Towards Robust Temporal Reasoning of Large Language Models via a Multi-Hop QA Dataset and Pseudo-Instruction Tuning
arxiv(2023)
Abstract
Knowledge in the real world is being updated constantly. However, it is
costly to frequently update large language models (LLMs). Therefore, it is
crucial for LLMs to understand the concept of temporal knowledge. However,
prior works on temporal question answering (TQA) did not emphasize multi-answer
and multi-hop types of temporal reasoning. In this paper, we propose a complex
temporal question-answering dataset Complex-TR that focuses on multi-answer and
multi-hop temporal reasoning. Besides, we also propose a novel data
augmentation strategy to improve the complex temporal reasoning capability and
robustness of LLMs. We conducted experiments on multiple temporal QA datasets.
Experimental results show that our method is able to improve LLMs' performance
on temporal QA benchmarks by significant margins. Our code and data are
released at: https://github.com/nusnlp/complex-tr.
MoreTranslated text
AI Read Science
Must-Reading Tree
Example
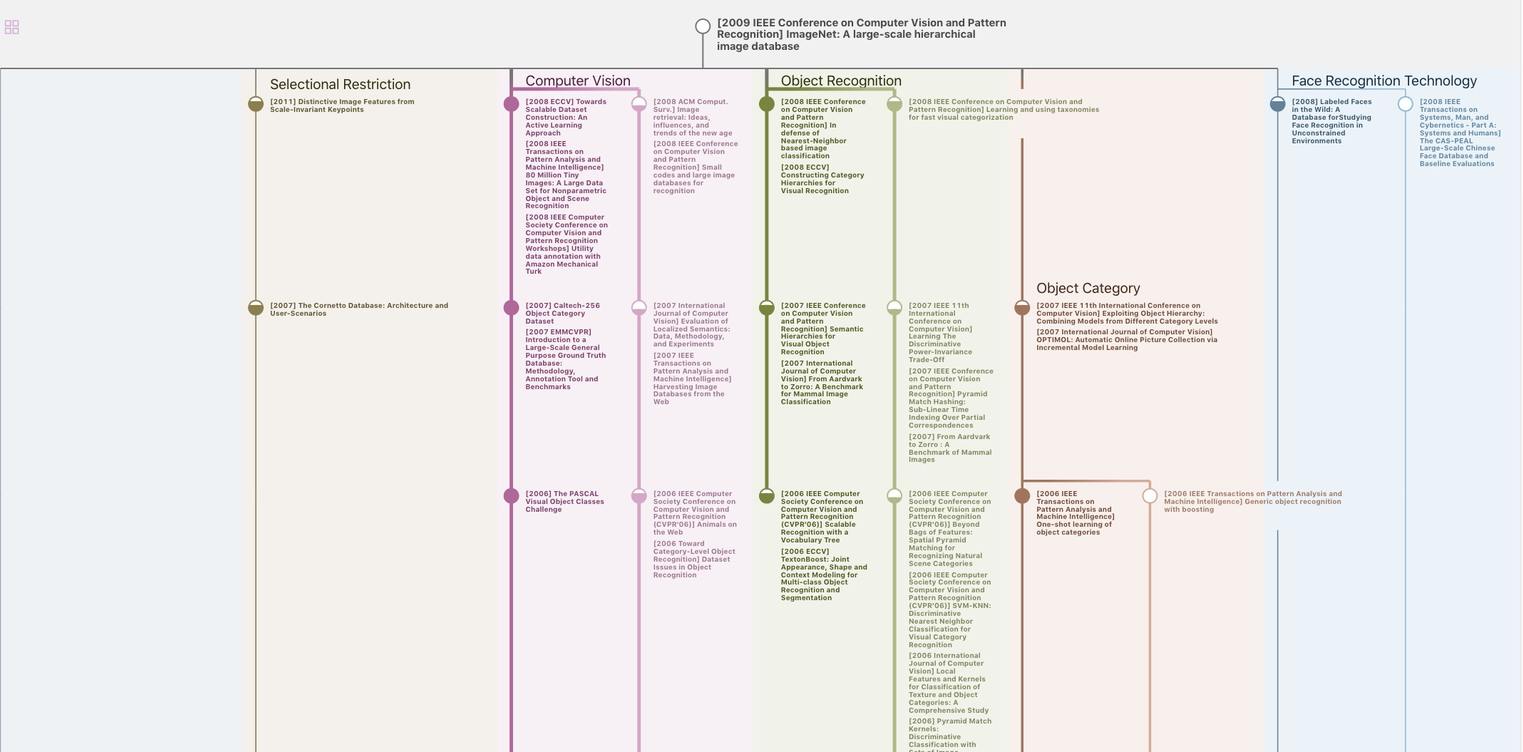
Generate MRT to find the research sequence of this paper
Chat Paper
Summary is being generated by the instructions you defined