NeuroVelo: interpretable learning of cellular dynamics from single-cell transcriptomic data
bioRxiv (Cold Spring Harbor Laboratory)(2024)
摘要
Reconstructing temporal cellular dynamics from static single-cell transcriptomics remains a major challenge. Methods based on RNA velocity, often in combination with non-linear dimensionality reduction, have been proposed. However, interpreting their results in the light of the underlying biology remains difficult, and their predictive power is limited. Here we propose NeuroVelo, a method that couples learning of an optimal linear projection with a non-linear low-dimensional dynamical system. Using dynamical systems theory, NeuroVelo can then identify genes and biological processes driving temporal cellular dynamics. We benchmark NeuroVelo against several current methods using single-cell multi-omic data, demonstrating that NeuroVelo is superior to competing methods in terms of identifying biological pathways and reconstructing evolutionary dynamics. NeuroVelo is available at: https://github.com/idriskb/NeuroVelo
### Competing Interest Statement
Between 2018 and 2022 NV received honoraria for lectures from Merck Serono, Pfizer, Bayer, Eli-Lilly and Servier; Research funding (Institutional) from Roche and BenevolentAI and has received paid consultancy fees from BenevolentAI. At the time of submission NV was a full-time employee of AstraZeneca Plc; any work done in relation to this article was undertaken before his current employment.
更多查看译文
AI 理解论文
溯源树
样例
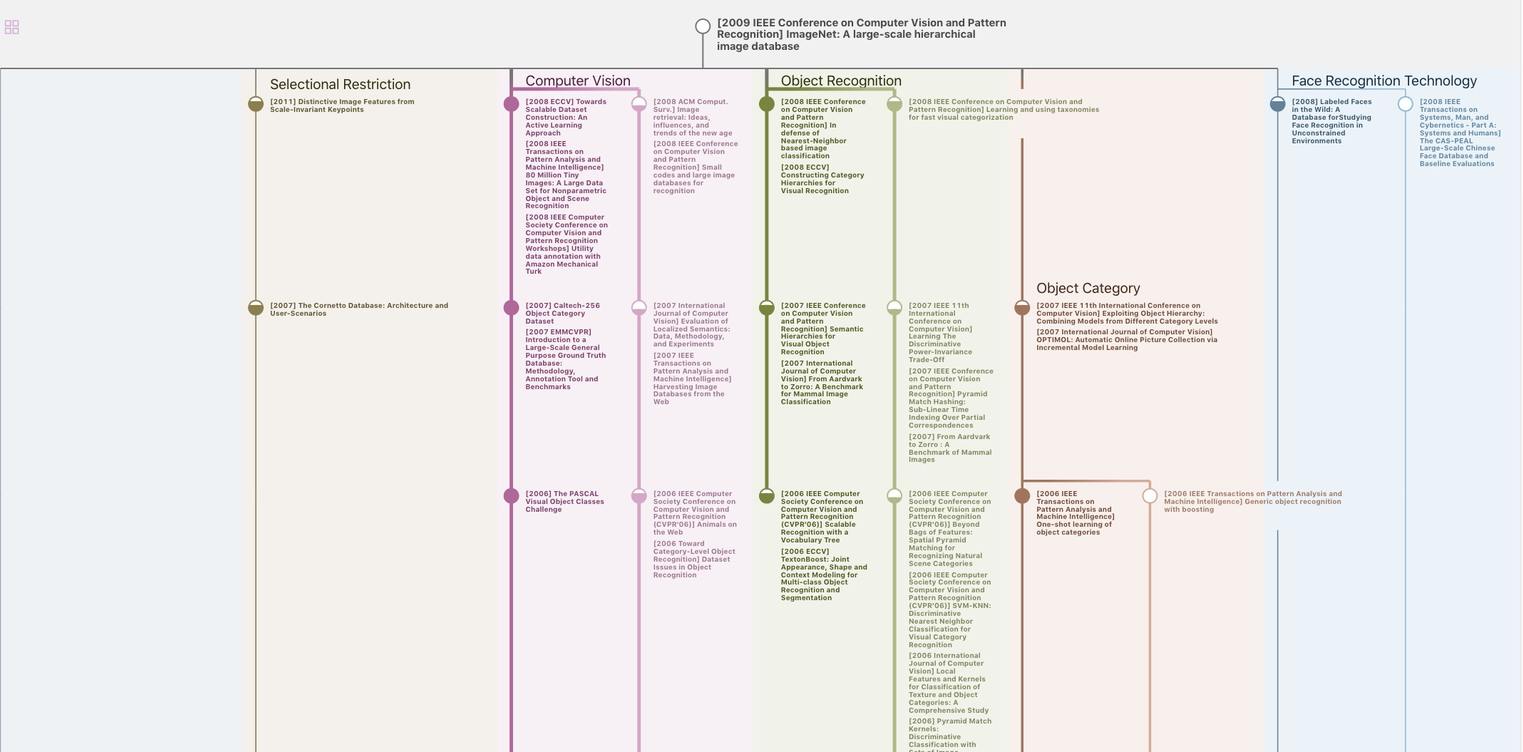
生成溯源树,研究论文发展脉络
Chat Paper
正在生成论文摘要