Chemotaxis of an elastic flagellated microrobot
PHYSICAL REVIEW E(2023)
摘要
Machine learning algorithms offer a tool to boost mobility and flexibility of a synthetic microswimmer, hence may help us design truly smart microrobots. In this work, we design a two-gait microrobot swimming in circular or helical trajectory. It utilizes the coupling between flagellum elasticity and resistive force to change the characteristics of swimming trajectory. Leveraging a deep reinforcement learning (DRL) approach, we show that the microrobot can self-learn chemotactic motion autonomously (without heuristics) using only several current and historical chemoattractant concentration and curvature information. The learned strategy is more efficient than a human-devised shortsighted strategy and can be further greatly improved in a stochastic environment. Furthermore, in the helical trajectory case, if additional heuristic information of direction is supplemented to evaluate the strategy during the learning process, then a highly efficient strategy can be discovered by the DRL. The microrobot can quickly align the helix vector to the gradient direction using just several smart sequential gait switchings. The success for the efficient strategies depends on how much historical information is provided and also the steering angle step size of the microrobot. Our results provide useful guidance for the design and smart maneuver of synthetic spermlike microswimmers.
更多查看译文
AI 理解论文
溯源树
样例
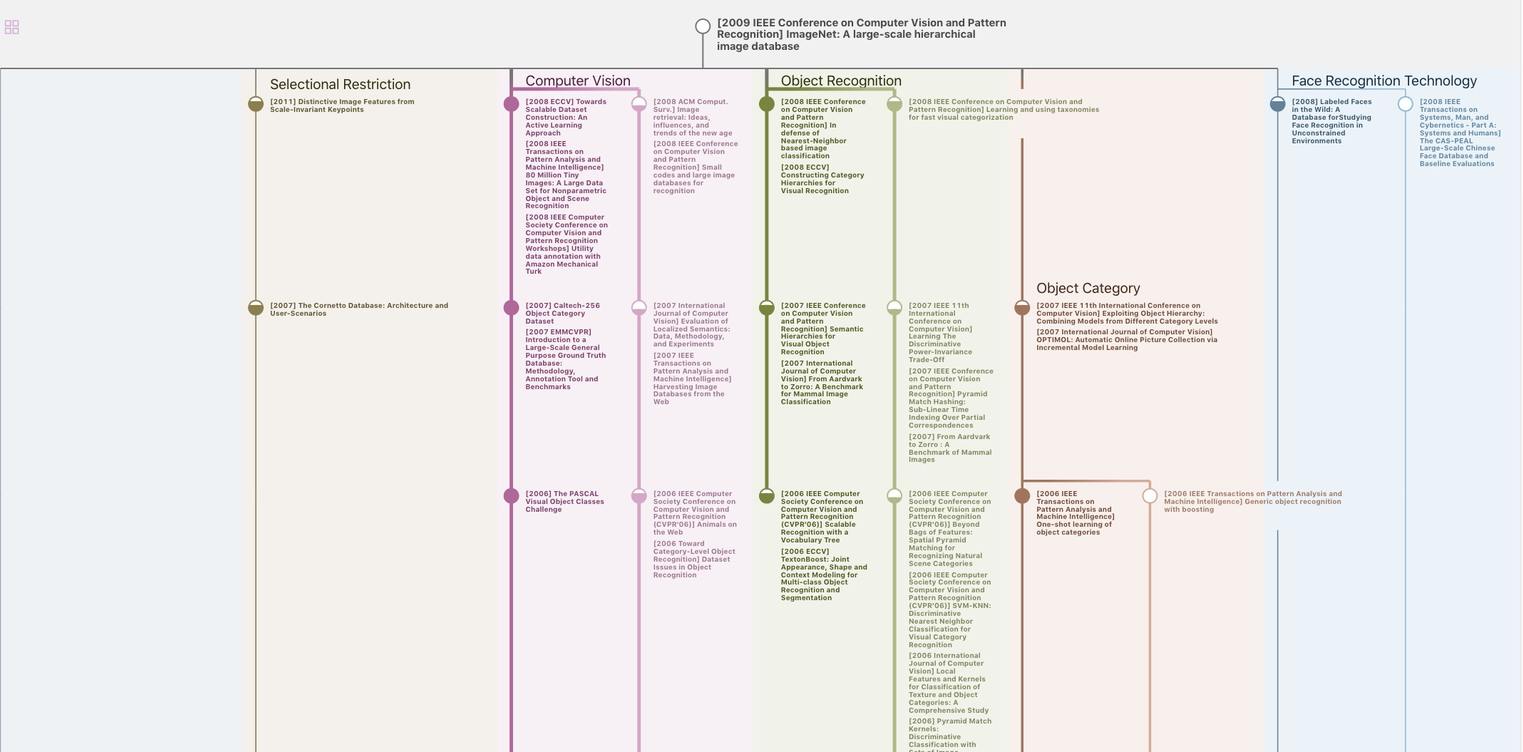
生成溯源树,研究论文发展脉络
Chat Paper
正在生成论文摘要