Predictive Modeling of Urinary Stone Composition Using Machine Learning and Clinical Data: Implications for Treatment Strategies and Pathophysiological Insights.
Journal of endourology(2024)
Abstract
Purpose: Preventative strategies and surgical treatments for urolithiasis depend on stone composition. However, stone composition is often unknown until the stone is passed or surgically managed. Given that stone composition likely reflects the physiological parameters during its formation, we used clinical data from stone formers to predict stone composition. Materials and Methods: Data on stone composition, 24-hour urine, serum biochemistry, patient demographics, and medical history were prospectively collected from 777 kidney stone patients. Data were used to train gradient boosted machine and logistic regression models to distinguish calcium vs noncalcium, calcium oxalate monohydrate vs dihydrate, and calcium oxalate vs calcium phosphate vs uric acid stone types. Model performance was evaluated using the kappa score, and the influence of each predictor variable was assessed. Results: The calcium vs noncalcium model differentiated stone types with a kappa of 0.5231. The most influential predictors were 24-hour urine calcium, blood urate, and phosphate. The calcium oxalate monohydrate vs dihydrate model is the first of its kind and could discriminate stone types with a kappa of 0.2042. The key predictors were 24-hour urine urea, calcium, and oxalate. The multiclass model had a kappa of 0.3023 and the top predictors were age and 24-hour urine calcium and creatinine. Conclusions: Clinical data can be leveraged with machine learning algorithms to predict stone composition, which may help urologists determine stone type and guide their management plan before stone treatment. Investigating the most influential predictors of each classifier may improve the understanding of key clinical features of urolithiasis and shed light on pathophysiology.
MoreTranslated text
AI Read Science
Must-Reading Tree
Example
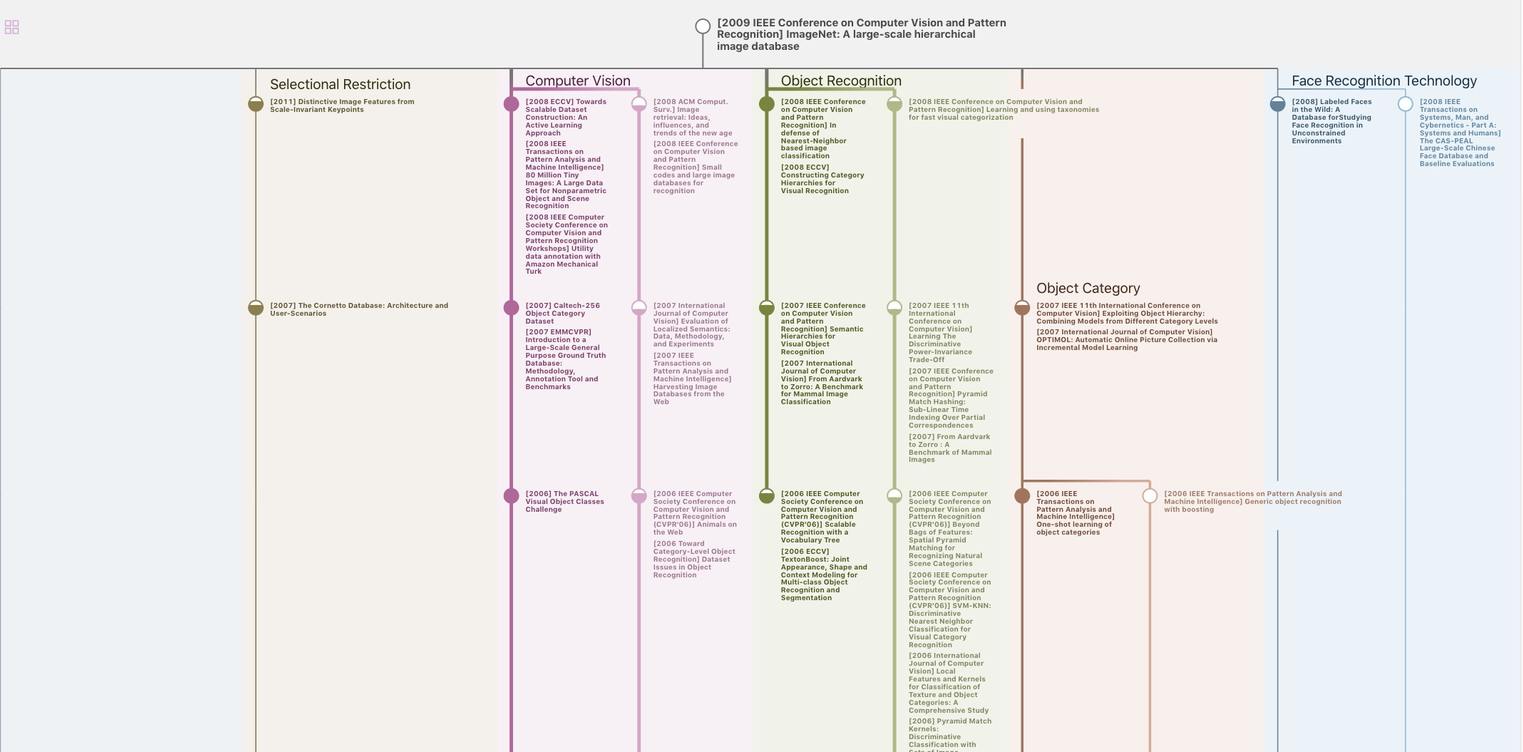
Generate MRT to find the research sequence of this paper
Chat Paper
Summary is being generated by the instructions you defined