Heterogeneous Ensemble Approaches for Robust Face Mask Detection in Crowd Scenes
Journal of Computational and Cognitive Engineering(2023)
摘要
Face masks are one of the effective tools to slow the spread of disease and reduce medical overload by protecting people from infectious diseases including COVID-19. To prevent infection from respiratory droplets, it is imperative to wear a mask that covers the nose and mouth completely. However, it is difficult to make it mandatory for crowds to wear masks in public places where many people gather. For example, detecting incorrect mask-wearing in crowded scenes is a tedious and attention-grabbing task. Therefore, the success of deep learning in computer vision motivates automated monitoring systems. However, deep learning-based detection models are unstable if the domain task is changed and may have different strengths and weaknesses. Therefore, in this study, we propose a heterogeneous ensemble-based detection model for robust face mask detection in crowd scenes. First, independent detection models such as You look Only Ones (YOLO) v6, YOLO v7, and Faster R-CNN are employed for the model ensemble. Second, the prediction results obtained from the detection models are post-processed such as merging, non-maximum suppression, and weighted box fusion. The experimental results show that the classification performance of our proposed model has an F1 score of about 90.5% and that the improvement of the generalization ability due to the ensemble strategy contributed to the improvement of the classification performance.
更多查看译文
关键词
face mask detection,crowd scenes,deep learning,heterogeneous ensemble
AI 理解论文
溯源树
样例
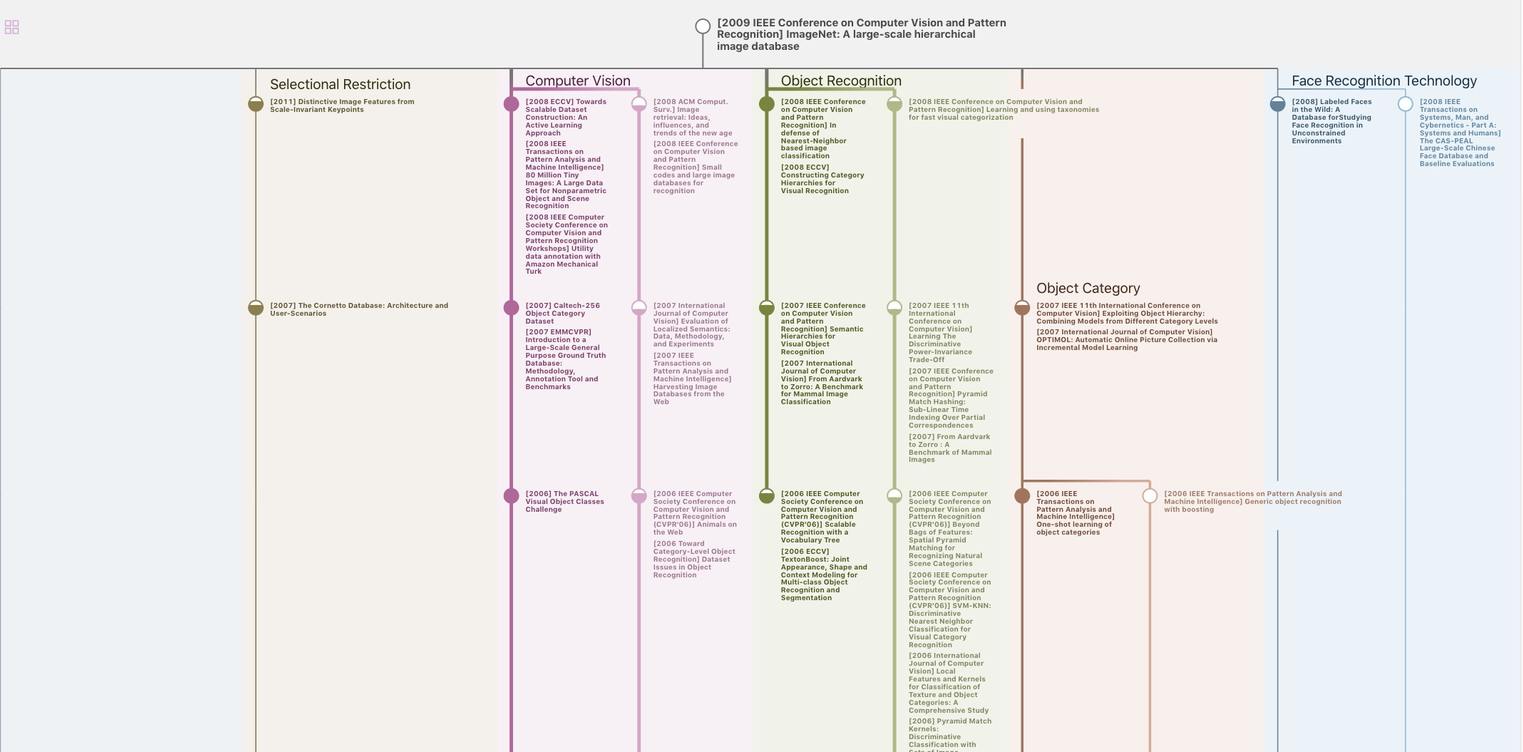
生成溯源树,研究论文发展脉络
Chat Paper
正在生成论文摘要