can
be used to identify and rectify single-view inconsistencies. We demonstrate the
effectiveness of the proposed approach on the Active Vision dataset and the
ADE20K dataset. We evaluate the quality of our labeling process by comparing it
with human annotations. Also, we demonstrate the effectiveness of the obtained
labels in downstream tasks such as object goal navigation and part discovery.
In the context of object goal navigation, we depict enhanced performance using
this fusion approach compared to a zero-shot baseline that utilizes large
monolithic vision-language pre-trained models.
更多查看译文
关键词
Object Detection,Segmentation Model,Indoor Environments,Semantic Segmentation,Object Parts,Object Instances,Object Detection Model,Semantic Segmentation Models,Point Cloud,ImageNet,Bounding Box,Latent Space,Small Objects,Target Object,Depth Map,Depth Images,Segmentation Results,Domain Adaptation,Instance Segmentation,Semantic Labels,Semantic Map,Camera Pose,Semantic Annotation,Navigation Task,Label Propagation,Vision Transformer,Background Class,Dense Grid,Dot Product
AI 理解论文
溯源树
样例
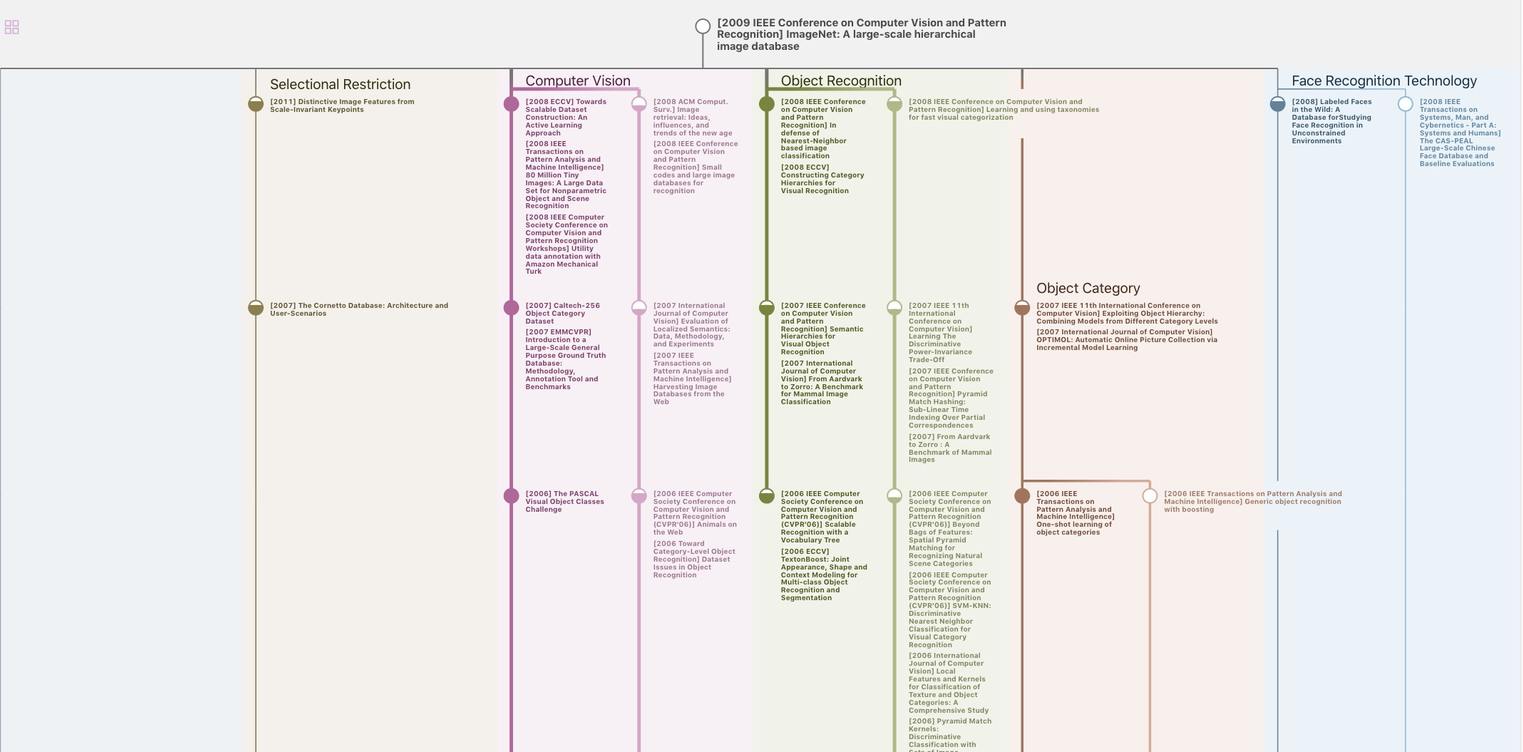
生成溯源树,研究论文发展脉络
Chat Paper
正在生成论文摘要