A multi-objective firefly algorithm combining logistic mapping and cross-variation
International Journal of Computing Science and Mathematics(2023)
Abstract
In the process of evolution, the multi-objective firefly algorithm (MOFA) has low optimisation accuracy and is prone to premature convergence, resulting in poor distribution and convergence of the population. To solve this problem, a multi-objective firefly algorithm (MOFA-LC) combining logistic mapping and cross-mutation was proposed. To improve the distribution of the population, the initial population with good ergodicity and uniformity was generated by logistic mapping. To improve population convergence, Levy flights and non-dominated sorting are used to improve the position updating formula. After the individual position updating, the cross-mutation method in the genetic algorithm can be used to improve the optimisation accuracy of the algorithm and make it jump out of the local optimal, overcome the intelligent convergence of the algorithm, and maintain the convergence of the population. In the experimental part, two typical test functions are selected to plot the IGD convergence curves of MOFA-LC and 11 recent multi-objective optimisation algorithms. The results show that MOFA-LC has obvious advantages over other algorithms.
MoreTranslated text
Key words
logistic mapping,algorithm,multi-objective,cross-variation
AI Read Science
Must-Reading Tree
Example
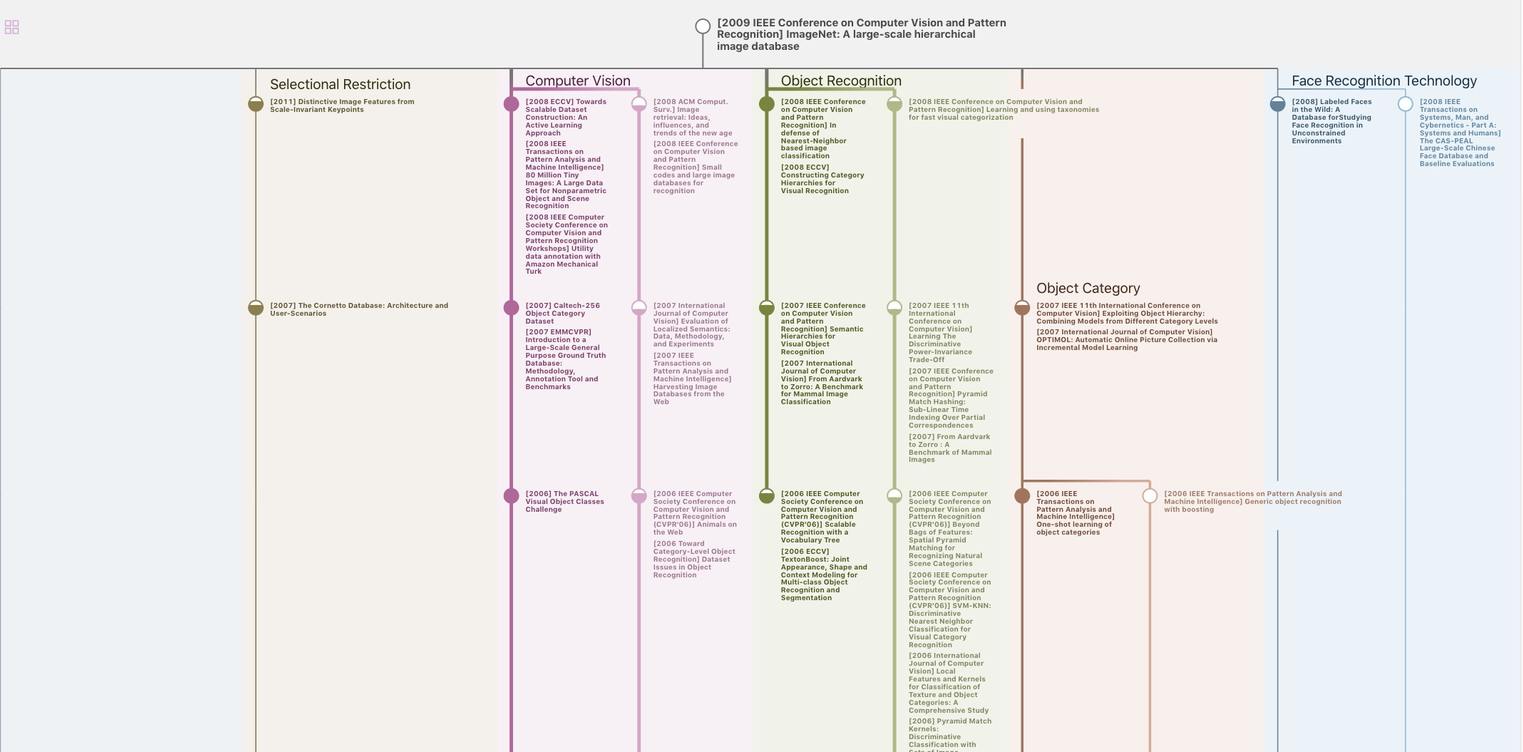
Generate MRT to find the research sequence of this paper
Chat Paper
Summary is being generated by the instructions you defined