Evaluating perceptual and semantic interpretability of saliency methods
Applied AI Letters(2022)
摘要
In order to be useful, XAI explanations have to be faithful to the AI system they seek to elucidate and also interpretable to the people that engage with them. There exist multiple algorithmic methods for assessing faithfulness, but this is not so for interpretability, which is typically only assessed through expensive user studies. Here we propose two complementary metrics to algorithmically evaluate the interpretability of saliency map explanations. One metric assesses perceptual interpretability by quantifying the visual coherence of the saliency map. The second metric assesses semantic interpretability by capturing the degree of overlap between the saliency map and textbook features—features human experts use to make a classification. We use a melanoma dataset and a deep‐neural network classifier as a case‐study to explore how our two interpretability metrics relate to each other and a faithfulness metric. Across six commonly used saliency methods, we find that none achieves high scores across all three metrics for all test images, but that different methods perform well in different regions of the data distribution. This variation between methods can be leveraged to consistently achieve high interpretability and faithfulness by using our metrics to inform saliency mask selection on a case‐by‐case basis. Our interpretability metrics provide a new way to evaluate saliency‐based explanations and allow for the adaptive combination of saliency‐based explanation methods. XAI explanations have to be faithful to the AI system and also interpretable to people. We propose two complementary metrics—perceptual and semantic interpretability—to algorithmically evaluate the interpretability of saliency map explanations. Across six commonly used saliency methods, we find that none achieves high scores across the faithfulness and interpretability metrics, but that different methods perform well in different regions of the data distribution.
更多查看译文
关键词
explainable AI,interpretability,melanoma,textbook features
AI 理解论文
溯源树
样例
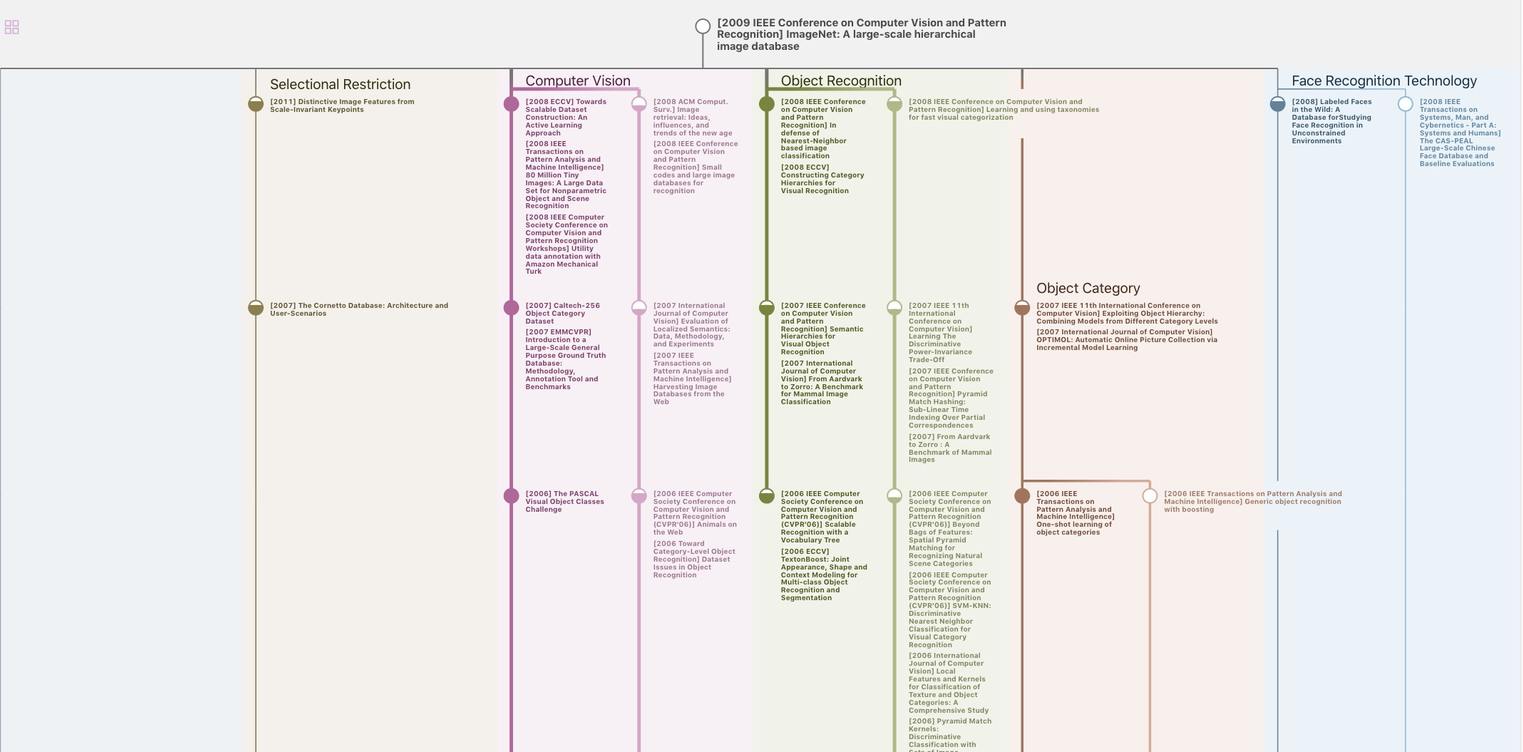
生成溯源树,研究论文发展脉络
Chat Paper
正在生成论文摘要