Deep4Ener: Energy Demand forecasting for Unseen Consumers with Scarce Data Using a Single Deep Learning Model
ACM SIGEnergy Energy Informatics Review(2023)
摘要
Forecasting the energy demand of individual consumers is a vital component of future smart energy grids since it enables energy-saving mechanisms such as Demand Response, activity scheduling, and prosumer energy markets. However, training a separate model with each consumer's available smart meter data can raise significant cold-start and scalability issues, despite the fact that personalization can be achieved in cases where the respective training sets have adequate data. Namely, making accurate forecasts for new consumers with limited historical data is challenging since a machine learning model requires a significant volume of data to be trained adequately, while scalability becomes an issue when the number of consumers increases. Training a single model on multiple consumers can mitigate these issues, hence we propose a single-model RNN-based deep learning architecture named Deep4Ener, for consumer-level energy demand forecasting, trained on multiple users and capable of making predictions for unseen consumers with scarce historical data that were not included in the training phase. Deep4Ener learns common energy demand characteristics among different consumers, by utilizing a novel architecture for energy profiling, including clustering, and an encoder neural network for feature extraction. Experiments with data from two open datasets show that Deep4Ener achieves high predictive performance both for known and completely new consumers, while outperforming the current state-of-the-art, namely one-model-per-consumer, standalone RNN, and Amazon's DeepAR approaches. Finally, we demonstrate that Deep4Ener shines when combined with Transfer Learning to further improve its forecasting performance on different energy demand consumers with limited data available.
更多查看译文
关键词
energy demand forecasting,deep4ener learning,unseen consumers
AI 理解论文
溯源树
样例
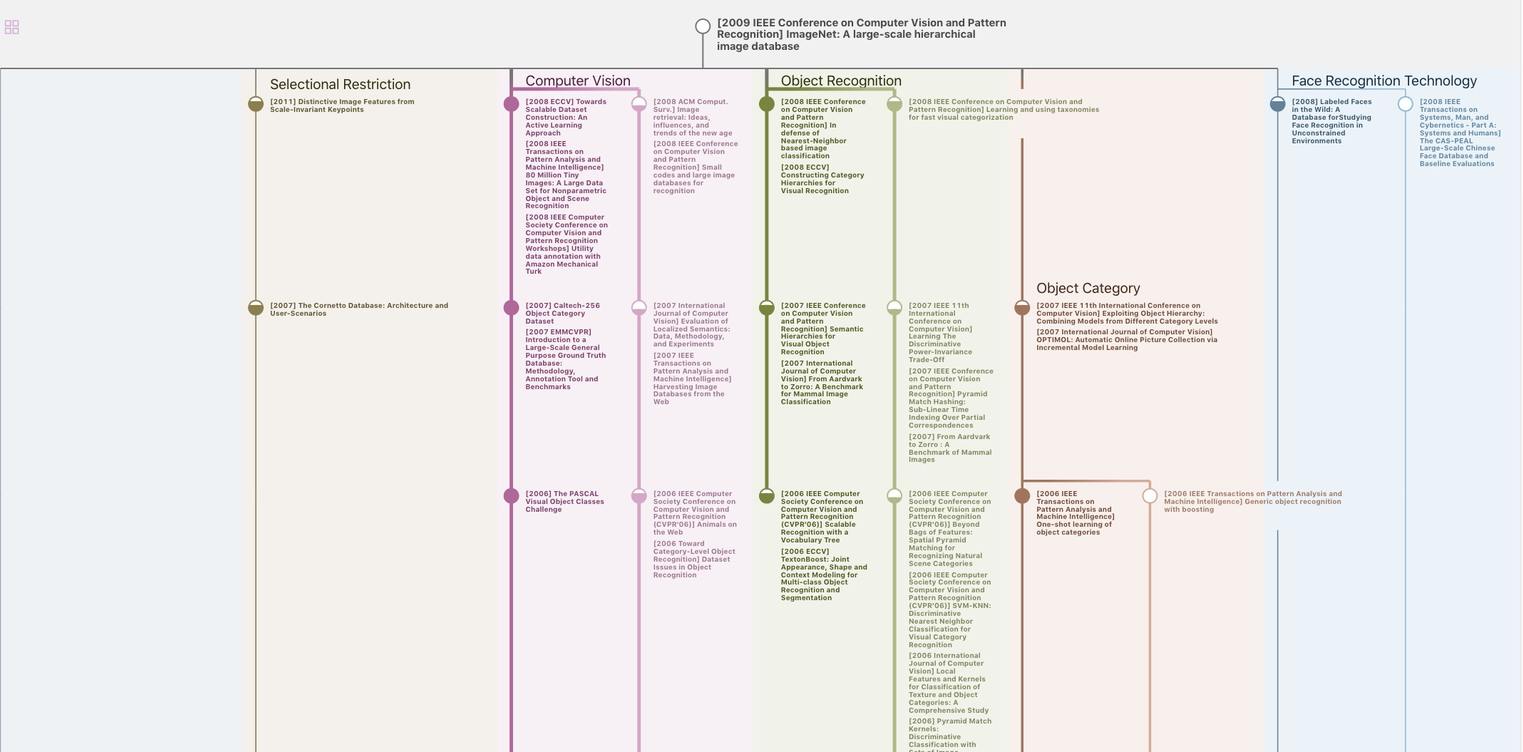
生成溯源树,研究论文发展脉络
Chat Paper
正在生成论文摘要