Self-Supervised Disentanglement by Leveraging Structure in Data Augmentations.
CoRR(2023)
Abstract
Self-supervised representation learning often uses data augmentations to induce some invariance to "style" attributes of the data. However, with downstream tasks generally unknown at training time, it is difficult to deduce a priori which attributes of the data are indeed "style" and can be safely discarded. To address this, we introduce a more principled approach that seeks to disentangle style features rather than discard them. The key idea is to add multiple style embedding spaces where: (i) each is invariant to all-but-one augmentation; and (ii) joint entropy is maximized. We formalize our structured data-augmentation procedure from a causal latent-variable-model perspective, and prove identifiability of both content and (multiple blocks of) style variables. We empirically demonstrate the benefits of our approach on synthetic datasets and then present promising but limited results on ImageNet.
MoreTranslated text
AI Read Science
Must-Reading Tree
Example
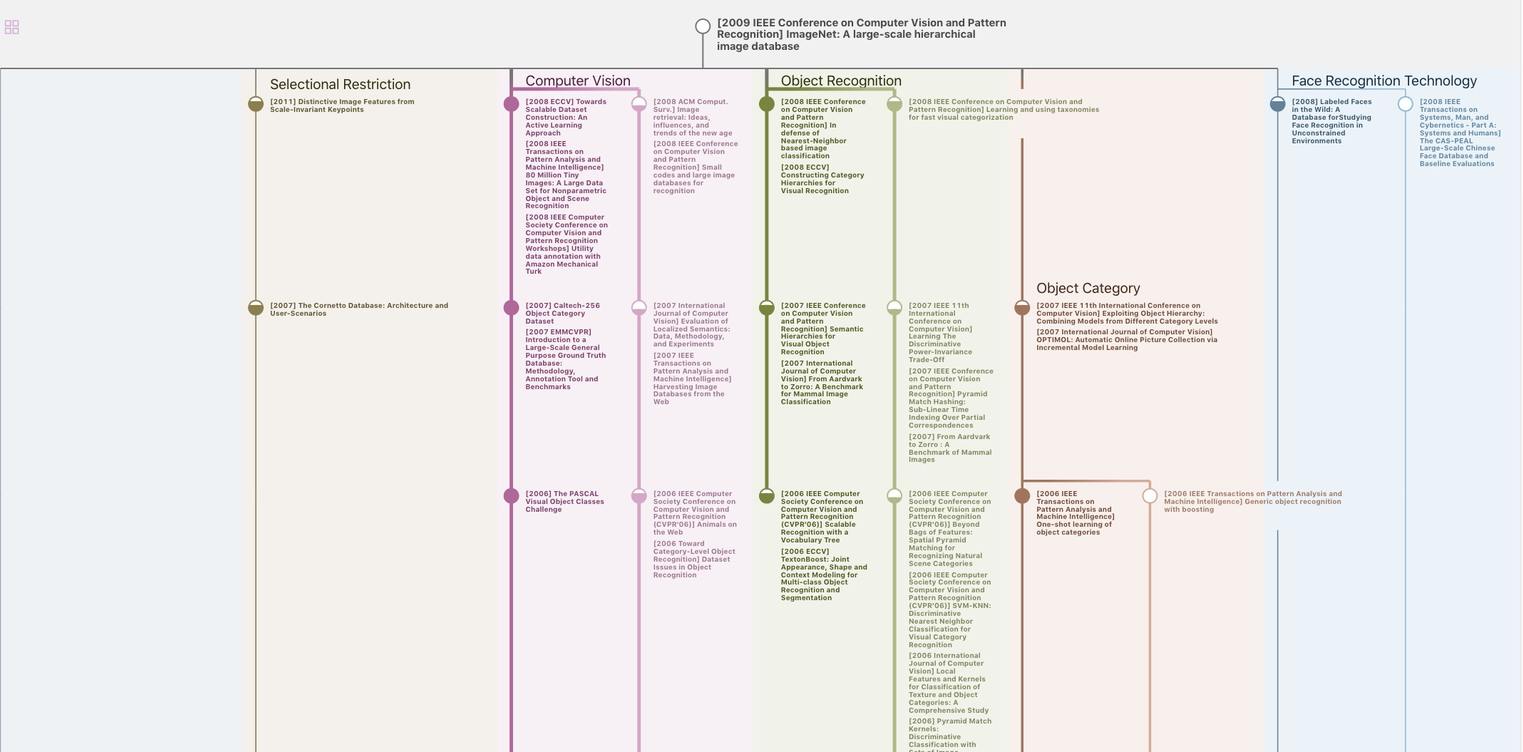
Generate MRT to find the research sequence of this paper
Chat Paper
Summary is being generated by the instructions you defined