CGCom: a framework for inferring Cell-cell Communication based on Graph Neural Network
bioRxiv the preprint server for biology(2023)
摘要
Cell-cell communication is crucial in maintaining cellular homeostasis, cell survival and various regulatory relationships among interacting cells. Thanks to recent advances of spatial transcriptomics technologies, we can now explore if and how cells’ proximal information available from spatial transcriptomics datasets can be used to infer cell-cell communication. Here we present a cell-cell communication inference framework, called CGCom, which uses a graph neural network (GNN) to learn communication patterns among interacting cells by combining single-cell spatial transcriptomic datasets with publicly available ligand-receptor information and the molecular regulatory information down-stream of the ligand-receptor signaling. To evaluate the performance of CGCom, we applied it to mouse embryo seqFISH datasets. Our results demonstrate that CGCom can not only accurately infer cell communication between individual cell pairs but also generalize its learning to predict communication between different cell types. We compared the performance of CGCom with two existing methods, CellChat and CellPhoneDB, and our comparative study revealed both common and unique communication patterns from the three approaches.
Commonly found communication patterns include three sets of ligand-receptor communication relationships, one between surface ectoderm cells and spinal cord cells, one between gut tube cells and endothelium, and one between neural crest and endothelium, all of which have already been reported in the literature thus offering credibility of all three methods. However, we hypothesize that CGCom is superior in reducing false positives thanks to its use of cell proximal information and its learning between specific cell pairs rather than between cell types. CGCom is a GNN-based solution that can take advantage of spatially resolved single-cell transcriptomic data in predicting cell-cell communication with a higher accuracy.
### Competing Interest Statement
The authors have declared no competing interest.
更多查看译文
AI 理解论文
溯源树
样例
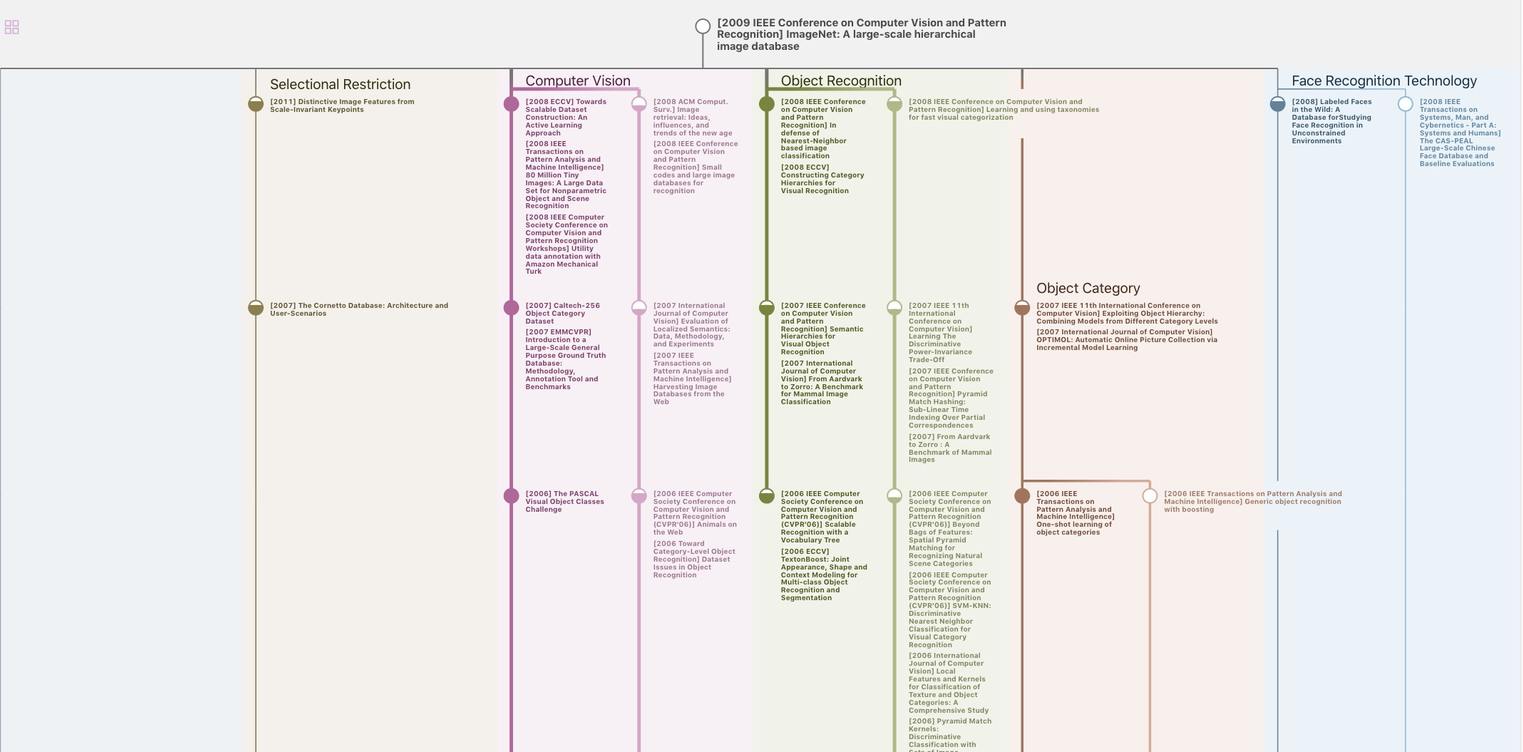
生成溯源树,研究论文发展脉络
Chat Paper
正在生成论文摘要