Horizontal Federated Density Peaks Clustering
IEEE TRANSACTIONS ON NEURAL NETWORKS AND LEARNING SYSTEMS(2023)
摘要
Density peaks clustering (DPC) is a popular clustering algorithm, which has been studied and favored by many scholars because of its simplicity, fewer parameters, and no iteration. However, in previous improvements of DPC, the issue of privacy data leakage was not considered, and the "Domino" effect caused by the misallocation of noncenters has not been effectively addressed. In view of the above shortcomings, a horizontal federated DPC (HFDPC) is proposed. First, HFDPC introduces the idea of horizontal federated learning and proposes a protection mechanism for client parameter transmission. Second, DPC is improved by using similar density chain (SDC) to alleviate the "Domino" effect caused by multiple local peaks in the flow pattern dataset. Finally, a novel data dimension reduction and image encryption are used to improve the effectiveness of data partitioning. The experimental results show that compared with DPC and some of its improvements, HFDPC has a certain degree of improvement in accuracy and speed.
更多查看译文
关键词
Federated learning,Clustering algorithms,Distributed databases,Partitioning algorithms,Dimensionality reduction,Data models,Servers,Arnold transformation,density peaks clustering (DPC),dimension reduction,horizontal federated learning,similar density chain (SDC)
AI 理解论文
溯源树
样例
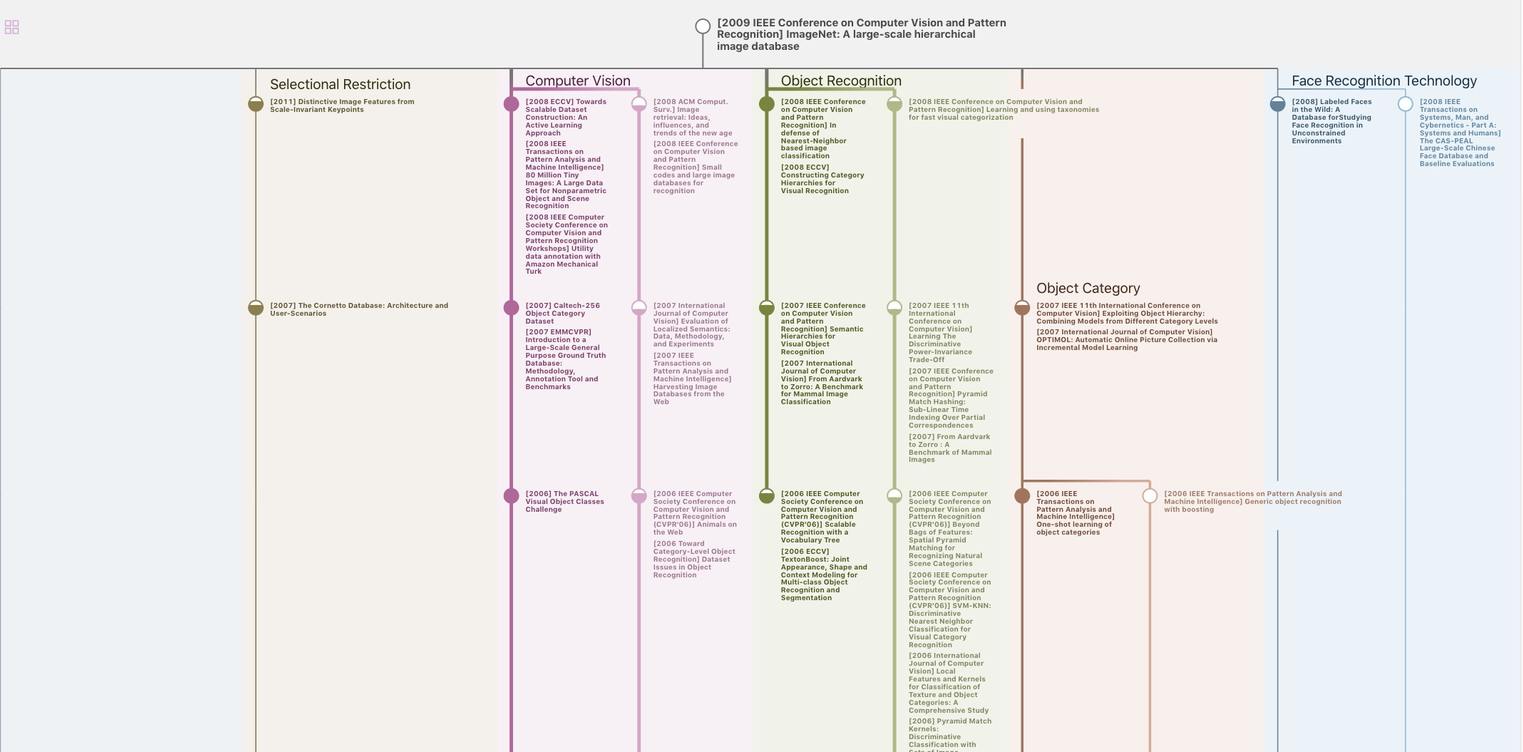
生成溯源树,研究论文发展脉络
Chat Paper
正在生成论文摘要