Galaxy stellar and total mass estimation using machine learning
MONTHLY NOTICES OF THE ROYAL ASTRONOMICAL SOCIETY(2024)
摘要
Conventional galaxy mass estimation methods suffer from model assumptions and degeneracies. Machine learning (ML), which reduces the reliance on such assumptions, can be used to determine how well present-day observations can yield predictions for the distributions of stellar and dark matter. In this work, we use a general sample of galaxies from the TNG100 simulation to investigate the ability of multibranch convolutional neural network (CNN) based ML methods to predict the central (i.e. within 1-2 effective radii) stellar and total masses, and the stellar mass-to-light ratio (M-*/L). These models take galaxy images and spatially resolved mean velocity and velocity dispersion maps as inputs. Such CNN-based models can, in general, break the degeneracy between baryonic and dark matter in the sense that the model can make reliable predictions on the individual contributions of each component. For example, with r-band images and two galaxy kinematic maps as inputs, our model predicting M-*/L has a prediction uncertainty of 0.04 dex. Moreover, to investigate which (global) features significantly contribute to the correct predictions of the properties above, we utilize a gradient-boosting machine. We find that galaxy luminosity dominates the prediction of all masses in the central regions, with stellar velocity dispersion coming next. We also investigate the main contributing features when predicting stellar and dark matter mass fractions (f(*), f(DM)) and the dark matter mass M-DM, and discuss the underlying astrophysics.
更多查看译文
关键词
methods: data analysis,galaxies: kinematics and dynamics
AI 理解论文
溯源树
样例
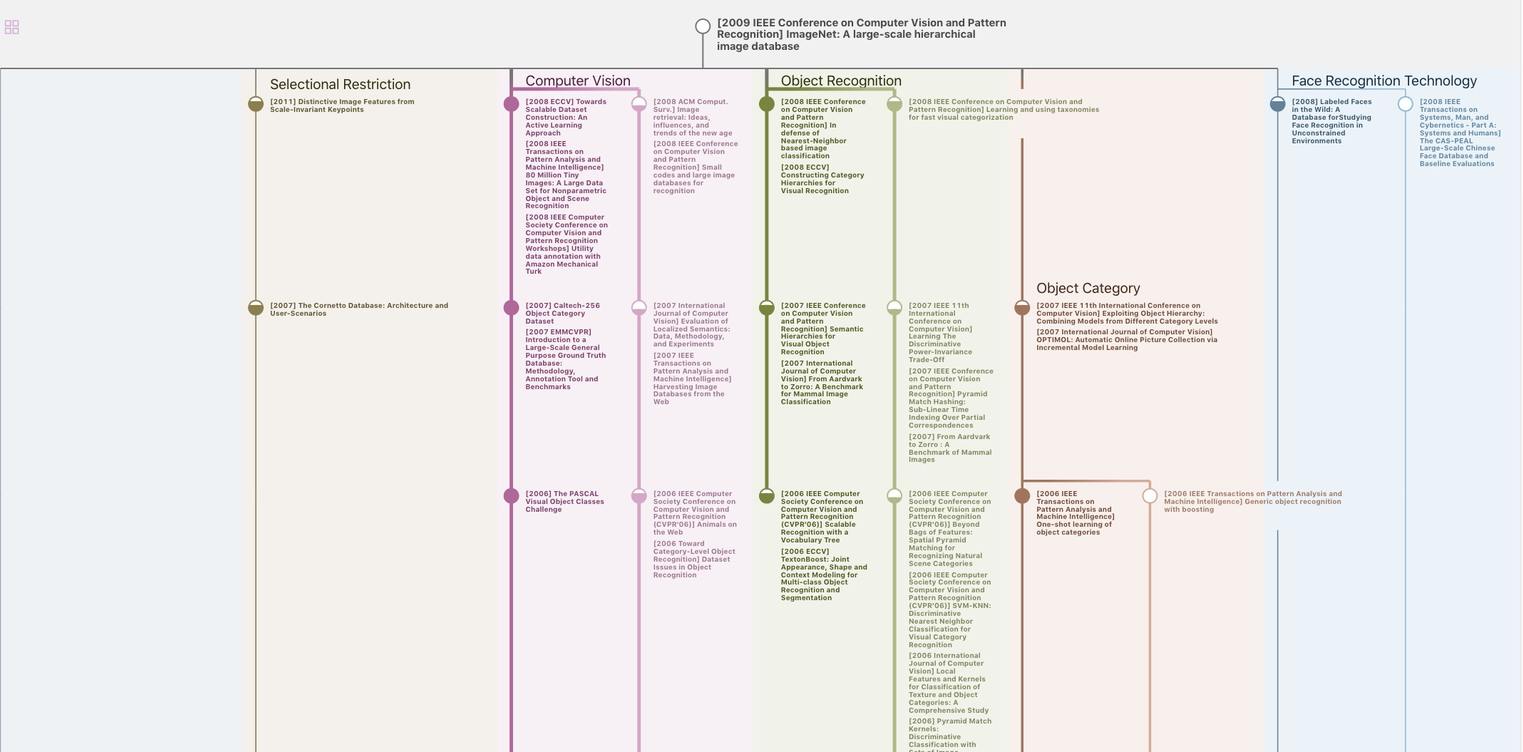
生成溯源树,研究论文发展脉络
Chat Paper
正在生成论文摘要