Soft Matching Distance: A metric on neural representations that captures\n single-neuron tuning
UniReps(2023)
Abstract
Common measures of neural representational (dis)similarity are designed to be\ninsensitive to rotations and reflections of the neural activation space.\nMotivated by the premise that the tuning of individual units may be important,\nthere has been recent interest in developing stricter notions of\nrepresentational (dis)similarity that require neurons to be individually\nmatched across networks. When two networks have the same size (i.e. same number\nof neurons), a distance metric can be formulated by optimizing over neuron\nindex permutations to maximize tuning curve alignment. However, it is not clear\nhow to generalize this metric to measure distances between networks with\ndifferent sizes. Here, we leverage a connection to optimal transport theory to\nderive a natural generalization based on soft permutations. The resulting\nmetric is symmetric, satisfies the triangle inequality, and can be interpreted\nas a Wasserstein distance between two empirical distributions. Further, our\nproposed metric avoids counter-intuitive outcomes suffered by alternative\napproaches, and captures complementary geometric insights into neural\nrepresentations that are entirely missed by rotation-invariant metrics.
MoreTranslated text
AI Read Science
Must-Reading Tree
Example
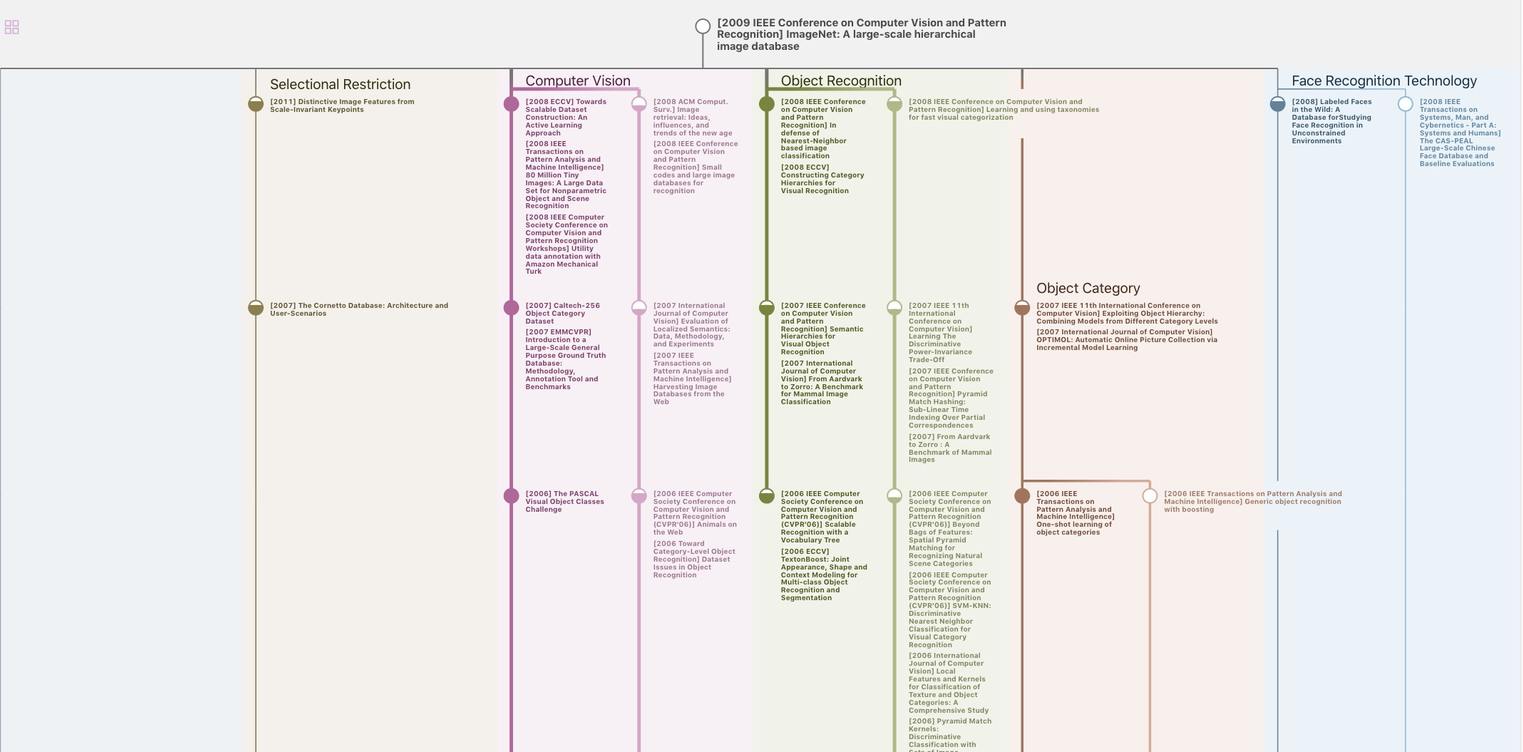
Generate MRT to find the research sequence of this paper
Chat Paper
Summary is being generated by the instructions you defined