DRCNet: Road Extraction From Remote Sensing Images Using DenseNet With Recurrent Criss-Cross Attention and Convolutional Block Attention Module
IEEE ACCESS(2023)
摘要
Extracting road networks from remote sensing images holds critical implications for various applications including autonomous driving, path planning, and road navigation. Despite its importance, the task remains arduous due to the complex backdrops in remote sensing imagery, intricate road geometries, and the challenges arising from vegetation and structural obstructions. To address these multifaceted issues, we introduce a specialized model for road extraction in remote sensing images, termed DRCNet. This model employs a pre-trained DenseNet-121 as its encoder and is fortified with both Recurrent Criss-Cross Attention (RCCA) and Convolutional Block Attention Module (CBAM). RCCA facilitates the capture of global contextual information across all pixels, thereby enriching the model's understanding of global image relationships. Simultaneously, CBAM is integrated within the skip connections to optimize the network's focus on significant road features. Comprehensive experiments conducted on both the DeepGlobe Road and Massachusetts Road datasets substantiate that DRCNet outperforms other benchmark models in road detection tasks.
更多查看译文
关键词
Roads,Feature extraction,Remote sensing,Data mining,Decoding,Convolutional codes,Semantics,Path planning,Road extraction,remote sensing image,DenseNet-121 network,attention module
AI 理解论文
溯源树
样例
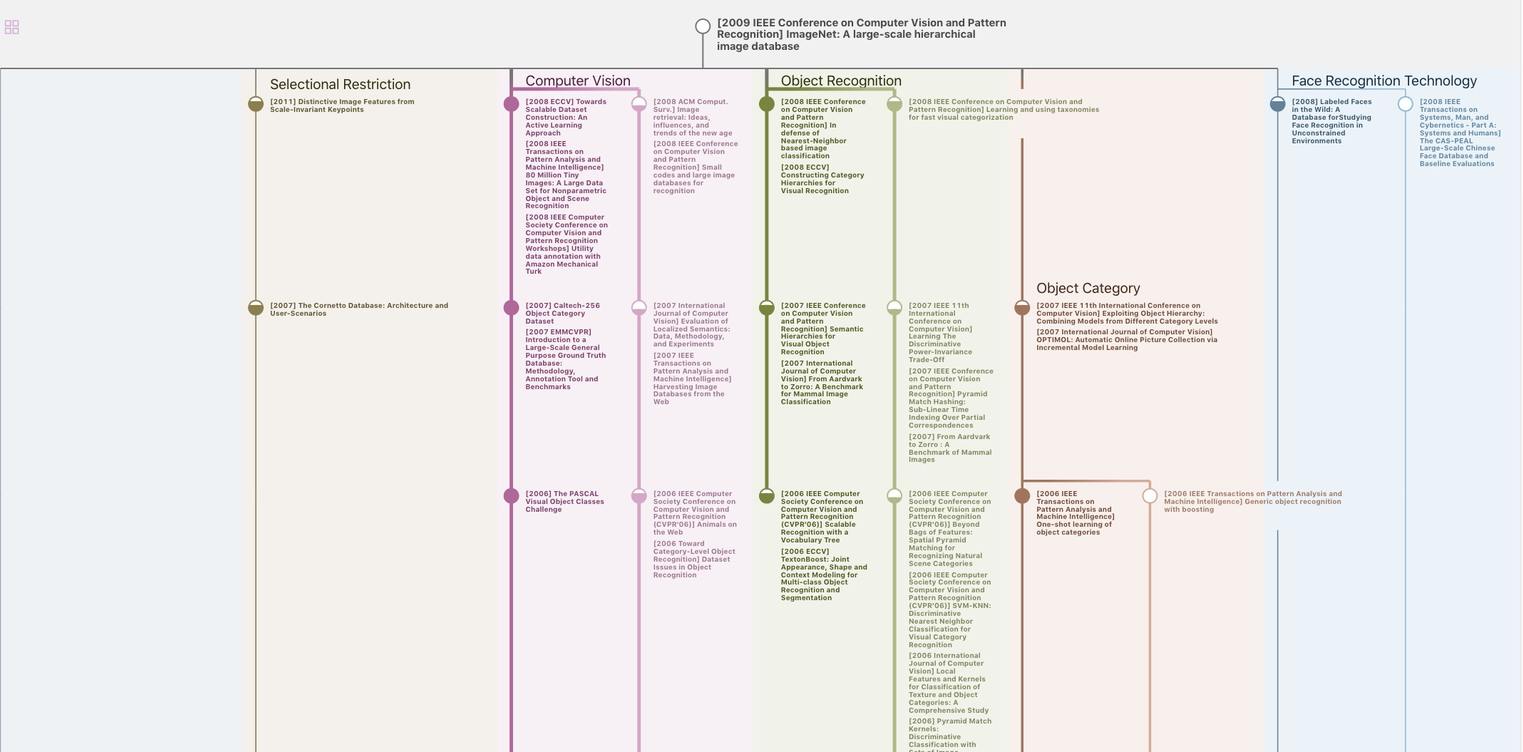
生成溯源树,研究论文发展脉络
Chat Paper
正在生成论文摘要