A Causality-Aligned Structure Rationalization Scheme Against Adversarial Biased Perturbations for Graph Neural Networks
IEEE TRANSACTIONS ON INFORMATION FORENSICS AND SECURITY(2024)
摘要
The graph neural networks (GNNs) are susceptible to adversarial perturbations and distribution biases, which pose potential security concerns for real-world applications. Current endeavors mainly focus on graph matching, while the subtle relationships between the nodes and structures of graph-structured data remain under-explored. Accordingly, two fundamental challenges arise as follows: 1) the intricate connections among nodes may induce the distribution shift of graph samples even under the same scenario, and 2) the perturbations of inherent graph-structured representations can introduce spurious shortcuts, which lead to GNN models relying on biased data to make unstable predictions. To address these problems, we propose a novel causality-aligned structure rationalization (CASR) scheme to construct invariant rationales by probing the coherent and causal patterns, which facilitates GNN models to make stable and reliable predictions in case of adversarial biased perturbations. Specifically, the initial graph samples across domains are leveraged to boost the diversity of datasets and perceive the interaction between shortcuts. Subsequently, the causal invariant rationales can be obtained during the interventions. This allows the GNN model to extrapolate risk variations from a single observed environment to multiple unknown environments. Moreover, the query feedback mechanism can progressively promote the consistency-driven optimal rationalization by reinforcing real essences and eliminating spurious shortcuts. Extensive experiments demonstrate the effectiveness of our scheme against adversarial biased perturbations from data manipulation attacks and out-of-distribution (OOD) shifts on various graph-structured datasets. Notably, we reveal that the capture of distinctive rationales can greatly reduce the dependence on shortcut cues and improve the robustness of OOD generalization.
更多查看译文
关键词
Perturbation methods,Predictive models,Reliability,Robustness,Graph neural networks,Data models,Correlation,Adversarial biased perturbations,spurious correlations,invariant causal rationales,OOD generalization,graph neural networks
AI 理解论文
溯源树
样例
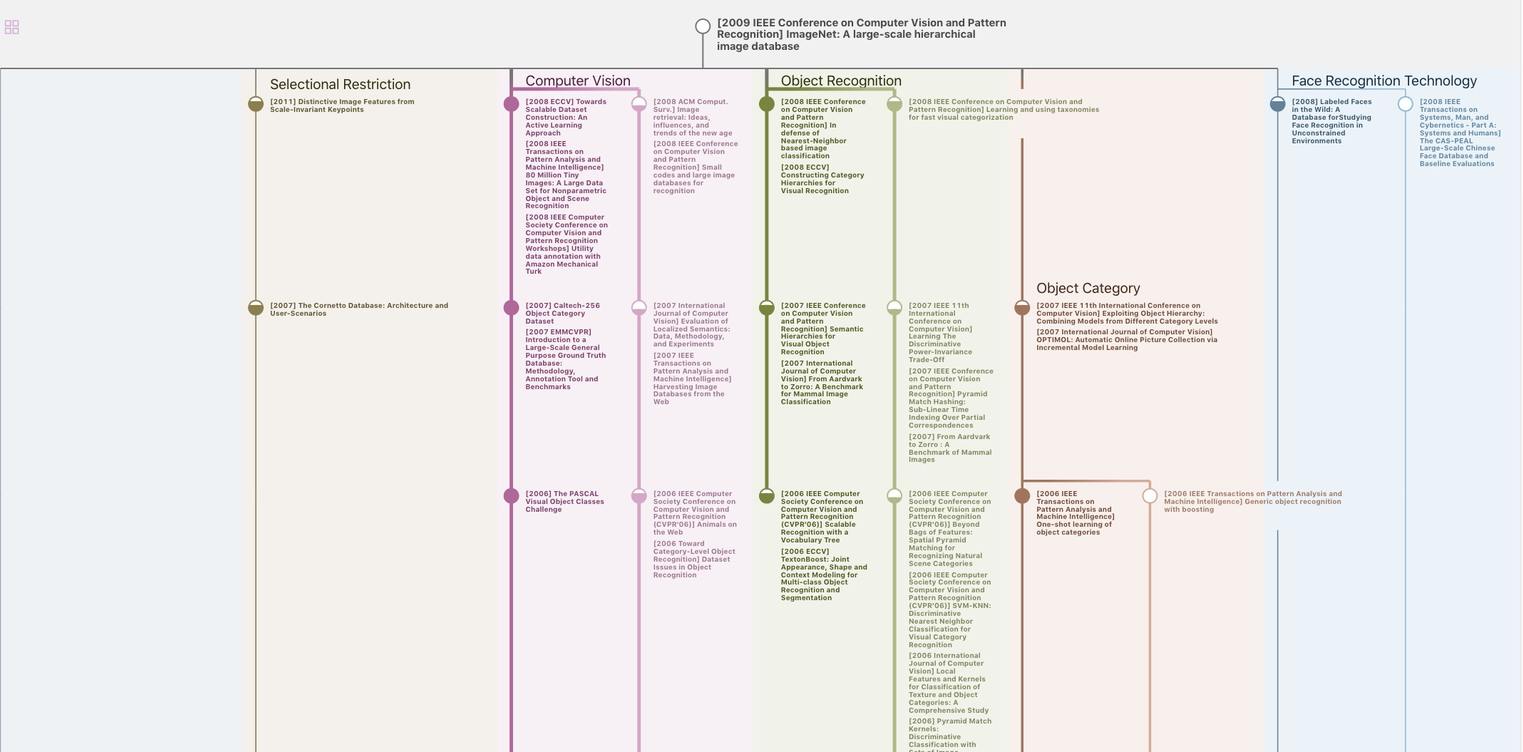
生成溯源树,研究论文发展脉络
Chat Paper
正在生成论文摘要