Evaluating LLM Agent Group Dynamics against Human Group Dynamics: A Case Study on Wisdom of Partisan Crowds.
CoRR(2023)
Abstract
This study investigates the potential of Large Language Models (LLMs) to
simulate human group dynamics, particularly within politically charged
contexts. We replicate the Wisdom of Partisan Crowds phenomenon using LLMs to
role-play as Democrat and Republican personas, engaging in a structured
interaction akin to human group study. Our approach evaluates how agents'
responses evolve through social influence. Our key findings indicate that LLM
agents role-playing detailed personas and without Chain-of-Thought (CoT)
reasoning closely align with human behaviors, while having CoT reasoning hurts
the alignment. However, incorporating explicit biases into agent prompts does
not necessarily enhance the wisdom of partisan crowds. Moreover, fine-tuning
LLMs with human data shows promise in achieving human-like behavior but poses a
risk of overfitting certain behaviors. These findings show the potential and
limitations of using LLM agents in modeling human group phenomena.
MoreTranslated text
AI Read Science
Must-Reading Tree
Example
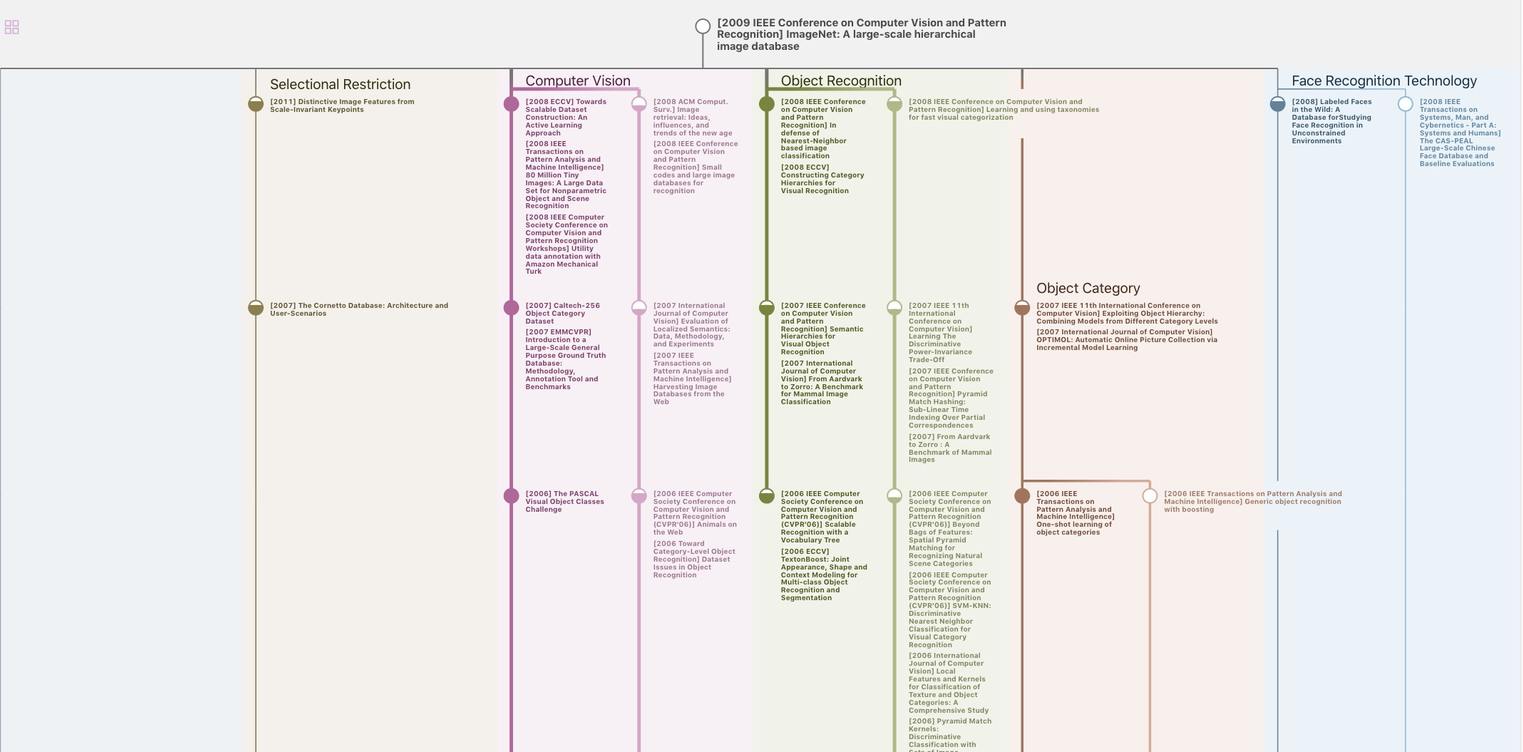
Generate MRT to find the research sequence of this paper
Chat Paper
Summary is being generated by the instructions you defined